What is Data Integrity?
Remember playing "Chinese whispers" as a kid? Someone would start by whispering a phrase like "sunny day" to the next person, who would then pass it on. By the end of the line, the message might have morphed into something completely different, like "funny play." This illustrates how easily information can become distorted; know as loss of data integrity in the data world.
Now, imagine this game being played on a massive, global scale, with the stakes being much higher. Cisco's annual internet report suggests that by 2023, the number of networked devices could exceed three times the world's population. With such an enormous amount of data being transmitted and processed, maintaining its accuracy and integrity becomes a formidable challenge.
This is where the concept of big data comes into play. Big data isn't just about how much data we collect, but also how we use, protect, and share it. For businesses, researchers, governments, and individuals, managing big data effectively is crucial for fostering growth, innovation, and trust. Keeping data intact and reliable is essential in this rapidly expanding digital landscape.
What is Data Integrity?
Data integrity refers to the ongoing effort of a business to collect and preserve data that is accurate and consistent across various sources, teams, and formats. When conducting a data integrity audit, several key questions are posed: Is the data precise and unaltered? Has it been damaged or unintentionally lost? Is it modified in any form? Are the data sets complete and devoid of repetitions? These factors are crucial in determining the integrity of a data set, or the lack thereof.
To maintain data integrity, companies employ various methods such as quality control measures, integrating data from multiple sources, validating data accuracy, removing duplicate entries (de-duping), ensuring proper preservation and storage, managing privileged access, implementing robust cybersecurity measures, and conducting regular audits.
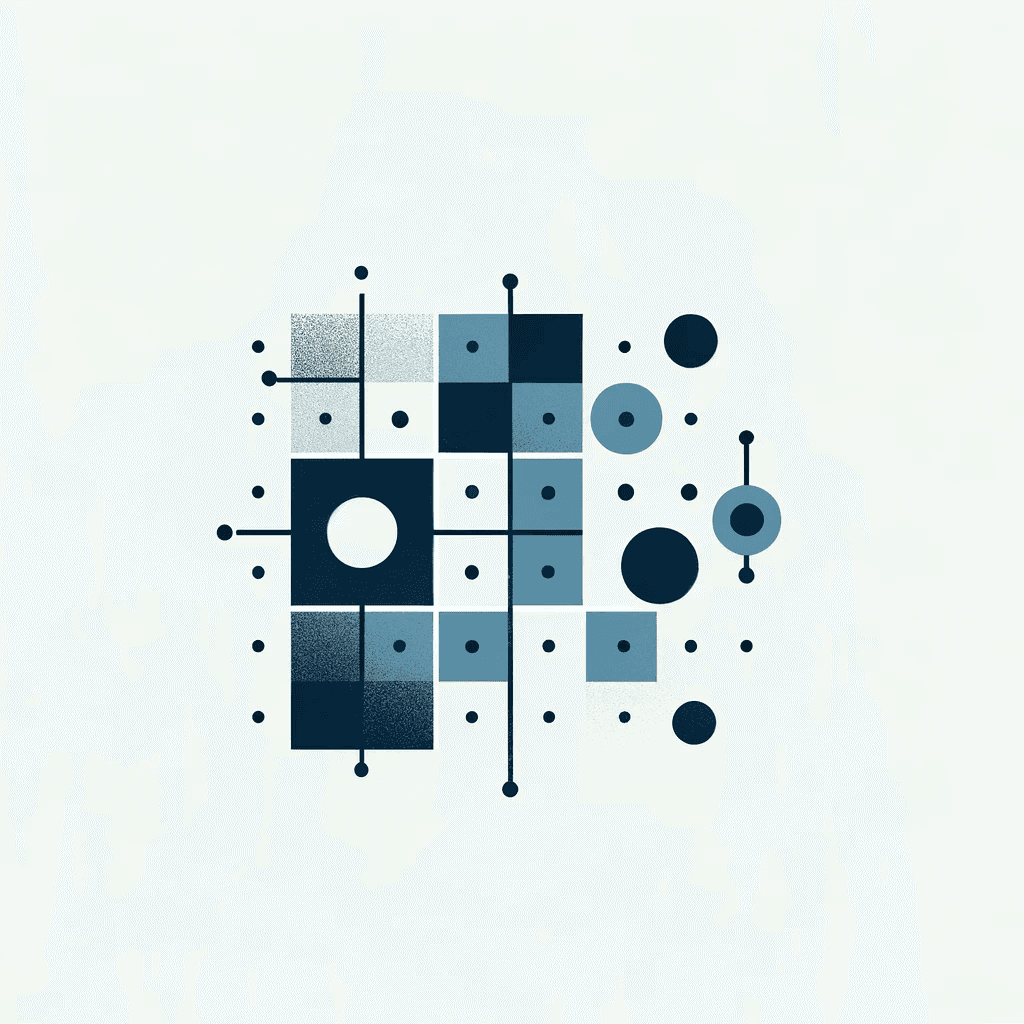
What are Data Integrity Threats?
