Building the Data Foundation for AI
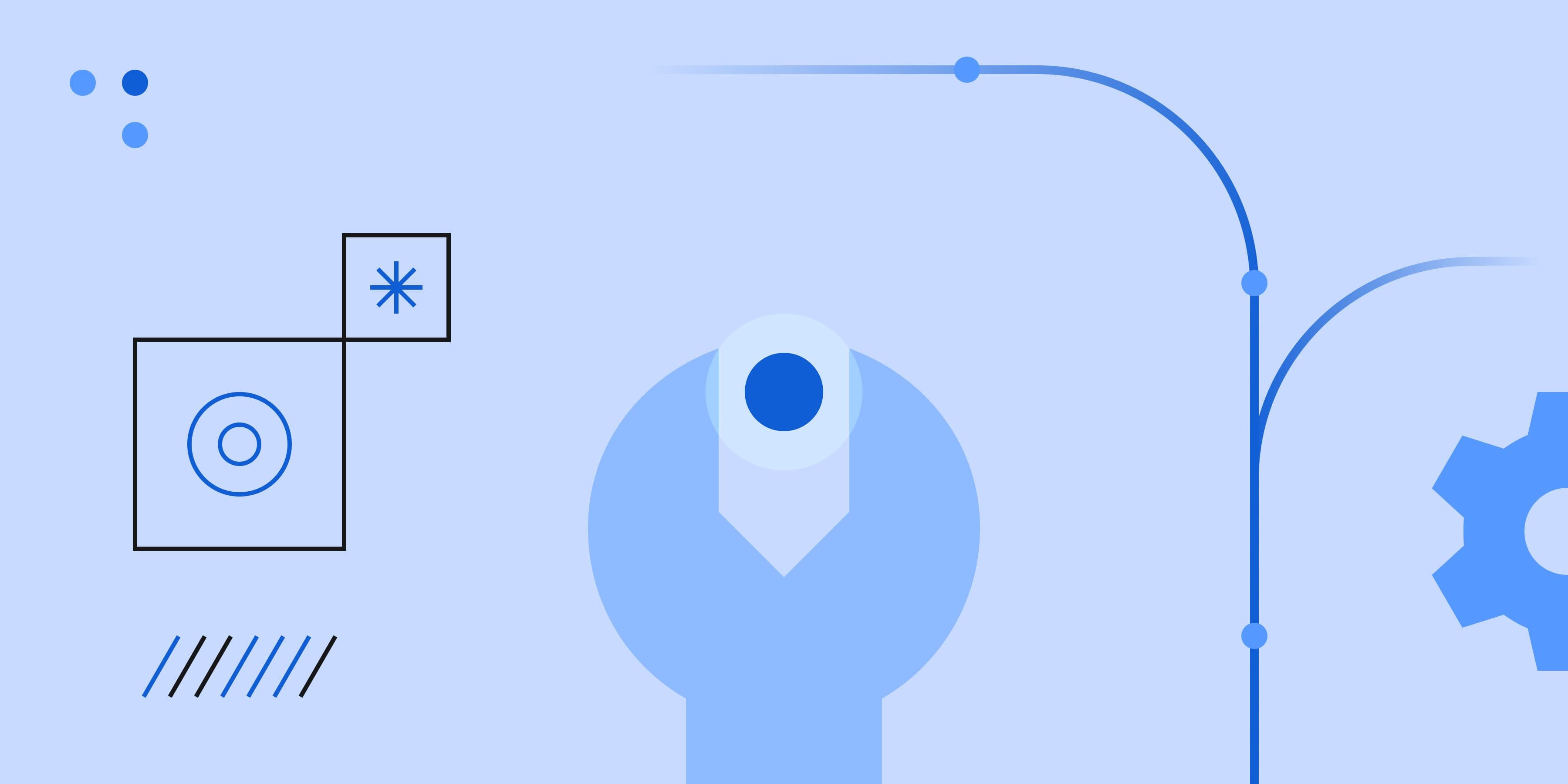
Long before Generative AI entered the public consciousness, sophisticated marketers created personalized experiences using Machine Learning models built by their data teams to improve the effectiveness of their marketing programs.
Now, with the democratization of AI - thanks to ChatGPT - thousands of AI tools have cropped up that create personalized experiences out-of-the-box. From website personalization to customer service chat bots, these AI tools run the full gamut of solutions.
