Feeling stuck with Segment? Say š to RudderStack.
Events
TechCrunch Sessions: Data Warehouse: the Foundation of the Modern Data Stack
What we will cover:
- Data trends from the last decade: why is now the time for the date warehouse?
- How have use cases for the data warehouse changed?
- Batch vs. real-time: what are the use cases and is the warehouse up to the task?
- What are examples architectures for modern stacks?
- How does the modern stack enable machine learning use cases?
- What is advice for leaders building and managing data infrastructure at their company?
Speakers
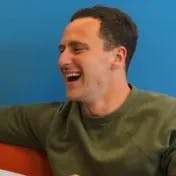
Benjamin Gotfredson
Global Startup Program Manager at Snowflake

Jamila Satani
Marketing Analytics & Insights Leader at Tonal
Creative analytics leader with 10+ years of experience delivering actionable insights through data analysis and a visual storytelling approach.
Adam Gross
Ex-CEO, Heroku
Adam Gross is an investor and board member who has build several successful companies in the data space and served in leadership roles at companies like Heroku and Salesforce.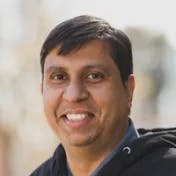
Soumyadeb Mitra
Founder and CEO of RudderStack
Transcript
Adam Gross (0:05)
Good morning, everybody. Thanks for taking the time to join our session. I hope that one of the more interesting and compelling ones that you will be experiencing today, today, we are going to be talking about one of my favorite topics, which is data warehouses and data architectures. And really everything that's kind of new and changing and different in this increasingly vibrant and important strategic aspect of information technology. I will be your moderator for the next 15 minutes or so my name is Adam gross. I am an investor and board member, but previously worked at companies like Heroku, and Salesforce and Dropbox, and a lot of experience building, operating and using these kinds of systems as well, which maybe I'll pepper into the discussion. With that maybe we'll take a minute to introduce our distinguished guests. And perhaps we can start with you, Ben.
Ben Gottfredson (1:00)
Thank you, Adam. Absolute pleasure to be here. My name is Ben Gottfredson. I've been with Snowflake for almost seven years now. So not only Snowflake in that time really gone from bootstrapped startup to the enterprise it is today. But I've noticed a ton of major transformations in that same period. So Adam, like you love discussing this topic, and impressed by the staff, we have to actually discuss it today. So, honored to be here.
Adam Gross(1:27)
Great, thanks for taking the time to join us. Soumyadeb, maybe we can go to you next.
Soumyadeb (1:34)
Hey, this is Soumyadeb. And founder and CEO of RudderStack. Really excited to be here in this panel on Snowflake and total, like data stack at a very high level is building the next generation customer data platform for for a data warehouse first world. So this topic is very close to my heart, big background have been an entrepreneur, like sort of my previous company to eight by eight. And that's some of the pain points in that journey, like prompted us to start a stack. And I have a PhD in data. So I've always worked in data of my life, but data applied to modeling in the last couple of years. But again, humbled and excited to be here.
Adam Gross(2:16)
Great. Thanks for joining us. And last but certainly not least, maybe Jamila, you can introduce yourself. And I think Jamila, you might be muted.
Jamila (2:30)
Sorry about that. Yeah, thank you for introducing me, Adam. Super happy to be on the panel here. And yeah, as mentioned, I'm Jamila, a director of marketing analytics at tonal and in terms of you know, my background have been in data for most of my career started out kind of, you know, with more enterprise level retail, consulting side, and now more on the tech and you know, startup kind of side, for the those of you who may not be familiar with tonal, super cool product, it is essentially a smart home gym, that essentially you can mount on your wall like a TV, it has 200 pounds of digital weight behind it. And it's really adaptive. So there's a lot of machine learning and AI behind the scenes where in the middle of a workout, it'll adjust your weight for you, it'll count for you, it will recommend content for you to a super exciting product. So I've been around here for about nine months. And I'm really focusing on the analytic side at all, but more in the marketing space. So super, super pumped to be here. And yeah, can't wait to chat more about this topic.
Adam Gross(3:29)
Great. Thank you. So it is an exciting time in the data infrastructure space. It's an exciting time for vendors, for customers, for people working in it. I think that's reflected in the energy we see and kind of new companies in new applications. It might not be kind of clear to everybody, kind of what's different. I think many of us have used and worked with databases and data warehouses for a long time. I think why we're here today is to talk about all the things that are enabled with this kind of new generation of products that are emerging to cover fundamental changes in how databases and data warehousing works. Ben, you're an old hand at Snowflake, probably the pioneer in this new model. Maybe we can start with you and just talk a little bit about what's changed from a kind of technology point of view, what's different now than the previous generation that's kind of enabling all this kind of new stuff?
