The Modern Data Stack is Warehouse-first
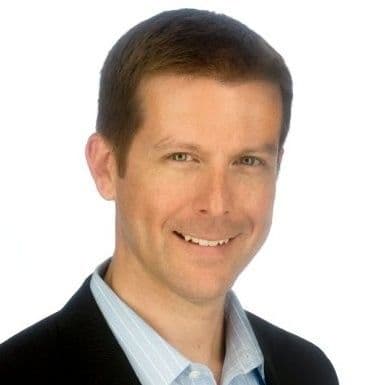
Ryan Koonce
CEO, Mammoth Growth
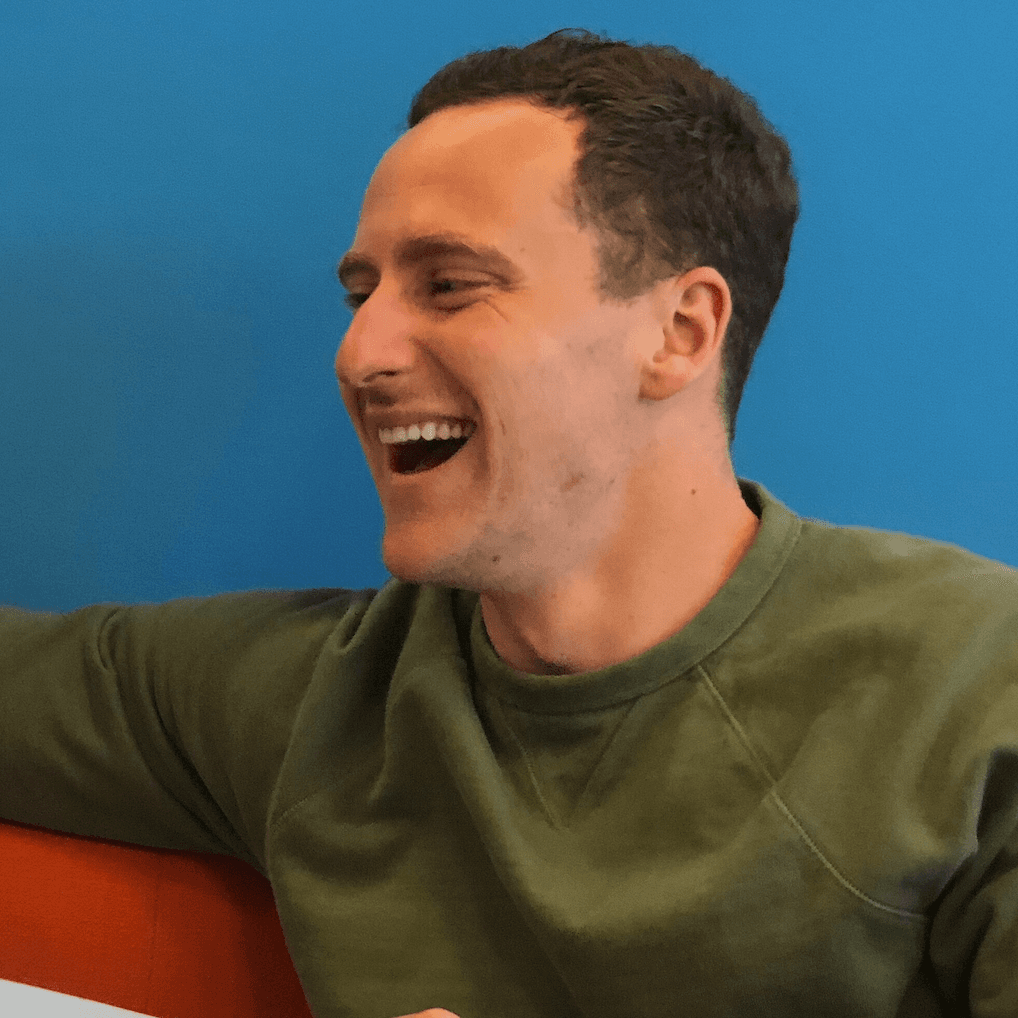
Benjamin Gotfredson
Global Startup Program Manager at Snowflake
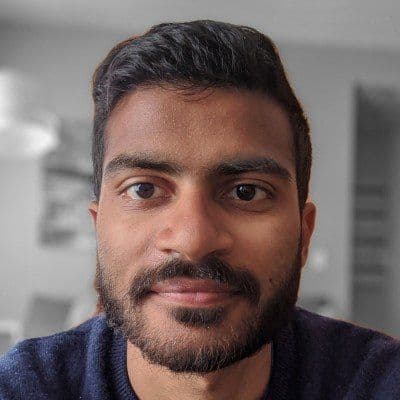
Vijay Iyengar
Director of Product at Mixpanel
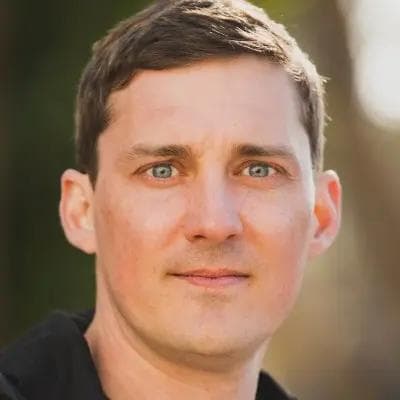
Eric Dodds
Senior Director of Product Strategy
Advancements in data tooling are enabling data-driven companies to become drastically more sophisticated, and they’re making data more accessible to everyone.
