What is Reference Data Management RDM?
This article explores the intricate world of reference data management (RDM), a discipline that ensures standardization across diverse data sets and systems.
Effective RDM is not just about maintaining lists; it's about sustaining a framework that enables seamless connectivity, clear communication, and operational efficiency. As organizations grapple with increasing data volumes and the need for data synchronization across global operations, the importance of robust reference data management becomes undeniable. From improving data quality to enhancing regulatory compliance, RDM plays a pivotal role.
We will delve into the strategies that make RDM a success, the challenges it helps to overcome, and the impact it has on the broader landscape of data governance. Whether you are a data management professional or a stakeholder in your organization's data journey, understanding RDM is critical for turning data into a strategic asset. Join us as we uncover the layers of reference data management and its indispensable role in today’s data-driven business environment.
What is Reference Data?
Reference data is crucial in every sector for categorizing and structuring various types of information. Consider, for instance, the Dewey Decimal System utilized in libraries. This system organizes books and resources based on subject matter, author, and other attributes, acting as a framework for reference data in the context of library science.
Another illustration is the International Standard Book Number (ISBN), a unique identifier for books. This 13-digit code is essential for tracking and managing books in the publishing industry, from production through sales and distribution.
These examples highlight the role of reference data in imparting essential context for organizations. However, without proper management, this reference data can become a source of confusion and declining data integrity.
To address these challenges, Reference Data Management (RDM) plays a pivotal role. RDM ensures that all reference data within an organization is accurately categorized, defined, and readily accessible, thereby enhancing communication and maintaining data quality across various business processes.
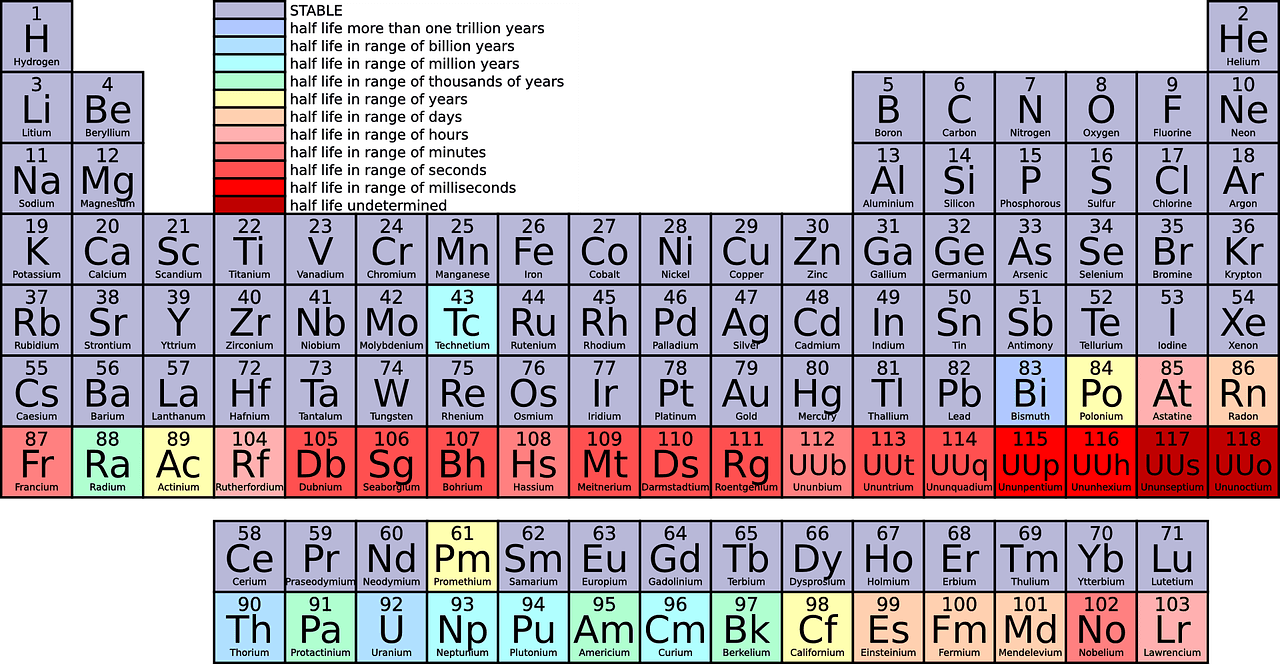
What is Reference Data Management (RDM)?