Ben Gottfredson (4:29)
Yeah, it's a good question. So, the first one that's obvious, disappointed, but it wasn't obvious in 2014 2015 is the impact of unlimited capacity coming from the major clouds, and what that would turn companies and startups that were very data-driven. And then what it would turn them into just by leveraging that public cloud capability like countless stories of my time at Snowflake where we watched a company go from 10 gigabytes to 100 terabytes. And they didn't even really think twice about it just kind of happened organically over the course of nine to twelve months. And all of a sudden, they're a data-driven company with what two years ago would have been, you know, an absurdly large data environment. And that just repeated many times over. So I'd say what the public clouds enabled is a big driver, I think simplified toolsets is a big driver. I remember going to Hadoop conferences in 2014, and 2015. And it's pretty overwhelming the languages you needed to know to get that thing. In today, it's kind of unified across toolsets that are a little bit easier to understand and kind of lowers the barrier to entry for using tools like Snowflake and other data platforms like it. And I think the third point would be new data types really come about right? Like, it's not the same super structured datasets in the past that you can analyze. There's a lot of semi-structured or unstructured data that companies can start leveraging that was really difficult to it's not impossible before.
Adam Gross(6:11)
So it seemed some, one of the kinds of foundational differences between this kind of new era and the previous one is the kinds of databases and data warehouses we're talking about now, are cloud-only, I can't, when you told me about got this right. I can't install Snowflake on-prem, I can only get it on a public cloud. And by virtue of that, I'm able to take advantage of a kind of a very different infrastructure and economies of scale. And obviously, kind of flexible. Consumption that's important. And is, is that accurate with that kind of foundation of this kind of new models, it's going to be a collateral.
Ben Gottfredson (6:54)
100%. And, again, going back to the early days of Snowflake, that was a contentious point. And I think in 2021, it's not as contentious of a point, we had a lot of big banks and institutions that were super hesitant to consider anything on the cloud. And it basically said, come back to us when you guys have an on-premise solution. And over the past six-seven years, it's kind of gone the reverse trend, where they're now finally getting comfortable with cloud adoption. So yeah, I mean, that's the future. And that's no negotiation point is how I'd see it. But I'd be here curious to hear the rest of the panel, you that point as well.
Adam Gross(7:33)
Maybe that's a good opportunity to go to Jamila. And somebody I went to Salesforce a long time, Dropbox Heroku, all cloud-only companies are familiar with the challenges of kind of enterprise adoption of cloud, you've had a long history of working with different environments. How have the organizations you've been at thinking about this kind of cloud-only approach, and security and governance questions that come with it?
Jamila (8:02)
I can definitely tell you it differs based-off, of industry, for sure. So if I kind of, you know, rewind back, you know, 10 plus years ago, it was very transactional, you store data based on transactions, you wouldn't necessarily look at, you know, customer attributes, or you know, store too much information, you kind of have the bare minimum of what you need. And it would primarily be, you know, financial reporting, you know, annual statements, things like that, and you wouldn't really be seeing marketing or supply chain, you know, teams using data as much as they could, today, there's a lot more, I think, smart decision making that goes behind it, you know, before they maybe didn't look too much into you know, buying patterns at a daily or hourly or even like store level, it was very aggregate, you know, you buy involved, even TV, and, you know, when you're bidding programmatically for advertisements, before it was very much like, once a year, you know, once a quarter, you really plan ahead of time you buy in bulk, and it's very manual, you know, you're making deals with people. Now, it's all about systems and algorithms, and everything is happening behind the scenes, even like with website data, that is a completely like, a new form of data, and the volume is crazy. There's a ton of data coming in, in real-time really quickly. And so this is a big change I've seen is, you know, when I started out, it was, you know, primarily financial data, you know, you're spending time on a spreadsheet, you're downloading it from some on-prem, you know, database, it could take, you know, days to, you know, get what you need, you know, massaging the data. And then if you have to do it, again, it's a manual process. Now, it's kind of automated, where, you know, you can kind of set up event stream, you know, using tools like RudderStack, for example, dump them into a tool like Snowflake, where you can then, you know, leverage other tools on top of it for data modeling. I know DBT, you know, is one of a fairly popular one, you know, leveraging tools like careful, for example, as well, and you can kind of have repeatable processes sending data forms, and now decisions are being made with algorithms in real-time. And so this is, I'd say, like, probably one of the biggest shifts that I've seen, where now you can just do so much more in so much less time and the velocity and volume Data has really exploded now to speak to the industries. And, you know, I've worked in the retail side and on the telco side, and I can definitely say, you know, on the telco side, there is a lot more regulatory requirements. And so, you know, for them in the transition phase was very, you know, traditional on-prem than they did the whole, you know, has Ben mentioned Hadoop, you know, data lake in house, still on-prem. But it was painful, you know, it's still very slow, still really clunky. And then now, you know, in the same boat where it's okay, we want to move to the cloud, let's POC it, let's use digital data to try it out. Because digital data is, you know, on-prem doesn't work for that you need something faster, and something better. And so we kind of tested out, but still getting, you know, the enterprise-level contracts, and the approvals and privacy and legal, like all of that two years to kind of figure out. And so that's still a barrier, I think it's definitely becoming less of a barrier, as we're seeing, you know, a lot more governments even starting to move on to the cloud. So I think there's definitely a lot of shifts happening.