Data integrity faces numerous threats, each with its unique challenges and impacts. These threats can significantly compromise the reliability and accuracy of data. They include:
1. Data Loss or Deletion: This happens when unauthorized access, often by hackers, leads to the deliberate destruction or alteration of data. For instance, a cyberattack could result in the loss or corruption of critical company data.
2. Transfer Errors: Occur during the movement of data between platforms or systems, such as errors in cell referencing when shifting data from Microsoft Excel to Google Sheets. These errors can lead to inconsistencies and inaccuracies.
3. Data Entry Errors: Simple human errors in data entry, like transposing numbers or misspelling words, can lead to significant inaccuracies in data records.
4. Collection Errors: These arise when the methods or tools used to gather data are flawed. An example is survey data that fails to accurately represent the target demographic, leading to skewed results.
5. Commission of Inaccurate Data: This involves deliberately creating or adding false or misleading data into the system, which can skew analysis and decision-making.
6. Manipulation of Existing Data: Altering or tampering with existing data, which can be done with malicious intent or through careless handling, affects the data's authenticity.
7. Corrupted Data: Data can become corrupted due to technical issues such as server power outages or software malfunctions. This corruption can render the data unusable or misleading.
8. Duplicate Data: Creating multiple records for the same entity, like having several customer profiles for one individual, can lead to confusion and inefficiency in data management.
9. Aging Data: Over time, information can become outdated or irrelevant, but if not updated or archived properly, it can lead to incorrect conclusions.
10. Unauthorized Access: Data integrity is compromised when individuals without proper clearance or malicious intent gain access to sensitive data.
11. Inadequate Data Backup: Failing to back up data properly can result in permanent data loss in case of a system failure or other disaster.
12. Software Bugs: Errors in software can lead to unexpected data alterations or loss, impacting the data's reliability.
Each of these threats requires specific strategies and tools to mitigate, such as robust cybersecurity measures, regular audits, thorough training in data handling, and the implementation of reliable backup and recovery systems.
Data Integrity VS Data Security
Data integrity and data security, while interconnected, address different aspects of data management. Data integrity is concerned with ensuring the accuracy, consistency, and reliability of data throughout its lifecycle. It involves processes like validation, duplication checks, error correction, and maintaining consistency in data format and structure. On the other hand, data security focuses on protecting data from unauthorized access, breaches, and other forms of cyber threats. It encompasses measures like encryption, access control, and protection against malware. While data security is about safeguarding data from external threats and unauthorized access, data integrity ensures the data remains correct, unaltered, and reliable for its intended use. Both are critical in the digital age for maintaining trustworthiness and usefulness of data, but they operate in different, though complementary, spheres.
Data integrity encompasses a broader scope. Indeed, ensuring data integrity involves not only security measures but also a variety of other practices.
These practices include automation, which helps reduce human error; error-checking protocols to ensure accuracy; physical integrity, addressing the physical storage and handling of data; logical integrity, ensuring data is consistently structured and accessible; and domain integrity, maintaining the validity of data within its expected range or format.
Additionally, access controls are crucial in restricting data access to authorized users; referential integrity ensures that relationships between data in a database remain consistent; and entity integrity guarantees the uniqueness and completeness of data records. All these elements are integral to maintaining the fidelity and accuracy of data.