Reference Data Management (RDM) is a comprehensive and structured system designed to efficiently manage and maintain reference data within an organization. Reference data, often considered the backbone of data organization, encompasses a wide range of critical information that is vital for the smooth operation of a company. RDM, as a strategic approach, involves the organization, updating, and consolidation of reference data to facilitate various aspects of business operations.
RDM can be categorized into two primary types: internal reference data and external reference data. Each of these serves distinct purposes and plays a pivotal role in ensuring the effective functioning of an organization.
1. Internal Reference Data: This category encompasses company-specific information that is unique to an organization. Examples of internal reference data include product codes, transaction codes, and other data elements that are tailored to the specific needs and processes of the company. Managing internal reference data is crucial for maintaining consistency, accuracy, and efficiency in internal operations.
2. External Reference Data: External reference data is data provided by external organizations, standards bodies, or regulatory agencies. Organizations often rely on authoritative sources like the International Organization for Standardization (ISO) for external reference data. This type of data includes standardized information such as country codes, postal codes, and state abbreviations. Adhering to external reference data standards is essential for achieving compliance with international regulations and industry norms.
Reference Data Management serves multiple critical functions within an organization:
- Consistency and Accuracy: RDM ensures that reference data remains consistent across various departments and systems, reducing the risk of data errors and inconsistencies.
- Compliance: It helps organizations adhere to international standards and industry regulations by incorporating external reference data that reflects best practices and compliance requirements.
- Efficiency: RDM streamlines workflows by providing a centralized repository for reference data, enabling quick access and updates.
Reference data can be further categorized into two varieties:
1. Multi-Domain Reference Data: This type of reference data is versatile and applicable across multiple industries, business units, and content types. It serves as a common foundation for various functions within an organization, fostering data integration and synergy.
2. Real-Time Reference Data: Real-time reference data is particularly relevant in industries like capital markets and Internet of Things (IoT), where timely and accurate metadata is critical for decision-making and operations. It ensures that data is always up-to-date and accessible in real time.
In essence, Reference Data Management extends from Master Data Management (MDM), which focuses on unifying and centralizing core organizational data, such as product information, customer profiles, financial data, and other critical assets. RDM takes this master data and further categorizes it into subgroups based on specific attributes or characteristics, making it more accessible for processing, sharing, and enhancing workflow efficiency.
Reference data encompasses a wide range of identifiers and attributes, including:
- Location Attributes: These include country codes, postal codes, state abbreviations, and geographical data that assist in precise location-based analysis and operations.
- Product Codes: Unique identifiers for products or services that facilitate inventory management, procurement, and sales tracking.
- Pricing and Transaction Codes: Codes used in financial transactions, pricing models, and billing processes.
- Exchange Codes: Codes specific to financial markets and stock exchanges, enabling accurate trade and investment operations.
- Languages: Information about languages and dialects used in multilingual operations and content management.
- Financial Hierarchies: Hierarchical structures that organize financial data, ensuring consistency and accuracy in financial reporting.
- Currencies: Codes and exchange rates for different currencies used in international transactions.
- Measurements: Standard units of measurement for quantities, dimensions, and metrics, ensuring uniformity in data handling.
- Industry and Classification Codes: Codes that classify products, industries, and businesses according to standardized criteria, aiding in market analysis and regulatory compliance.
In summary, Reference Data Management is a critical component of data governance, providing the necessary framework for organizing, maintaining, and utilizing reference data to optimize business processes, ensure compliance, and drive operational efficiency across various industries and domains.
The Significance of Reference Data Management and Integration
Data serves as the lifeblood of modern organizations, offering critical insights for evaluating business operations, refining products and concepts, analyzing customer interactions, and driving growth strategies. However, the effective utilization of data hinges on its organization, accessibility, and currency. This is where reference data management and data integration step in as indispensable components of data governance.
Challenges Associated with Reference Data Management
Organizations that neglect the management of their reference data encounter a spectrum of hurdles in the realm of data governance, including:
- Manual Maintenance and Spreadsheet Updates: The need to manually maintain various tools and update sprawling spreadsheets becomes a cumbersome and error-prone process.
- Data Duplication and Inaccuracy: The absence of standardized naming conventions often results in duplicate, irrelevant, or incorrect data, undermining data integrity.