Adam Gross (11:01)
That's right. And a good segue, maybe to talk a little about, again, kind of what's enabling this transition from the kind of a product and technology point of view, maybe I'll ask you to chime in here, Soumyadeb. Jamila mentioned Hadoop. Hadoop was kind of the last great hope for transforming all the ways we were going to use data. That's I think, different than this new generation of tools like Snowflake and other cloud data warehouses. What happened is, it's new to the picture, is this, something that's different enough? So kind of why now? What's different for folks who might have been kind of sold out?
Soumyadeb (11:45)
Yeah, I think like, I mean, the, there are two main things in my mind, right? Number one is like Hadoop, never do the- Hadoop is a technology, right? It's like some MapReduce or like some kind of storage technology. So like, and some of that is still driving the cloud providers like not exactly maybe the s3 is of the world, and so on to kind of build over the same technology. It was, I think the main challenge was the deployment model. There's nothing fundamentally wrong with Hadoop, but like how people were deploying Hadoop, you set up a server in your on-prem, data center, and you manage that, and so on. So like, it was kind of painful to do that, compared to like, let's just put all your data in AWS and AWS takes care of everything, right. So thatās kind of one thing. Another big challenge was like the compute part of it. I mean, like, storage is one part, but like compute itself, how do you scale up-scale down? I mean, you don't need the form of machines, every like you're doing some ml computation, it runs only for like, a couple of hours a week or a day, right, I mean, do you like provision your compute for the entire peak capacity, again, that was a big challenge on the board, it kind of goes away in the cloud. So I think it was less about the technology and more about the deployment model how people were using to do versus like, what cloud and it was kind of, in the model.
Adam Gross (13:08)
I guess it kind of depends on the deployment. But typically, you're kind of styling statically provisioning, a whole bunch of machines that you got to keep around the whole time, as opposed to kind of this next generation, sometimes called serverless, meaning, you know, you only are consuming compute and as you need it to run queries. And of course, then it's just going to be a whole lot more efficient and cost-effective than kind of keeping the infrastructure hot and ready the entire time.
Soumyadeb (13:36)
Yeah. And in fact, like Snowflake kind of took it to the extreme where you can literally provision compute, run a SQL query and bring it down, right, as opposed to like, provisioning a data warehouse. So that kind of flexibility really enables use cases that you could not do cost-effectively in the previous world. Like, if I have a very costly query, I can just provision the biggest instance on Snowflake and just pay for it, the minute it runs, which was absolutely not possible.
Adam Gross (14:01)
You mentioned that, I am sorry. Go ahead.
Ben Gottfredson (14:04)
I was just gonna say, I think there are a few interesting applications of that elasticity, especially for marketing analytics, and one of them is the separation of compute and storage, you don't have to be concerned with limiting how much storage you're actually keeping in your data platform. And so you can act on more time-sensitive data sets, and not worry about cycling anything in and out. And then like Soumyadeb touched on elasticity on compute, really can help on more complex workloads, spin up to, you know, an environment that is much larger than you typically need for X amount of time to get that job done. And that's a common use case in the CDP marketing landscape, at least that we see.
Adam Gross (14:47)
And one of the interesting things you mentioned as well there, which is a difference from the kind of the previous and new generation is tools like Snowflake are SQL native? I don't mean to, you know, I'm not writing a MapReduce job in some esoteric language. And yes, there were tools and to do that, it kind of translate that. But this is kind of a sequel first sequel only model, which has the benefit that most organizations and most data people kind of already understands how to use those. Which brings kind of interesting question, maybe go back to you Jamila on this kind of curious, both, you're talking about the kind of business applications of this right, moving from just capturing kind of very corresponding transactional data to having kind of a much richer, broader, deeper, real-time set of data for an organization? I'm curious how that impacted the organization. One, technically, in terms of, you know, how can you as an organization, what have you seen how they evolved in order to be able to take advantage of this because that's a pretty non-trivial shift. And then even more importantly, from a business point of view, being able to adapt to leverage these new models, these new all this new data is an even bigger shift. So I'm kind of curious about your thoughts on both of those.
Jamila (16:11)
Yeah, for sure, I think I can kind of maybe speak to this from an organizational structure standpoint. So I remember, you know, back in the day, when it was purely transactional, it owned everything. Now you look at it, it doesn't own everything, typically, what you'll notice is that different teams have different use cases, they have different speeds at which they require different things. And so there are more DevOps teams, there are more, you know, software engineering, productivity, engineering teams that support like real-life products, where, you know, you need to have up times and, you know, kind of modifications and different API's to connect to, to enable services to happen. And then you also notice now that there are data engineering teams, there's data science teams, these teams didn't exist back in the day. And I think this is probably, you know, the result of the proliferation, we have so many different new data sources, and a new kind of hybrid skill set is needed. And so that's kind of one of I think, the biggest things I've seen from an organizational standpoint, in terms of what's changed, the use cases, from a business standpoint have also changed. I know, historically, there's typically, you know, you see a lot of friction between IT teams, you know, and business teams, you know, the business wants something, it says, Oh, we'll get back to you, you know, it a long time passes, and then you kind of you know, you're behind competition now. And so, I think from a business standpoint, you know, being able to at least POC different things and get things up and off the ground running quickly, is why they started developing these, you know, data science and data engineering and you know, DevOps teams, because this unblocks people, but you still have a little bit of governance, where you know, you do have different teams playing in different areas, but it allows you to be more flexible. And so that's one thing, you know, if you want to get a quick example would be you know, you want to get a model up for people that are on your website, and you want to be able to score people on your website, based on certain metrics to understand that they're likely or not to do certain actions. And this, you need to have a web analytics team to kind of track stuff on the website, you need to have a data team to kind of capture that data and store that data, you need to have an engineering team to kind of massage that data kind of know, put it into a place where you can actually access it. And this is kind of where the data warehouse comes in. But it's at the center of it. All right, once it's in the data warehouse, then there's more work needed, because you need to then manipulate the data, you might need a combination of SQL, maybe Python too right, and then you got to send that score somewhere else in real-time and enable it back in the API back end service. So that, you know, you can actually then introduce content and you know, flip things around based on what people see when they come to your website, for example. And so that this is a really huge change. I think that wasn't really there before.
