What is Diagnostic Analytics?
Diagnostic analytics is one of many different types of analytics that you can perform to glean insights from your data. Diagnostic analytics can inform marketing campaigns, updates to products and services, and ways to make internal processes more efficient.
This page gives an overview of diagnostic analytics — what it is, how to use it in your business to make better data-driven decisions, its benefits and limitations, and examples of the types of questions that diagnostic analytics aims to answer.
What is diagnostic analytics?
Diagnostic analytics is the area of data analytics that is concerned with identifying the root cause of problems or issues. Unlike other types of data analytics, which focus on understanding what has happened in the past or on predicting what will happen in the future, diagnostic analytics is focused on understanding why something has happened. Being able to give a concrete “why” behind users’ actions or lack thereof is one of the hardest tasks in data analytics as it is a process of inference, not proof.
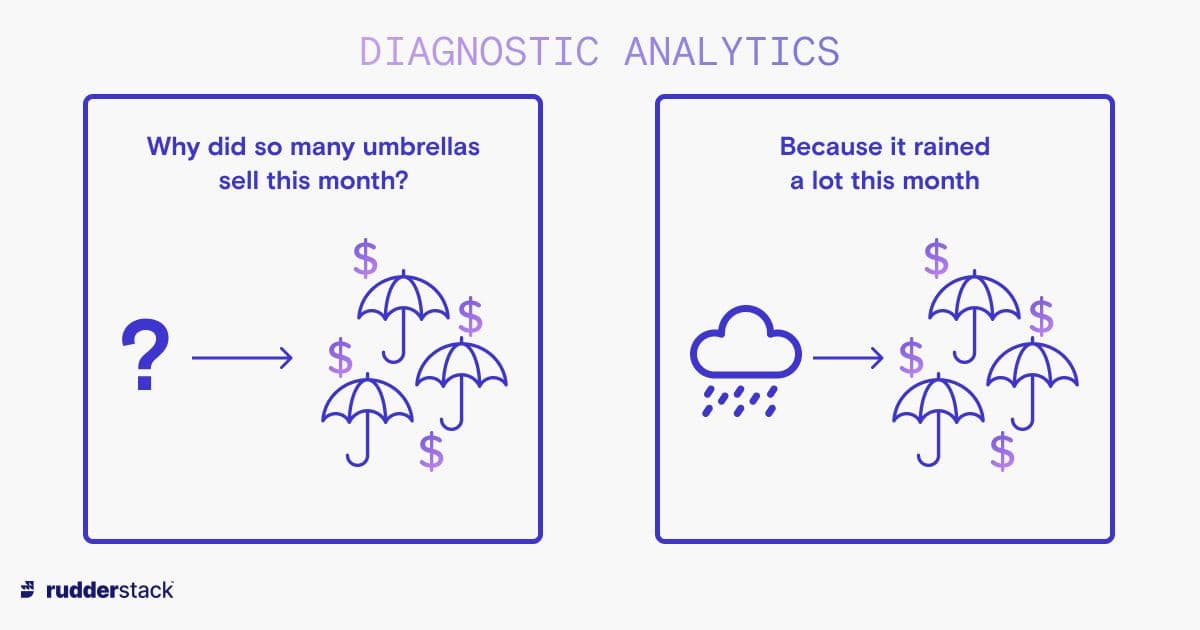
It often follows descriptive analytics, which focuses on what has happened in the past. Diagnostic analytics relies on information from descriptive analytics to proceed, as you need to know what happened before you can ask why it happened. This is followed in turn by prescriptive analytics, which focuses on what to do in the future. Following the order of “what?” then “why” then “what next?” is a sensible way to do data analytics, as you need to know what happened and why before you can decide what to do next.
One example of diagnostic analytics is a marketing funnel analysis. This means looking at the set of steps that a user might take before reaching a final goal, such as a conversion or a sale, and understanding why they do or don’t complete each step. For example, before a user reaches the goal of a purchase, they may reach a series of intermediate goals such as visiting your website, adding an item to their shopping cart, and clicking the “checkout” button. Diagnostic analytics allows you to analyze why people are not converting or purchasing by looking at which steps they were at when they dropped off, and inferring why.