With AI expected to drive a 10-20% increase in customer engagement, it’s no wonder budgets for AI are anticipated to surge from $124 billion in 2022 to $297 billion in 2027 (Gartner).
Despite the increase in popularity of AI tools, the hard reality from the advent of computing has not changed - you need quality data to deliver quality insights, or in other words, garbage-in, garbage out. In the context of customer data, most of these AI tools have focused on the “last-mile” of customer activation. They do not address the underlying data foundation that these powerful AI models need to truly deliver value.
As an example, the effectiveness of a customer service chatbot can be significantly enhanced to provide hyper-personalized experiences if it has access to the full customer profile, including lifetime value, date of last purchase, and likelihood to churn. In this blog, we call these last-mile AI tools “point solutions”.
Each point solution creates their own personalization model using a different dataset. The result is bespoke AI implementations that create local maxima across several siloed tools, each addressing a different part of the customer journey. Not only does this duplicate work for data and business teams alike, but the lack of universal context and continuity also leads to a disjointed, suboptimal experience for customers (see here).
So while leaders think they are getting ahead of the curve by investing in AI point solutions, they’re actually undermining their long-term AI strategy – and their bottom line.
Here’s the deal: Weaving together multiple AI tools is not an AI strategy and won’t get your organization where you want to go.
So how do companies realize value from their AI investments? The answer lies in the warehouse or data lake.
That’s because a robust AI strategy starts with a solid data foundation. First, you need to collect clean, quality data; second, you need to make sure that data is complete and accessible to all of your customer engagement tools (including your customer service chatbot!).
Beyond Point Solutions - The Need for a Solid Data Foundation
Building a solid foundation for AI means going back to basics: data quality, completeness and accessibility.
The truth is, AI models are only as good as the data they're built on. When you have data scattered across tools, it’s nearly impossible to avoid data quality issues. Even the best data scientists can’t out-engineer bad data.
Moreover, poor data quality majorly strains the data team's time. Instead of spending their hours building advanced models, they’re wrangling data that’s stuck in siloed systems across your tech stack, trying to make sense of it while they build in parallel.
What’s left are half-baked models built on bad data and a burned out data team - a far cry from the business results promised by AI.
It’s not just data quality that’s imperative - data completeness is of equal importance. Incomplete datasets mean incorrect models, which, at best, won’t drive the results you’re looking for, and at worst, could be detrimental to your reputation or business.
Finally, your data must be accessible. Data that’s stuck in siloed tools and can’t be activated in other platforms is of no use at all.
Building a Solid Data Foundation Starts in the Warehouse
At this juncture, it’s worth mentioning that the proliferation of AI tooling is primarily driven by business teams looking to test and eventually leverage these tools to deliver value. That is admirable and should not necessarily be discouraged.
The more crucial point is that these point solutions deployed by business teams are not sufficient and over time, should be subsumed as part of a broader AI-readiness effort led by the data team. That is because building the data foundation is, at its core, an engineering problem.
Once you have identified that AI is an important component of your strategy, it’s time to ensure your data team is at the table and is appropriately resourced. When the data team is involved, the most important building blocks for a successful implementation are within view - with the cloud data warehouse or data lake at the center.
Building AI applications on top of your warehouse or data lake eliminates issues posed by those pesky silos created by point solutions. Collecting data from all sources in your warehouse creates a single source of truth, ensuring that all downstream tools receive the same data.
Once you’ve invested in collecting and unifying quality data in your warehouse, your data team will no longer have to spend time wrestling with bad data platform-by-platform. Instead, clean, consistent and complete data will flow to downstream tools, freeing your team to work unencumbered on advanced GenAI and ML projects.
What’s more, your warehouse is the best place to build the most comprehensive customer 360s that power integrated personalized experiences. With quality data from every source at the ready, your data team can build a complete view of the customer with insight across the entire customer journey to power rich personalized experiences at every touch point.
With AI point solutions, you can certainly build personalized experiences, but the problem is that those personalization engines only have insight into the behaviors customers are taking within their own tool. For example, an email outreach platform has no idea what pages a customer may have visited on your website, information that is extremely valuable and crucial to building the most specific personalized campaign possible.