Adam Gross (18:45)
There's a real tension there between, you know, kind of the old centralization IP model, which I think probably most of us see or have seen and experienced the limits of with a desire to kind of push that out to the different business units and kind of business owners and kind of maybe more decentralized that. But then, of course, you run it all the problems of repeating and technologies, you have all this heterogeneity, which can be hard to manage, what what what diamonds, would you give folks in terms of how to think about creating a structure, create an organization in terms of ownership, to enable these kinds of next-generation applications to be able to take advantage of all this stuff and data inside of organizations?
Jamila (19:32)
So yeah, typically, what I've seen is, you know, marketing teams might say, hey, we want to try something out. And you know, you work with legal your privacy to get all the contract signed. And I've actually seen sometimes that you know, marketing teams or product teams, typically they introduce new tools, and they start leveraging the tools and then at a point you know, once it becomes big enough and it's proven itself out then you kind of work with it to say like okay, look, we now need to integrate This into kind of our workhorse, and it's now a priority because they can kind of see the impact that it's having on the business. That's what I've seen, in my experience. I mean, it might be slightly different. So curious to hear, you know, like anything on your end as well.
Ben Gottfredson (20:15)
Yeah, I think, Jamila, you had a point earlier, and you said, you know, then it goes to the, to the warehouse for analysis, right. And that's if you're lucky. That's it, the data is not siloed into various SaaS applications. And our CIO wrote an interesting internal blog about this. But just because SAS application is on the cloud, doesn't mean it's unified on your cloud ecosystem in a cloud platform. And what we see a lot and I think what RudderStack actually comes into the frame, and is when data sets get completely siloed, onto different SaaS applications. Any work that's done on that single SaaS application is going to be only unique to that SAS application. So ultimately, what you need is a horizontal view across your Sass apps, and not a vertical view that's dependent on one new application that you bring in to solve one exact use case or workload. And so having some single source of truth, which is made a lot easier with the tool like RudderStack, makes the communication between the data teams between the company a lot more seamless, and that's something that we're you know, especially in the last five, six years having to retroactively solve for. And it's something that a smart team to be pretty forward-thinking about.
Soumyadeb (21:36)
It doesn't double down what like Ben, you're saying I mean, you've seen use cases like the churn prediction, you're trying to predict which of the customers are going to churn. And that requires bringing first-party product usage data coming from your apps, and, and so on. But also like third-party data locked into like your ticketing system like Zendesk or your CRM application, right. And then you need to unify all of our data to build these kinds of applications. And you cannot like you cannot do it in Zendesk or any other tool in isolation, you have to bring it into a data warehouse to build the next generation of applications. So yeah. And then after you have that score, you have to push it back into some kind of a tool where you can take the action on top of the data, right? Whether it's something like a customer management tool, or like Salesforce, where you're tracking a customer, so these kind of new-gen architectures required the warehouse to be at the center of it. Although, Adam, back to your point around like SQL, I think like the jury's still out like is SQL powerful enough to, enable all these applications? I know you're smiling as your favorite topic. But yeah, do we? What will SQL dry? What will spark drive, I think, like, hopefully, at some point will support link with support for Spark and so on. So yeah, it's still out. I think DBT has kind of layered, like taken sequel ahead in terms of adding programmatic abstractions. But then again, like, is it enough to build some of these complex applications and...
Adam Gross (23:00)
Iām pro, you know, I even throw some Python in there personally, myself, occasionally. I think it's, it's more about making simple things simple and the hard things possible. And I think the new model probably suffered from making some of the simple things unnecessarily hard. So, I think it's also refreshing with, you know, tools, like RudderStack and Snowflake and DBT, just the kind of democratization of the skills and technology making much more experience, making much more available, maybe better attention to the experience, and ultimately, people engage in this stuff. So thank you for allowing me that editorial opportunity. I'll ship it back to maybe something that people actually care about, you know, Ben mentioned, CDP's, sort of that you're talking about kind of the operational data warehouse, which is kind of this idea of a new model that's enabled by bringing in data from all the places, but not just kind of traditionally thinking about that as a place to run reports or do analytics, but actually do you know, business-relevant computation, which is then pushed back out to other systems? Maybe talk a little bit about how RudderStackās approach is different than some of the other kinds of approaches in the broader kind of CDP space? That's existed for a while.
