What is Data Analytics?
Data analytics is the process of collecting, cleansing, transforming, and modeling data to discover useful and actionable insights to support business decision-making. In other words, data analytics helps you make sense of data so you can use it to improve your business.
In today's data-driven world, businesses of all sizes are turning to data analytics to gain a competitive edge. Companies use the findings from their data analytics teams to inform their decisions in areas such as marketing campaigns, product launches, and company logistics.
What is data analytics?
Data analytics is the science of systematically analyzing large raw data sets to draw conclusions. Data analytics in business involves answering ongoing specific questions about an organization using its past data. This includes real-time data as well as longer-term historical data.
The core of data analytics is data analysis (analyzing raw data to draw conclusions), but there are many other steps involved in analytics work. Collecting and preparing data, producing data visualizations, and communicating results to interested stakeholders are all primary components of data analytics.
Data analysts are skilled at interpreting data and looking for trends that help their stakeholders gain meaningful, actionable insights into their data. However, noticing patterns in existing data is only part of the meaning of data analytics — a talented data analyst will also look for anomalies in the data they have collected in order to identify gaps in their data collection methods which will help improve the analytics process. Most business questions are focused on the things that are not happening, so any unnecessary gaps in the data may lead to wasted work, as the wrong follow-up questions get asked. For example, if a data analytics report shows a 33% drop in website traffic one month, the business may commission another data analytics project to find out why. If the data analyst later discovers that their original data was only for the first twenty days of the month and that they are missing potentially one-third of their data, then the second project was a waste of time.
Understanding data analytics
The process of data analytics tends to follow the data analytics lifecycle, which includes generating a hypothesis, data cleaning, data analysis, building and running models, and communicating results to relevant stakeholders. Data analytics is particularly focused on creating ongoing reports and predictions. It does this by automating the process for consuming and monitoring data, so that the same questions can be answered on a regular basis, allowing a business to track how the answers to important questions are changing over time.
There are a number of different techniques that fall under the umbrella of data analytics, including but not limited to:
- Data mining: This is a technique for uncovering patterns and correlations in large data sets.
- Statistical analysis: Some basic forms of statistical analysis can be used to test hypotheses, while more complex forms may be used for building predictive models.
- Machine learning: This is often used in more advanced forms of data analytics and is usually used by data scientists. Machine learning involves developing algorithms that can automatically learn and improve from experience, and this technique is used to build complex prediction models.
- Data visualization: This technique allows us to view data in a visual form, such as charts and graphs. Data analysts use data visualization tools and coding libraries to produce visuals that are useful both for themselves and for stakeholders.
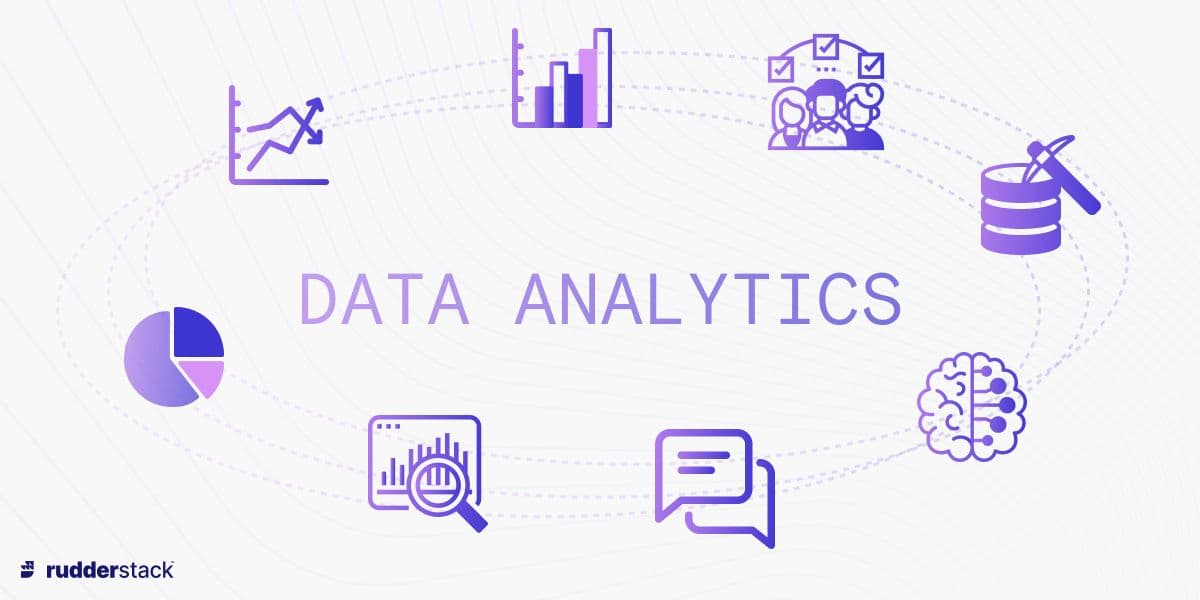
Types of data analytics
There are four primary types of data analytics: descriptive, diagnostic, predictive, and prescriptive. These often follow on from each other in the order “what, why, what next?” For example, it helps to know what happened (descriptive analytics) and why (diagnostic) before deciding what could (predictive) or should (prescriptive) happen next.