Why data integrity is critical for business success
Businesses often underestimate the importance of data integrity. Yet, it’s a vital principle of the GDPR guidelines. Getting it wrong costs serious money in product development, marketing, personnel, and even possible fines or lawsuits. To get it right, you need to cultivate data accuracy, control accessibility, and achieve full integration.
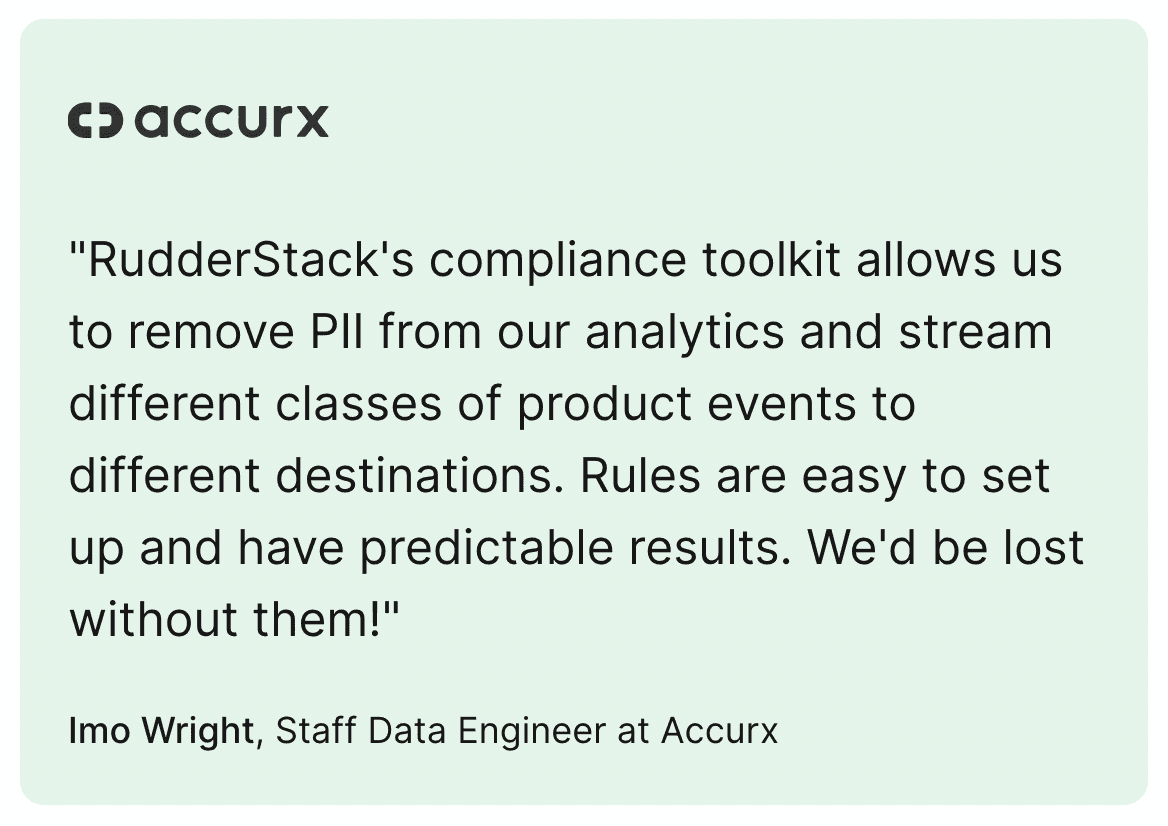
How Accurx Built a Scalable, GDPR and HIPAA-compliant Stack With RudderStack
‎Learn how RudderStack enabled Accurx to quickly move from a limited technology stack to a powerful, compliant Warehouse Native CDP that quickly increased product insights and engagement.
1. The Importance of Accurate Data for Trust
Reflect on the game of Chinese whispers. Imagine this in the context of your customer data being distorted. The incorrect data might indicate that most customers prefer apples for breakfast, while the reality is they prefer bananas. Such inaccuracies can lead to immediate consequences, like your product not being purchased, and long-term repercussions, such as diminished trust in your brand. Misleading data can result in financial waste on undesired products and a loss of customers and investors, who are reluctant to engage with a company known for its inaccuracies.