Soumyadeb (24:24)
Yeah, that's what I'll start with at a very high level, we are kind of building the CDP around this data warehouse epicenter, right? Like none of the other CDP vendors were like, purely SaaS applications selling to the marketing teams, the idea that you sell everything to them, and they will give you the tooling required to like let's say, create audiences and like predictive audiences and so on, and then you can activate that data, but you never get access to the raw data. So for example, what you cannot do is connect, let's say Tableau or Looker and build charts on top of that or build more advanced ml use cases or even like marketing attribution use cases? So that's kind of the traditional CDP approach. What we are doing is saying that you should have complete control over the data in your data warehouse, so that you can enable this kind of application. At the same time, you need to do some basic things, you have to do identity stitching, you cannot just dump the raw data and leave it everything, we leave everything to the customer to like, kind of figure that out. So you need to do some basic hygiene, clean, clean up governance, data quality, all the data, but like, enable these kinds of use cases, on top of your data warehouse.
Adam Gross (25:27)
Jamila, is this idea of kind of the operational data warehouse and you heard Ben talk about it as well, is this kind of an explicit strategy inside of organizations you've worked in? Or is this more like us, it's kind of happening in the background, and we're getting lead there eventually.
Jamila (25:41)
No, it's a key pillar. We definitely I think, as organizations want to own our data, we don't want to have third-party vendors own our data. So that's kind of a key, a key piece of it. Working with a vendor, like RudderStack definitely has that benefit. The other part of it is identity stitching, sometimes be a black box, you don't know how better for doing it. You don't know if they're doing it, right. And so by being able to have your own data, you're able to kind of validate, and ideally, you have the team, you know, in place structure in place to be able to do this, if you're No, maybe a company that doesn't have someone who has the skills to do identify searching, then that can be a roadblock. And this is where SAS vendors may have been really helpful. But I think in all my experiences, this is kind of, you kind of want to do in house, you want to have that, you know, each company, if you think about it has their own way and their own identity graph, right? Like, you might have, you know, your own authentication service, your own site that you could then leverage to build your models, and you don't know if the vendors are using that same way. So So definitely, I think a key thing and then on top of it enables so many use cases, because now you can pump the data into Looker or or Tableau or whatever visualization tool you want to leverage. Right, you can actually slice and dice the data, you can do customer segmentation, you can you can build brand new KPIs that are not not really like standard KPIs that you get from like, let's say, Google Analytics, right, you can build your own type of KPIs own type of audiences. And then of course, the challenge always is, how do you govern it? And how do you then send that data back to the systems that need the feedback. And so this is also where, you know, this is where the CDP really comes in, right? Because you don't need to have a full up engineer who's rebuilding things reinventing the wheel all the time, when you just want to send something somewhere else. And so this is where you know, RudderStack, they've done all the groundwork, they've, you know, built the connections. So you know, Facebook, for example, right. And so now we can just kind of plug and play, leverage those connections, rinse and repeat for all the vendors that we work with. But we can send in from our central data warehouse, a common definition, and deploy it across multiple different channels. And the other challenge as well is different systems have different requirements in different ways that they need data. And so this is also where I think, you know, you need to have some kind of tool to help you do that. Otherwise, it gets too difficult to maintain. And so that's also where I see CDPs really fitting in is, you know, they've kind of figured out all of that stuff. So they productize that. So you don't need to reinvent the wheel. But that's that's kind of my opinion,
Adam Gross (28:13)
You mentioned that any stitching, which is obviously, you know, a super important problem, every digital business is multi-channel, being able to understand, you know, as you know, how like getting customers interacting with my app versus my exercise equipment versus my website versus whatever the case might be. I think that's probably a universal problem. I guess I would be a little nervous about any given organization's ability to take on a problem like that and say, you know, you know, you've asserted, this is something you think organizations for themselves? It's, it's your guidance to folks, you know, hey, you know, come on in the water's not that bad? Or is there things you kind of need to be ready to, to take on or own when you when you approach online, versus handing it off to the vendor?
Jamila (29:03)
I think it depends on the maturity of the company. So you know, if you're, let's say, Ground Zero startup, and you're building out your customer journey, you're building out your workflows, you got to think about what piece of information can I use at each step to stitch together the user journey? What are those touch points, right, and if you can identify what those touch points are, and you might be in a better position, you can connect that to, you know, a quick SAS vendor and, you know, as long as you're able to connect those pieces and have those pieces there, the vendor can do some stitching for you. But if you're, you know, a much larger enterprise, and you have like, I can give you a quick example in my past where, you know, I've worked at a huge company, they're, you know, really big loyalty program, lots of different lines of businesses and single customer unique identifier across all of it. Right? It will probably make sense for them to build their own in-house because for them the privacy implications number one, giving that data to a vendor to do it is built in Co-Op right where vendors like my throw Adobe out there. But, you know, Adobe has a Co-Op program where, you know, you can leverage them do identity stitching, using their products, but then, you know, at the end of the day who owns that data, right, and, you know, you could opt in to share that data with other vendors. Now, there's pros and cons, it depends on you know, your bank, you might not want to share that data to enrich your customer profiles, you know, with maybe other data, but you know, if you're not a bank, maybe don't have as many regulations. You know, you might be like, Hey, this is a selling feature for me, I'm gonna go in and, you know, that might be something that would be beneficial for them to help with identity stitching. But I think it depends on the business, right? If you don't have authentication, and if you don't have the necessary means in place to stitch your own customers, and you can't do it yourself. It's deterministically and probabilistically. Like, it's a very, very tricky slope, right to go down. So it really depends, I think, on the company. So sorry, I'm not going to be able to give you an explicit answer. But I think it's a, it's circumstantial, you know, depending on where you are.