- Descriptive analytics focuses on understanding what has happened in the past.
- Diagnostic analytics delves deeper into why something happened by examining relationships between different factors. This type of analysis often relies on statistical methods like regression analysis.
- Predictive analytics uses historical data to make predictions about what is likely to happen in the future.
- Prescriptive analytics goes one step further by providing recommendations for what a business should do to achieve success in the future.
Predictive and prescriptive analytics often employ more complex statistical analysis and even sophisticated machine learning algorithms. Because of the extra complexity involved, these two types of analytics are normally performed by data scientists not data analysts.
The difference between data analytics and business intelligence
While there is some overlap between the two fields, there are also plenty of differences between data analytics and business intelligence. Both fields aim to answer business questions using data; however, business intelligence is more holistic and is focused on the strategic direction and the operations of an entire company, whereas data analytics answers more specific questions that might be related to one particular department. The questions that data analysts answer are often more mathematically complex than those in business intelligence, as data analysts tend to have more mathematical or statistical training.
Why is data analytics important?
Data analytics allows your company to make fast, well-informed business decisions, as well as to better understand your customers. Working out what your customers want allows you to improve your services or build new products with confidence that your customers will use them.
Understanding your customers better allows for many improvements within your company. It will help you streamline your marketing strategy, which will save you money. It can also enable you to correctly price your products or services, by working out what price potential customers are willing to pay - whereas business intelligence might tell you pricing based on costs and profitability - both are important but work in different specializations.
Finally, understanding how your customers have interacted with marketing campaigns can provide many useful insights, such as which campaigns drive traffic to your website or lead to more conversions. This knowledge can help you improve your return on ad spend or lower your customer acquisition cost.
Without data analytics, businesses would find it much harder to spot trends and patterns in large data sets. When data analysts spot interesting or unusual patterns in their data, this can lead to business insights that can help optimize ways of working. Data analytics has a variety of applications across different sectors and industries:
- Marketing: The analysis of a social media campaign could help a marketing team improve future marketing campaigns or gather more information about their audience.
- Sales: A sales team may use data analytics to predict future sales and behaviors. For example, a SaaS sales team might ask which parts of their online service their prospects are using during their trial phase (or, just as importantly, which features are not used!)
- Healthcare: In healthcare, data analytics can be used to improve patient outcomes by identifying risk factors and targeting interventions.
- Efficiency: Data analytics can be used to help manufacturers spot bottlenecks or inefficiencies in their processes, leading to process improvements in a company.
- Risk management: Analytics insights allow companies to spot inconsistencies in finances that could point to fraud or mismanagement. Data analytics can also help to develop a risk management strategy if emerging risk trends are spotted.
Data analytics improves your business decisions
Data analytics is a powerful tool that can be used to improve your business. By understanding the trends and patterns in your data, you can make better-informed decisions that will help you improve your bottom line. Data analytics can be used across many areas in your organization, including sales, marketing, finance, risk management, and process improvements. It can be used to support business decisions at all levels, from small operational decisions to large strategic ones.
All four types of data analytics (descriptive, diagnostic, predictive, and prescriptive) can be useful, but prescriptive analytics is the most comprehensive form of data analytics. It is often seen as the capstone of a business’s data strategy and data maturity since it requires the previous three to be well established and working in order to be leveraged correctly. This is because it can provide suggestions on what a team or company should actually do, which is ultimately the most important question that data analytics can answer. With the other types of analytics, some information is provided, but a skilled person is also required to work out what the company should do based on that data.
The Data Maturity Guide
Learn how to build on your existing tools and take the next step on your journey.
Build a data pipeline in less than 5 minutes
Create an accountSee RudderStack in action
Get a personalized demoCollaborate with our community of data engineers
Join Slack Community