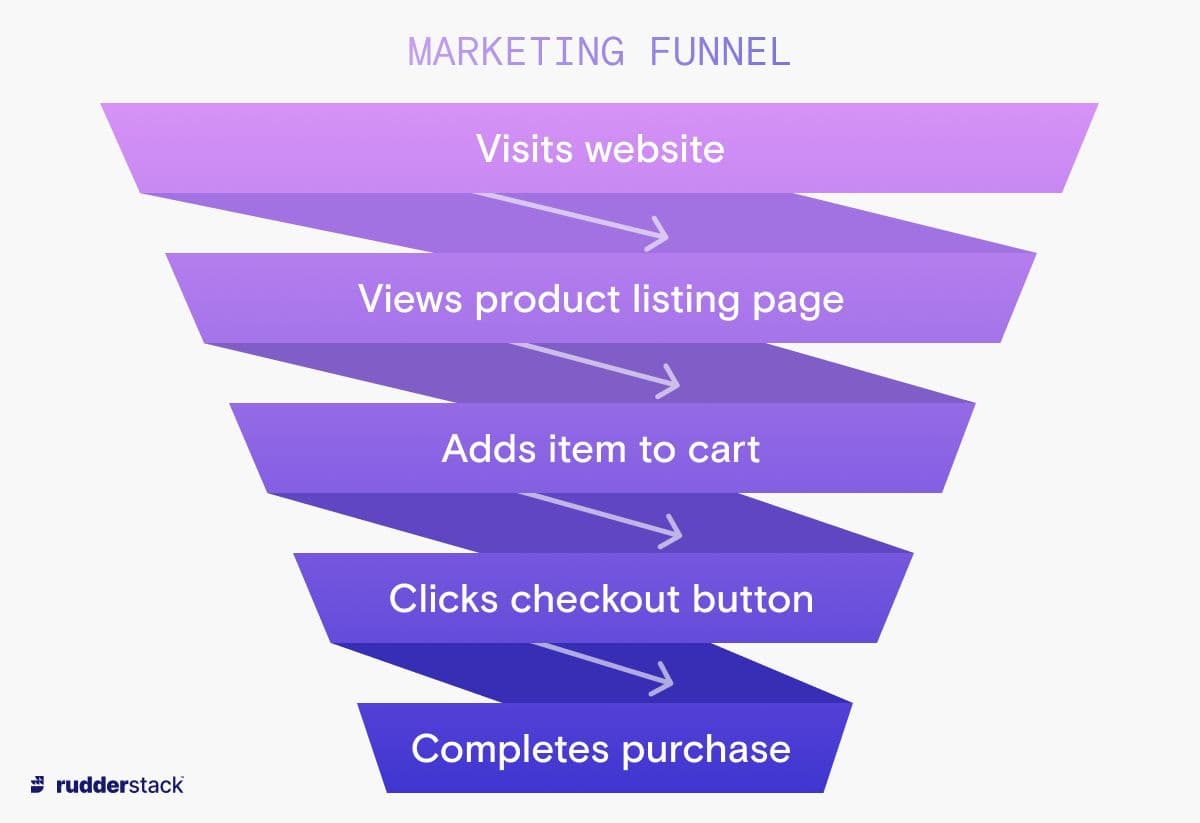
Benefits of diagnostic analytics
The purpose of diagnostic analytics is to give a business more actionable information than descriptive analytics alone. It enables you to find out why something is working (or not working), allowing you to correct any wrong assumptions you may have had. The benefits of diagnostic analytics include:
- Greater insights: It allows for deeper insights into your data when used in conjunction with other types of data analytics.
- Forming and testing hypotheses: Having evidence of what has previously happened helps businesses to form and test new hypotheses more easily.
- Identifying anomalies: Diagnostic analytics helps you determine whether data outliers were one-off anomalies or useful, significant findings.
- Avoiding future mistakes: It helps you identify when and where something didn’t perform well, enabling you to improve efficiency, reduce waste, and avoid costly mistakes.
- Ease of understanding: Diagnostic findings are generally simple to understand, and once they have been turned into data visualizations, they can be easily shared with stakeholders.
Limitations of diagnostic analytics
One limitation of diagnostic analytics is that it is easy to mistake correlation for causation. For example, there is a correlation between ice cream sales and bee stings, but that doesn’t mean that one caused the other. They are in fact both dependent on a third factor (warm temperatures). So any correlations in your data must always be fully investigated before assuming a causal link.
Diagnostic analytics can’t predict the future, or make suggestions about what should be done — it can only explain why something happened, and any further information can only be gained either from a knowledgeable person making educated guesses or from predictive or prescriptive analytics. Nor does it answer the question “What should we do?” — this is answered by the field of prescriptive analytics.
Diagnostic analytics doesn’t give definitive answers. It can’t tell you that A definitely caused B, only that a certain percentage of people who encountered event A did (or did not) encounter event B. The accuracy of outcomes can be improved, however, with better-quality data, larger data sets, and the involvement of domain experts in interpreting the data.
How to use diagnostic analytics in your business
The first step in diagnostic analytics is deciding on the questions you want answers to. These may include questions like:
- "What causes customers to cancel their subscriptions to our online product?"
- "Why has web traffic decreased by so much this month?"
- "Why are so many of our employees quitting their jobs this year?"
- "Why do sales always increase in November?"