Adam Gross (31:07)
it's a really important issue. And it's a little bit of the kind of the holy grail of, you know, some of these bigger data marketing systems. So I think it's, it's helpful to point out, maybe we'll shift gears a little bit in my remaining time, we talk a lot about kind of the infrastructure and the organizational issues, maybe we can just talk about some of the cool stuff. And I'll start again, with each one, maybe it doesn't have to be the most impactful thing, but just something maybe cool or interesting that you've seen you built or whatever organizations is working on as a result of taking advantage of these new steps.
Jamila (31:39)
Yeah, I can definitely say like, personalization is definitely a key thing. And so when you take a look at what content are people doing on the website, you know, what products are people viewing on the website, as well as apps, you know, throughout the entire space, being able to actually put groups of customers into different segments, and then layer on, you know, different propensity model scores that you have, and then figure out what you recommend to them. And, you know, getting those scores in real-time, you know, added to the data layer of the website, and then you're able to actually connect your content management system with what you see in the back end, from the data science work. And I think connecting those two that's, that's super cool, right? And it's very hard to do. It's not easy to do by any means. You think it's something simple, but really, it's not. And so I feel like that's probably an example I can share.
Adam Gross (32:26)
But doesn't pretty well be curious at some point. Learn more about that. Ben may be kind of similar question to you. You mentioned CDP marketing, operational data warehouse, kind of common use cases for personal like, any interesting examples of how I've seen this kind of ultimately, what do you use the effect of?
Ben Gottfredson (32:46)
Yeah, I mean, the interesting thing about Snowflake is it just kind of crosses over every industry you can imagine. I think the trend that is really exciting right now, it's maybe a little specific to us is the ability to share datasets between two different Snowflake users. And so you can think about how that might play out in a partnership between vendor and their customer. It just kind of really reduces the friction if you're able to share a lot. We're able to the dataset from the vendor's account to the customer's account without having to move in. And where I've seen it really make an impact and be interesting on my side is the reduced friction they have on their sales cycles. So instead of asking them like a siloed, isolated SAS application would have to like an old school CDP where they'd say, hey, you need to trust us, you need to send us this dataset, we'll put it on our system, then we'll provide it back to you. Instead, they can just share the dataset that's already living and existing in their Snowflake account, just by giving credential access. So that shift has made things a lot easier. And it's cool to see it play out when a customer of Snowflake is able to win a deal for themselves faster because it.
Adam Gross (34:07)
Yeah, that's super interesting. And maybe another topic as well to explore later, and Soumyadeb, Iāll ask you kind of the same question favorite customer examples are kind of cool apps or use cases you've seen being built with putting together all this data and hard work.
Soumyadeb (34:23)
Yeah, personalization is definitely one of that. One of the things I point out is like one of our customers build a churn model again, using the data that is being collected through RudderStack, and like they are a mobile game and they are trying to predict like, which are the customers who are likely to churn and like, if somebody is churning they can give them free coupons and bring them back in that. And like using the simple model that built they could increase the revenue by 10%. Like this whole thing, took some effort in terms of feature engineering, but like it like end to end this was put together within a week and that drove up the revenue by 10%. So these kind of use cases are like enabled by Like what tools like RudderSrack, but also like the cloud data warehouses like Snowflake and, and the democratization of these models also, like building, there's a lot of innovation that we didn't talk about as they had has happened in the MLs best itself, which kind of makes these models very easy to develop, deploy, and so on.
Adam Gross (35:20)
I'll give you a chance to kind of chime in there. What kinds of tools are you seeing people typically use on the ML side? In conjunction with these architectures? If there was a place for an organization to start? Would you have a recommendation?
Soumyadeb (35:37)
Yeah, so. So there are two broad approaches, right? I mean, some of the data warehouses have native support for like ML these days. So you can like train a model just with SQL. So that's kind of one set of like, accordingly, like smaller startups do that first. And then like as the company scales, it's usually like the spot for reading down the data into some kind of a data lake with the data breaks or whether it's like s3, and so on. And then, like, build your spot models, I guess, like it's both smaller companies will start with the warehouse, bigger ones are in the s3 world. I think the holy grail would be when you could like really run Spark very easily on a cloud data warehouse. And that's, that's the word I'm reading for.