Personalization engines are simply more powerful when they have full context of an individual’s behavior across the entire customer journey. Without that context, you’re not exploiting personalization’s full potential - and leaving money on the table.
With a clean, complete view of the customer in the warehouse, your data team can now easily build revenue-generating AI and ML models on that data, activating those models across any number of point solutions with confidence that each is playing a critical part in a highly orchestrated and integrated personalized campaign.
DIY is hard and SaaS solutions are not up to the challenge
Building a solid data foundation in the warehouse pays dividends. It creates an environment where your data team can operate at its fullest capacity and potential and leaves room to build towards an AI-fueled future.
That said, building the foundation for AI is easier said than done.
First, you need to collect customer data from every relevant source, which is typically a complex and fragmented landscape that includes multiple web properties, mobile applications, and SaaS tools.
Next, you need to fix any data quality issues, which often includes manual custom schema fixes or solving problems via brute-force SQL or Python.
Finally, you need to unify your data into complete customer profiles, a highly complicated task that requires writing and maintaining vast amounts of SQL to identify anonymous users, build an identity graph, and compute user features on top.
The two avenues to solve these problems have traditionally been to (1) do-it-yourself (DIY), or (2) purchase a SaaS solution, but each path comes with its own set of challenges.
The DIY approach enables data teams to retain the most control and flexibility over their data, and while it may work for businesses with large engineering teams, most companies that go down this route face obstacles. Projects take longer than expected and go over budget, and building a solution that’s sufficient for small data sets may not work at scale.
On the other hand, some data teams opt to implement SaaS CDP solutions to save time. These often come in the form of Marketing CDPs and handle last-mile customer engagement; however, these tools are often black boxes and are not able to access all customer data.
RudderStack opens up a third path for data teams: to build the data foundation in the warehouse faster than DIY approaches while retaining the same level of control.
RudderStack builds the foundation for you so that your data team can do the actual hard work: building AI-powered customer experiences to turn your customer data into competitive advantage.
Preparing for an AI-Driven Future with RudderStack
RudderStack, the leading warehouse-native CDP, is uniquely positioned to help data teams get AI-ready in weeks instead of months. Built directly on top of your warehouse or data lake, it leverages all of the advantages of storing your data in a centralized location while eliminating time-consuming and expensive data wrangling – giving time back to your data team to focus on value-creating AI initiatives.
RudderStack streamlines the cumbersome task of collecting behavioral event data across the entire customer journey through our Event Stream pipelines. With our suite of SDKs, you can gather data from all sources using a standard schema and consolidate it within your cloud data warehouse.
Further, our data quality toolkit does the engineering dirty work for you. It provides a collaborative catalog you can use to ensure every team and tool is on the same page. It makes it easy to manage any violations using custom rules. It also delivers system-wide observability with a granular monitoring and alerting dashboard. For bad data that does make it through, you can write code to fix schemas in real-time, without the need to redeploy your websites or apps.
RudderStack’s Profiles product then takes your clean customer data and automatically builds an identity graph that’s used as the basis for complete customer profiles. With RudderStack, you can swiftly produce a reliable customer 360 table while retaining the ability to create and modify user attributes without the need for complex SQL. Crucially, RudderStack abstracts the time- and resource-intensive MLOps infra work. Our Predictions ML product provides a feature store and MLOps infrastructure required for building, training and running production ML models.
With RudderStack, you don’t have to waste time and money managing integrations, cleaning data, resolving identities, or training, tuning, and serving AI models. We build the foundation for you so that your data team can do the actual hard work: building AI-powered customer experiences to turn your customer data into competitive advantage.
After implementing RudderStack to build their data foundation, Wyze, a leading smart home security company, realized some of the major benefits of the AI promised land. They increased the productivity of their data engineering and AI/ML teams three-fold and ten-fold, respectively. Using models built by their engineering and AI/ML teams, Wyze’s marketing team tripled campaign velocity, increasing conversion rates.
The Road Ahead – Embracing AI with Confidence
AI is a transformational technological development that’s here to stay. Business leaders know that harnessing the power of AI will be pivotal in creating sustained, competitive differentiation in the market. If you think you’re getting a lot of pressure from your CEO now to generate value using AI, that pressure is only going to increase going forward.