2. Data Access Management for Legal Compliance
Implementing robust data integrity policies involves regulating data access, ensuring everyone has exactly what they need - no more, no less. Managing access provides two key benefits. Firstly, it enhances efficiency as different teams can access and utilize data coherently. This controlled access not only safeguards your assets but also enables staff to perform their duties effectively according to their roles. Secondly, and perhaps more crucially, it guarantees that only authorized individuals can view or modify data. This heightened security aligns with legal standards like GDPR, offering more stringent data protection.
3. Gaining Insights from Integrated Data
Integration is essential for data consistency and completeness. If your business gathers customer data through various channels - such as mobile, phone, social media, and in-person interactions - integrating this data is crucial for comprehensive decision-making. By consolidating all this information into a single, extensive data lake and integrating it with your technological infrastructure, you unlock the potential for more advanced insights about your customers. This holistic view of customer interactions across all platforms enables more informed and strategic business decisions.
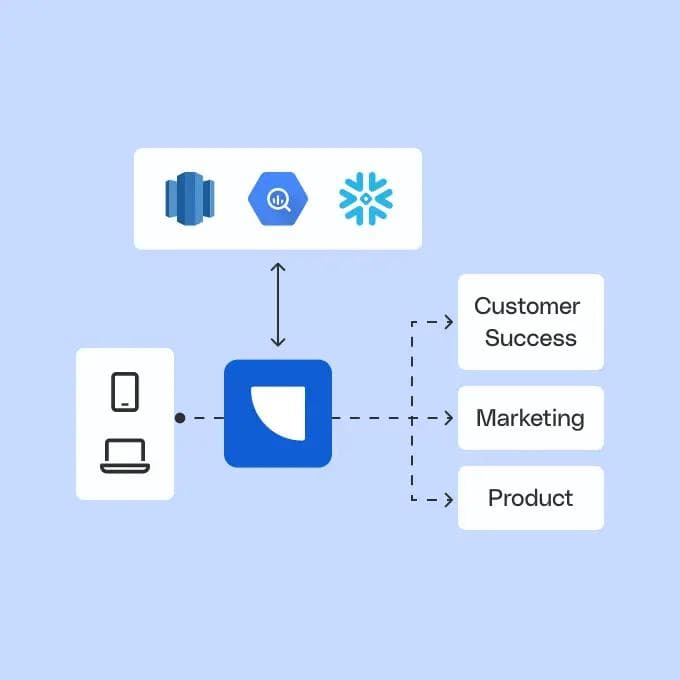
How to Ensure Data Integrity
Maintaining data integrity requires deliberate effort and ongoing investment, both in terms of time and resources, but the long-term benefits are substantial.
1. Uniform Data Collection and Pre-Storage Cleaning
Standardizing data collection involves gathering data in a consistent format. Begin by identifying your data sources and establishing uniform standards for aspects like spelling, capitalization, and spacing. For instance, if you're collecting customer addresses, decide on a consistent format (e.g., always using “Ave” instead of variations like “ave,” “Ave.,” or “avenue”). Automating this process can save time and reduce errors, and tools like Segment’s Protocols can be particularly useful for this task.
2. Prompt Validation and Resolution of Data Quality Issues
Validating data entails checking the accuracy of the source data to prevent errors before the data is used or integrated elsewhere. This includes correcting common errors in naming conventions, typos, or capitalization. Automated validation reports, such as those provided by Segment’s Protocols, can alert engineers to discrepancies, allowing for quicker correction without the need to manually scrutinize each data entry.
3. Preventing Bad Data at the Source
It’s more efficient to prevent data errors than to fix them afterwards. Implement measures to block incorrect data right at its source, thus avoiding contamination of your data warehouses or marketing tools. For example, using a system like Protocols, any data that doesn't align with your Tracking Plan can be isolated in a separate warehouse. This allows you to identify tracking issues and decide whether to keep or discard non-standard data, ensuring that only quality data is used in your operations.
The Data Maturity Guide
Learn how to build on your existing tools and take the next step on your journey.
Build a data pipeline in less than 5 minutes
Create an accountSee RudderStack in action
Get a personalized demoCollaborate with our community of data engineers
Join Slack Community