Ben Gottfredson (36:25)
Adam, I'd be remiss if I didn't add the cool use cases, tonal. I mean, next-generation gym built on a wall, I don't think you can get a cooler use case than that.
Adam Gross (36:39)
Oh, no, I mean, I'm doing this because I'm hoping to kind of finagle it somehow. So I think like a lot of us, we got addicted to our internet-connected fitness equipment. So you don't need to sell me twice on that. We're getting a little close towards the end of the session here. I'll quickly check to see if there's any questions people in the audience have. And I'm happy to put any of our guests in the hot seat. And so we can make it uncontroversial too? And if not, I'll just do my best to make that happen. So a question at all, to everybody. When you think about kind of moving towards this model, this operational data warehouse model? And, you know, maybe you're moving from kind of traditional siloed stuff. How should a company think about still being on human resources? And maybe I'll start with you Ben on that one? Yeah,
Ben Gottfredson (37:45)
I think it depends how I guess it depends where the the stage of the companies had, right. So they have a ton of ingrained processes and logic that's built out over years. And even cloud tools are worse off on-premise tools, it's going to be a more complex shift. Now, if the tool is already, or if the companies already live on the cloud with cloud-native apps, and not living with a ton of tech debt, the ship can be incredibly simple. So it really depends on the complexity built out on the existing systems. And for newer companies, obviously, that's going to end on the side of less complex migrations.
Adam Gross (38:27)
Can we have any advice on starting out phases, and how to think about there being are things to avoid?
Ben Gottfredson (38:34)
Yeah, I'd say, outsource what isn't going to be a core competency, when you're building things out. Just one example I've seen a few times is for your ETL process, a company might really try to just build that on their own that connector from some data source to their warehouse, and the amount of blood sweat, and tears that go into trying to make that thing work and run consistently. Every time at least I've seen it as outweighed with the potential costs and bringing in a vendor, you know, would have been. So make those smart trade-offs upfront. And think about what the initial investments going to reap for you in the long term, versus just trying to solve things around up front.
Adam Gross (39:23)
Great, Soumyadeb, I got one for you. We have these new data warehouses, we have tools to load data into there. And we have great new things we can do with the data. But increasingly, as we kind of want to use this data more and more in our applications, latency becomes a problem in terms of kind of the whole system. How long does it take me to go from the source system get loaded into the data warehouse? What are you seeing, what do you recommend? What do you think about kind of getting towards real-time in some of these flows? And how are you thinking about that RudderStack?
Soumyadeb (39:59)
Yeah, I think So like, firstly, I have like a few points that people talk a lot about real-time, like, it's a cool thing to talk about. But there are very few use cases which really need like real-time data. Maybe personalization is one of that. But that's kind of one. The other thing is like, I mean, if you look at data warehouses like this, this, I mean, it is actually pretty, you cannot get to like millisecond second level, real-time. But otherwise, you can put like, pretty real-time. And so the minute level of like loading data into Snowflake, and like, doing something with the data, and then activating that data backward, so your customers doing that around, like, on top of Snowflake. So I think, like, again, if you really need millisecond-level real-time, then you have to like put like a separate stack, and on some kind of in-memory key-value store or something. But for the majority of the use cases, like Snowflake is slick, perfect, right? Because with the streaming load, and so on,
Adam Gross (40:59)
I think you're probably being generous, imagining what people's experiences are, in terms of latency, I have worked at some very large-scale tech companies where we have 30, day latencies on some of our data. So kind of what I'm hearing is, it's realistic to get to a minute, but it's not realistic with these architectures, it's probably not realistic to get below that kind of resolution, you're probably going to need to look at a different stack of technology. And of course, you know, we can, you can probably think about which applications fall into which bucket Exactly. As they say, all consistency is eventual anyway. So, you know, even even in real time. Okay, we have a couple more questions. Um, if all of your data, we're thinking about kind of combining all the data into one data warehouse, how do you think about kind of point solutions? There are a lot of kind of analytical tools that are kind of point solutions that always require their own data. How do those in this picture? Do they go away? Do they complement work better with this architecture? Assuming that I had some experience here, too. So for that one to you.
Soumyadeb (42:26)
Yeah, I think like, like, eventually, like this point solutions will the same take Google Analytics, right? I mean, why would you have to like send data to Google Analytics to generate those reports, like your data is, you're already dumping the data into Snowflake. And there should be like a, like a tool like that just runs on top of Snowflake and gives you the same set of reports and, and a lot more flexibility around being like, customize those reports and run SQL on top of it. So, unfortunately, the world is not there yet. But it is going there. Every data will be centralized in cloud data warehouses. And the point solutions will be like tools on top of that, you still need some engagement tools. So for example, if you have to send an email or send a push notification, and so on, you'll still need like, go and execute that. But then that should be like just a transactional thing, which executes that thing. Centered source of truth should be data stored in Snowflake or cloud data warehouse.
Ben Gottfredson (43:21)
Yeah, now that you want the point solution to integrate really nicely and run on top of the warehouse and leverage the warehouse for storage and for compute, and it comes back to the concept of avoiding data silos, wherever you can. And if you're having to send your data out, it's creating a silo, right? And the, wherever you're sending, it is unlikely to have the same performance as the warehouse, or you're shipping it out. So keep that in mind.