- Resource-Intensive Data Gathering: Valuable time, financial resources, and manpower are squandered on the laborious task of manually collecting and consolidating data from disparate sources.
- Challenges in Code Standardization: Assigning and maintaining consistent values for codes becomes a complex endeavor, leading to confusion and inefficiency.
- Lack of Data-Sharing Mechanisms: The absence of effective data-sharing mechanisms fosters miscommunication and paves the way for errors to infiltrate the data ecosystem.
Benefits of Reference Data Management and Integration
Conversely, reference data management offers a multitude of advantages, notably enhancing data quality and usability.
Centralizing control through reference data management offers a significant advantage in ensuring consistency and adherence to compliance standards. It facilitates streamlined access, distribution, and updating of reference data across multiple systems, all within a well-governed framework tailored to meet the specific needs of the business. The effective implementation of reference data management not only enables businesses to expand their operations but also enhances their analytical processes. Moreover, it equips them with the agility to promptly respond to new data demands or shifts in the market landscape, all without the need for a comprehensive restructuring of the entire enterprise data infrastructure.
Reference data management serves as a cornerstone for establishing uniformity within your data ecosystem. By meticulously managing various iterations of reference data and interconnecting them through correspondence tables, businesses can achieve semantic consistency over time and across diverse standards. This consistency is paramount, as it prevents organizations from grappling with subpar data quality and minor discrepancies that may burgeon into costly errors over the long term.
Specifically, RDM provides the following benefits:
- Enhanced Workflows and Data Accessibility: RDM optimizes processes and boosts data accessibility, ensuring that relevant information is readily available to support decision-making and operations.
- Error Reduction and Data Cleansing: Through standardized practices, RDM eliminates errors and inaccuracies, elevating the overall quality and cleanliness of data.
- Efficient Updates for New Reference Data: The integration of RDM enables the swift incorporation of new reference data, keeping the information ecosystem up-to-date and relevant.
- Refined Business Intelligence Reporting: RDM contributes to the refinement of business intelligence (BI) reporting, enabling organizations to derive more insightful and accurate insights.
- Regulatory Compliance: Organizations can meet regulatory requirements effectively by incorporating RDM practices, ensuring data accuracy and consistency.
- Enhanced Interdepartmental Communication: RDM facilitates seamless communication between different departments and stakeholders by establishing common data standards and practices.
- Expedited Issue Resolution: With accurate and readily accessible data, organizations can resolve issues and make decisions faster, enhancing their agility and responsiveness.
Reference data management plays a pivotal role in dismantling data silos and centralizing data within a single, secure system.
How does reference data management work?
RDM can be effectively broken down into three main phases:
1. Data Collection and Integration:
- Purpose: This phase involves gathering reference data from various sources, which could include internal data repositories, external data providers, and third-party sources.
- Activities: It includes identifying relevant data, extracting it, and then integrating it into a centralized system. This process often involves data cleansing and normalization to ensure that the data is accurate and consistent.
- Challenges: Ensuring data quality and overcoming integration issues due to diverse data formats and standards.
2. Data Governance and Standardization:
- Purpose: Once the data is collected, it needs to be governed and standardized. This phase ensures that the data adheres to organizational policies and standards.
- Activities: It involves defining data standards, setting up governance policies, and implementing data quality rules. This phase also includes establishing roles and responsibilities for data stewardship and management.
- Challenges: Balancing flexibility and control, adapting to evolving data standards, and ensuring compliance with regulations and internal policies.
3. Data Distribution and Maintenance:
- Purpose: The final phase involves distributing the reference data to various systems and users within the organization and maintaining its accuracy over time.
- Activities: This includes setting up mechanisms for easy access to the reference data, ensuring data security, and continuously monitoring and updating the data as necessary.
- Challenges: Ensuring timely distribution of data updates, maintaining data security, and effectively handling changes in business requirements or data sources.
Throughout these phases, technology plays a key role. Modern RDM solutions often use databases, data warehousing technologies, and data integration tools to facilitate the process. Additionally, data quality tools and governance platforms are essential to maintain the integrity and usefulness of reference data.
Effective RDM leads to enhanced data consistency, improved decision-making capabilities, and better compliance with regulatory requirements. It's a foundational element for any organization that relies heavily on data for its operations and strategic initiatives.