The shift towards cloud data warehouses and data lakes has created the perfect environment to make delivering on complex use cases possible. You can collect more data than ever before, store it in a centralized location, and leverage it to build complex use cases.
The biggest obstacle standing between you and your AI goals: how you decide to deploy your data team. You could optimize for short-term AI gains, implementing AI point solutions that solve narrow problems. Or, you could invest in building a strong data foundation now, setting up your engineering team for success - and maximizing ROI.
RudderStack makes it easier than ever to prepare for an AI-driven future. When you make your data warehouse the foundation of your CDP, you can finally establish a single source of truth, manage data quality and start leveraging all of your customer data to drive better business outcomes - and most importantly - unleash the power of your data team.
If you’re ready to start building the foundation for AI, RudderStack can help. Schedule a demo with our team today to learn more about how RudderStack can help you turn your customer data into competitive advantage.
Build the data foundation for AI
Schedule a demo with our team today to learn how RudderStack can help you build your data foundation to turn your customer data into competitive advantage.Recent Posts
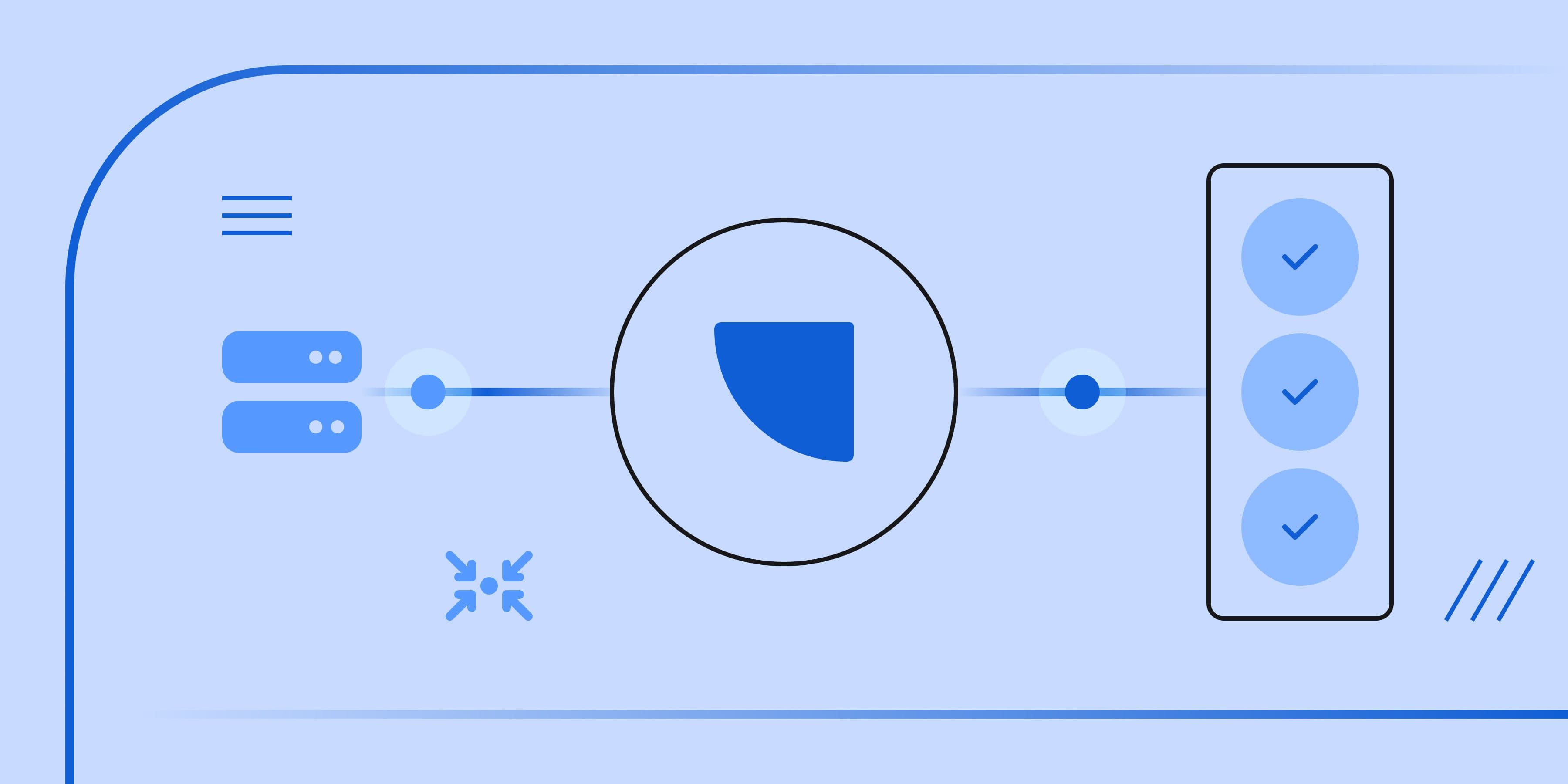
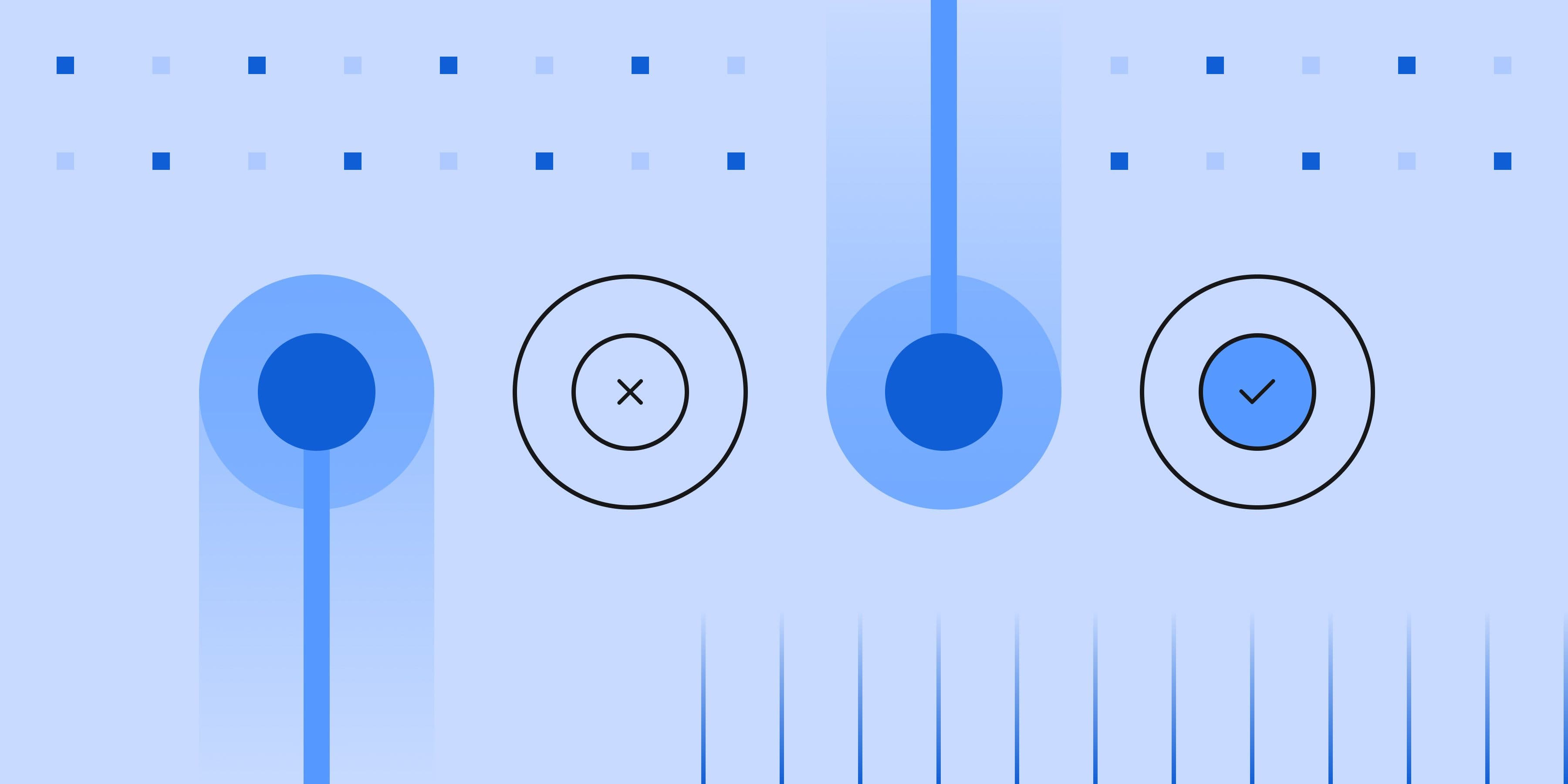
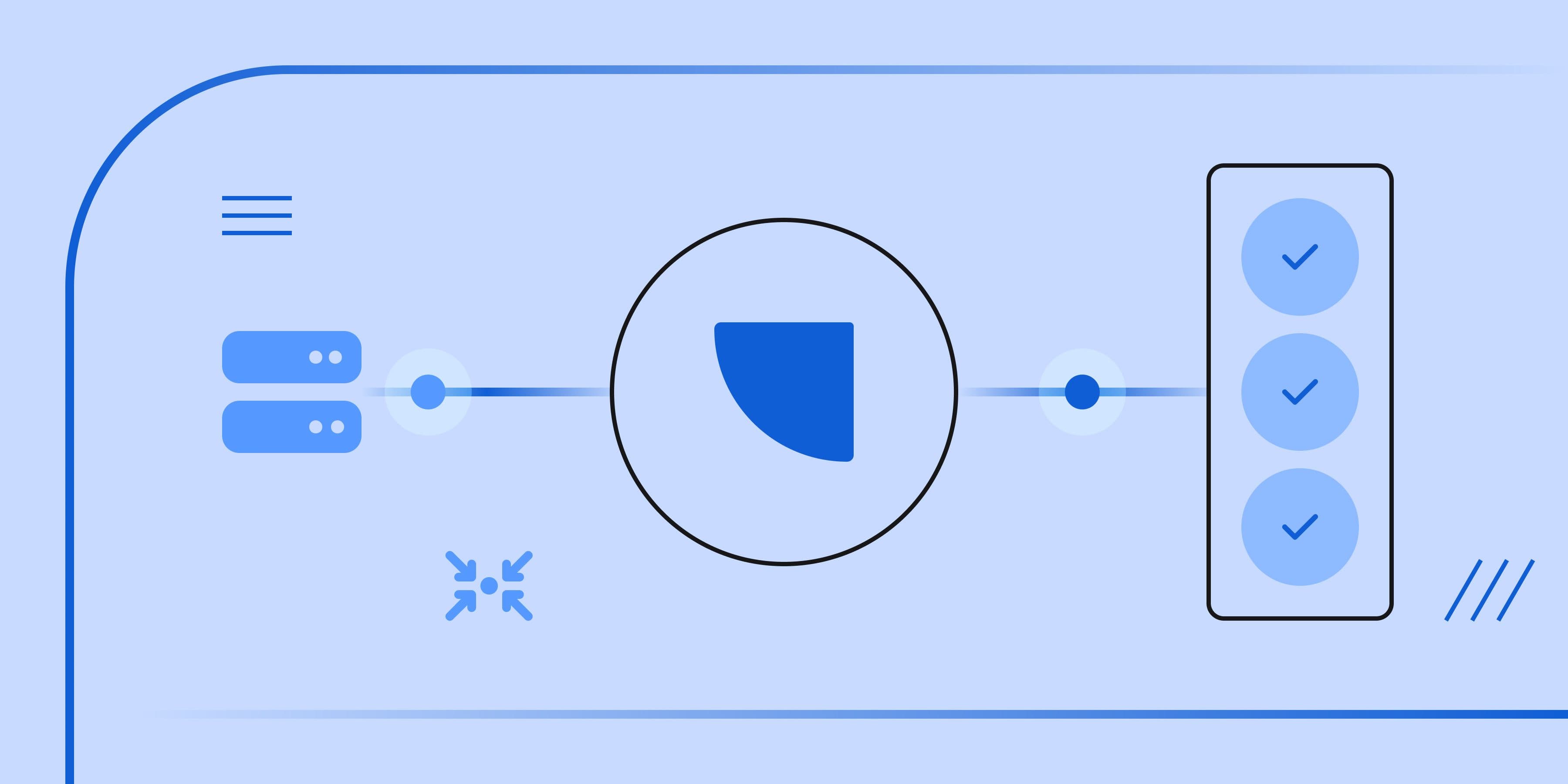