Adam Gross (43:51)
Jamila, can I ask you, you know, we can talk a little about kind of ml tools. And I'm curious, which you've, if there are any, that you've been using any of the organizations, you've been part of, that you've had success with you, you know, kind of see some of these other, you know, ml looks like spark or databricks as part of the stack and augmenting these data architectures.
Jamila (44:18)
Yeah, so my experience is very, very biased towards Google Cloud. So like natively, using, you know, the tools that are available within there. So I'm not gonna go into more detail on that, but pretty much exclusively, we've been, you know, leveraging tools, you know, BigQuery for storage of data, you know, big table for kind of some of the more semi-structured unstructured data, as well as, you know, kind of, you know, within BigQuery there's like machine learning applications, there's cloud AI notebooks, you can kind of leverage that with Kubernetes and compute clusters, you know, which that part I had to, you know, work with DevOps to configure it, like, kind of out of my realm a little bit. You know, and then from there, back-end services and API's connect, and that's kind of where the product team comes in and you know, kind of pulls in that data. So that's kind of my experience. But yeah, primarily, like, you know, cloud-based Google stack, you know, a little bit of, you know, you probably, you know, you can leverage spark Python, like through the cloudy I know, book, and, you know, leverage the typical stuff that you'd see. You know, the pre-built, you know, algorithms already do exist. So that's kind of my experience.
Adam Gross (45:28)
There's a lot of different stuff out there, especially in the ML world, and it can get a little confusing. So, definitely worth investigating some options there. Ben, I think you mentioned this, and I'll sell directly to you. There's a question about the kind of data enrichment? And what kind of use cases are you seeing around folks augmenting data inside of Snowflake with the original buyers?
Ben Gottfredson (45:57)
Yeah, it's a fun, fun prompt to talk about, we have a data marketplace that is adding 10s of new data sets every week. So it's in the hundreds now. And the one that I think it's been most interesting over the last year and a half, two years is our COVID-19. Data tracker, which was free and available in our data marketplace. That's the, you know, example that was getting the most use at that time through our marketplace. And then I think the segment that we've seen growing fastest has been a time-sensitive financial data set. Knowing that, you know, hedge funds, or maybe the biggest consumer of them, they want to be able to act and trade on datasets, you know, in real-time, or as close as they can. And data sharing provides that opportunity and sending off the old school method of having to share it to a data provider, or should we get it from a data provider. So I think naturally, that's kind of where we're seeing the most acceleration in it in the finance world. But, but there are also these cool edge cases that are being applied to like the COVID-19 data set.
Adam Gross (47:08)
Great. I'll resist the temptation to talk about how much I was trying to get to buy annuities. But that for another day, we have a couple of minutes left, maybe I'll just ask our panelists to kind of share any closing thoughts based on the questions and discussion they had? Everybody be successful out there. Maybe, Jamila, we'll start with you.
Jamila (47:34)
That was me. Yeah. Yeah, closing thoughts. I mean, I think it's definitely, you know, moving towards the cloud, definitely moving towards more real-time, but I would say real-time, like, in my, in my opinion, is usually not real-time, you know, you're, you're probably serving content in real-time. But the scoring, the modeling, the training, that typically all happens, you know, on a training set, with historical data, typically what I've seen, and so, you know, you may not need every component to be real-time, but there's definitely a lot of use cases and like, I want to, I want to RudderStacks horn a bit. But, you know, we've been leveraging RudderStack, it is a very cool tool. You know, CDP's, just, generally speaking, are very, very powerful, you know, with the data, being able to own your own data, being able to have your own use cases, you know, as long as you have the right team in place, and you have the right analytical talent, and the support structures, you know, DevOps, etc. It's definitely worth kind of going down that road because your warehouse is at the center, but you kind of need the data coming in, and the data going out to people to enable your use cases. So that's my closing thought.
Adam Gross (48:41)
Great. Ben, any parting thoughts in our meeting notes?
Ben Gottfredson (48:47)
Yeah, just keep it simple. Try to keep a single source of truth, you know, in the data platform you're using, and then, you know, bring in a tool like a RudderStack that allows you to go vertical across your Sass apps instead of siloed data that's horizontal, confined to each app. And if you make these trade-offs, you know, upfront, you're going to be paying for wireless tech debt. And so I've seen over and over again, so if you keep it simple at the beginning, it will, by and large, stay simple, even as it gets more complex, and you're doing more interesting things with the data sets. You can really avoid painful situations in the future. So keep it simple is the parting advice.
Adam Gross (49:29)
Soumyadeb, for you?
Soumyadeb (49:32)
Yeah, I'll second what Ben said, like about data silos keep control over your data, like, particularly when it comes to customer data that will pay off in many ways in a long way. Long Run, like the kind of use cases you can build and like the kind of like the business benefit of doing the deck. So yeah, avoid cycles and silos.
Adam Gross (49:51)
Well, thank you very much to our panelists for taking time and to our audience for joining us. I hope you found it useful and look forward to learning more about what you guys built.