Manage your data with RudderStack
RudderStack’s customer data platform (CDP) helps collect, clean, and consolidate customer data across an organization.
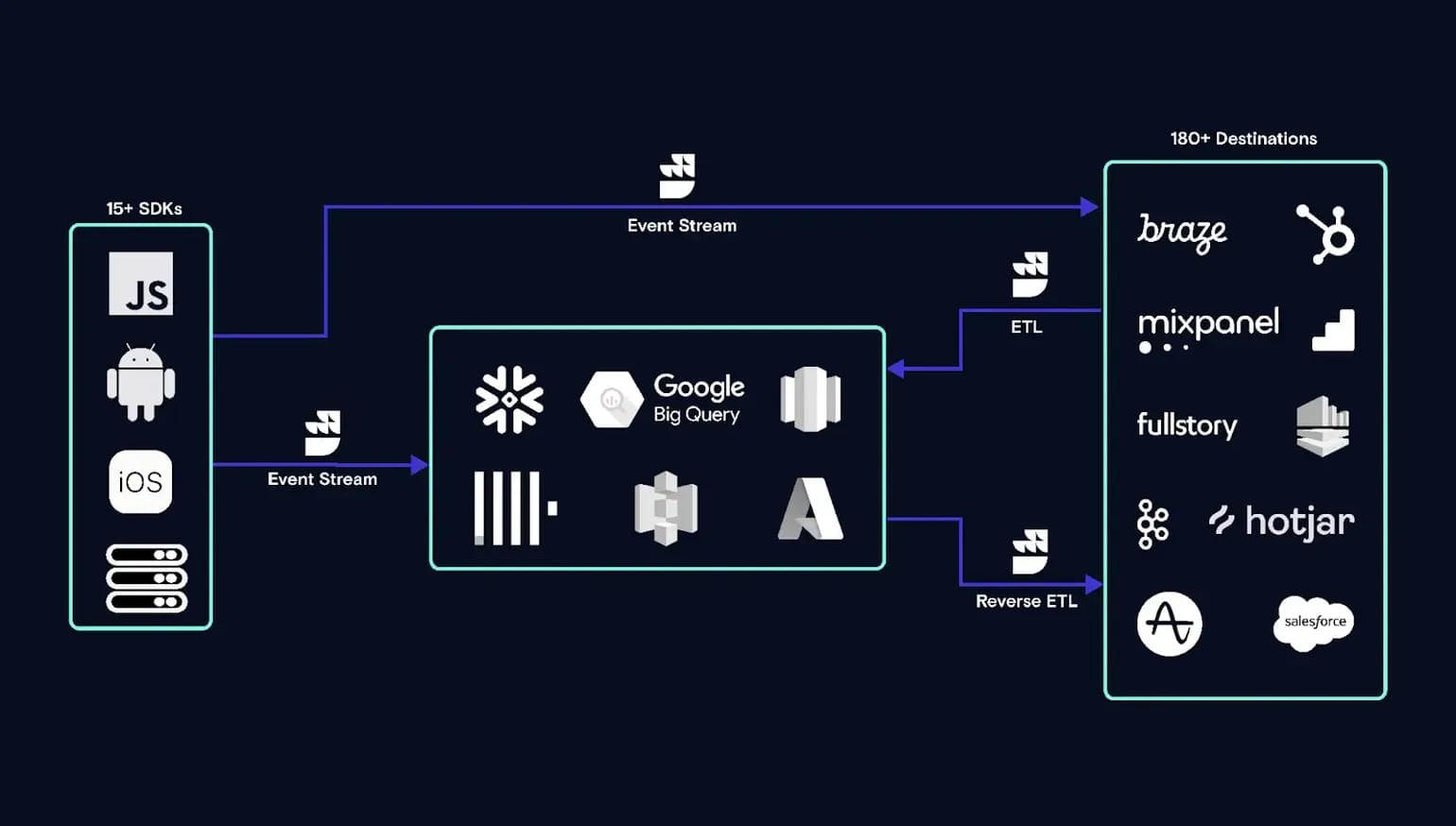
The Data Maturity Guide
Learn how to build on your existing tools and take the next step on your journey.
Build a data pipeline in less than 5 minutes
Create an accountSee RudderStack in action
Get a personalized demoCollaborate with our community of data engineers
Join Slack Community