You should ensure that you have access to a reasonably large data set containing good-quality data that’s relevant to your question. This will help you to draw useful inferences and avoid making decisions based on outliers or the opinions of a vocal minority. Some examples of the kinds of data sets that are large enough to be useful are sales records, marketing statistics, and product inventory records. Once you have some suitable and relevant data, you can develop your hypothesis — your proposed reason for why the thing you are studying happened — to help direct your analytics. Even though diagnostic analytics doesn’t deal with future predictions, it’s still possible to have a hypothesis about the past. For example, you might hypothesize that the reason sales fell last month was because you spent less on advertising.
Next, you will need to prepare your data by cleaning it (which may involve removing defective data or duplicates), transforming it into a useful format, and loading it into a single location such as a data warehouse. You can also filter the data so that only what is relevant is left for the analysis, or do data drilling, which involves looking at hierarchical data at a higher or lower level — so “drilling down” is when you access data at a deeper, more granular level than before. For example, when looking at how potential customers have responded to a particular marketing campaign, you might drill down to see how those who live in a particular region responded. Once your data is prepared, you can use one of the diagnostic analytics techniques below.
Finally, you will need to create some data visualizations to use when communicating your results to any interested stakeholders. If your analytics need to be run regularly, you should automate the above steps and run it regularly against your production data, which is known as operationalizing your analytics. As you formalize your diagnostic analytics steps, it will be useful to refer to the data analytics lifecycle, which covers all the necessary steps including operationalizing your analytics.
Once you are comfortable posing questions, forming hypotheses, and using your data to support or disprove them, you can get creative. Less-proven data sets, or data from third parties, can be introduced to see if they can yield any additional depth or experimental insights from your diagnostic analytics process.
Diagnostic analytics techniques
Different diagnostic analytics techniques can be appropriate depending on the type of question you are trying to answer and how comprehensive your data is. These techniques tend to involve either statistical analysis or machine learning.
Statistical analysis
Statistical analysis is the process of finding trends and patterns in data through the use of statistical models. These models are also used to help work out which relationships between variables are the most important. Some common statistical models for diagnostic analytics are:
- Correlation analysis: finding out if there is a relationship between two or more specified variables or data sets and investigating how strong that relationship is.
- Regression analysis: working out which variables affect the thing you’re interested in the most (for example, sales).
Machine learning
Machine learning algorithms can also be used in diagnostic analytics, for example:
- Binary classification: making a decision about something that only has two possible answers. The answers to questions like “is the weather affecting sales or not?” and “do customers like our new product or not?” can only be yes or no.
- Time-series analysis: looking at trends (or data) that change over time. This could be to answer a question such as why sales decreased in the previous quarter.
While machine learning techniques are useful, humans with domain-specific knowledge are still needed to provide context to the outcomes of diagnostic analytics. For example, an expert might realize that a credit card customer making regular withdrawals of a similar amount suggests that they may be using one credit card to pay down another, and are thus a risk, whereas a machine might not have the context to be able to understand what this unusual pattern means.
Diagnostic analytics examples
The following examples show how different departments might use diagnostic analytics to make improvements to their business by developing a better understanding of why things happened in the past.
Sales: A sales team might use diagnostic analytics to understand why profits are dropping (or increasing), or to identify sales trends based on environmental factors — “Is it our new seasonal sale that is driving sales, or did a competitor increase their pricing?”
Marketing: A marketing team might perform diagnostic analytics to find out why a campaign, website, or social media post saw an engagement increase, or why their company is experiencing a declining click-through rate.
IT: Diagnostic analytics can be used to work out what problems are happening in a company's IT infrastructure — “Why does a certain module take a long time to run? Is the database a bottleneck, is the application code waiting for an external API, or is the application server itself bottlenecked?”
Product: A product team needs to know why people aren’t using a particular feature of their product (or why they are).
Customer support: Diagnostic analytics allows this team to understand why customers are creating so many support tickets for a particular feature — “Is it a bug in the application or are users having UX problems and struggling to use the feature?”
Diagnostic analytics and customer data platforms
Diagnostic analytics helps address the question of why something happened by analyzing data. It also helps to spot trends and explain customer behavior. Using diagnostic analytics can inform a company's future decisions, based on hard evidence, to improve business performance and increase sales.
A customer data platform (CDP) provides an easy way to prepare your data by facilitating data collection, getting it all into one place, then cleaning and transforming it until it is ready for analysis. When choosing a CDP, make sure that it can handle the number of events that you need to process, and that it takes data security seriously. Any CDP supplier should be certified as being compliant with a cybersecurity framework such as SOC 2 or ISO 27001.
The Data Maturity Guide
Learn how to build on your existing tools and take the next step on your journey.
Build a data pipeline in less than 5 minutes
Create an accountSee RudderStack in action
Get a personalized demoCollaborate with our community of data engineers
Join Slack Community