In this live panel discussion, we explore how building a modern data stack around your cloud data platform unlocks your data’s full potential. You’ll hear from leaders at Snowflake, Mixpanel, Mammoth Growth, and RudderStack.
- Defining the modern data stack
- Why the cloud data platform belongs at the center
- Breaking down data silos
- Moving data across the stack
- Delivering deeper insights
- Fueling growth
Ryan Koonce (00:00)
All right, without further ado, thanks everybody for coming today. My name is Ryan Koonce. I'm the founder and CEO of Mammoth Growth, and we are going to talk about The Warehouse-First Modern Data Stack. And with us today, we have Ben Gotfredson, he's the Global Startup Program Manager at Snowflake. We've also got Vijay Iyengar, Director of Product at Mixpanel, and Eric Dodds from Growth at RudderStack. And so without further ado, we'd like to jump right in.
Ryan Koonce (00:33)
And in talking about the Warehouse as sort of the center of the Modern Data Stack, I want Ben, just sort of highlight Snowflake and where we've come from and to, and why Snowflake really matters. I mean, if you go back to when I started my career, we were talking about Hive and Hadoop, and then it was sort of MongoDB, and then it was Redshift and now we have Snowflake. And at Mammoth, we are huge Snowflake advocates and there's a whole bunch of reasons why. If we have a choice, we'll pick Snowflake as sort of our data warehouse. If you could, I'd love you to chime in and just sort of give everybody a little bit of a heads up on why Snowflake is what it is and why there's so much excitement around it.
Ben Gotfredson (01:24)
Yeah. Just quickly, my name is Ben Gotfredson. I've been with Snowflake for about seven years. So it's really gone from a startup to the enterprise that it is in that time. And in my role, I support startups that are kind of going through a similar growth phase and just enable them and develop a strategy behind their success that they're going to have on Snowflake. So a lot of these concepts are front of mind in every conversation I'm having with either early adopter new Snowflake users that aren't going to be very technical or really data-driven startups that are going to be using Snowflake in a pretty complex way.
Ben Gotfredson (02:05)
And I think the first point I'd like to really address is what are the type of technology shifts that are driving the change that we're seeing, right? Especially in those seven years that I just mentioned, right? So from 2014 to 2015 to now, what change enable more and more companies to have this kind of approach? Identified a few, I think are worth sharing. So on one point, it's just the capacity that the public cloud players are providing all of us, right, from AWS to Google, to Microsoft, just the ability to have this unlimited capacity of compute and storage at your fingertips is kind of changing the game.
Ben Gotfredson (02:49)
I think the second point that all of our products and companies had to touch on is simplified tools, right? You don't need the same complex skills that you needed to get Hadoop cluster off the ground in 2013, 2014. You know SQL, you can kind of get the ball rolling and all the tools that are surrounding the data Cloud, the data platform, the data warehouse are also coming at a very simplified approach for that. A few more, I think the data types that we're analyzing today are new, right? It's not just structured data sets we're able to get into a warehouse. We're seeing way more semi-structured data and more recently unstructured data being used. [crosstalk 00:03:31].
Ryan Koonce (03:31)
Hey Ben, can I interject real quick there?
Ben Gotfredson (03:34)
Yeah.
Ryan Koonce (03:34)
I think, I mean, just to sort of jump in on that, when you talk about, just for the audience, structured data and unstructured data, maybe you could define a little bit what those sources look like and how Snowflake is able to benefit them in a way that maybe might have been more difficult before. Because I think sometimes, we talk to clients that are a little bit more nebulous.
Ben Gotfredson (03:53)
Yeah. It's a good point. I mean, I think the biggest one that we see is semi-structured data, which definitely has been for the last five, six years. So you think of IoT being generated typically in the form of JSON can be coming from any different suite of different sources but the one common link is that it tends to come in a massive amount and previously you were having to transform that semi-structured data at IoT data into a structured format before you can start getting any insights and pull any data from it. So maybe that's something you've experienced firsthand, but cutting that step out means an easier pipeline to manage but also you're getting the data in front of yourself quicker in your team.
Ryan Koonce (04:37)
Yeah, definitely. And we talk about two things, one thing you said was you get a lot of stuff with Snowflake for free and what we mean by that is all the DevOps that goes around, standing it up and making it work, and then I think the second thing that might be a misconception with a lot of companies is this idea that it's going to be really expensive. And, to be honest, for that datastore and because we have all these cloud providers at our fingertips today, it's usually not the major constraint, I mean those conversations, for example, that massive IOT feed you were talking about.
Ben Gotfredson (05:13)
Yeah. Agreed. That kind of ties into another shift, right? Which is just the usage-based model being deployed instead of having to plan one, three years ahead and buy upfront, just being able to actually spend on what you end up using on the warehouse takes some knowledge on how to actually use a tool like Snowflake and what the costs around it are, but ultimately it does tend to drive to more price effective solution. And then I guess the last point I'd make on the technology shift is really like the surrounding ecosystem. Right. I remember six, seven years ago, a lot of the companies we were meeting with were having to build their own ETL processes, build their own ETL tools, and with all the simplicity that's come on the front end, the ingestion, and also the visualization, it's allowed for a lot of progression on the data warehousing side too, I'd say.
Ryan Koonce (06:03)
Yeah, definitely. And with that in mind, I think, one of the things that we really focus on at Mammoth is the ability to have that connectivity into stuff like, and actually for us, the faster we can get to insights the better. And with that, Eric, I'd love you to jump in and introduce yourself and maybe talk a little bit about moving data into Snowflake and how that data collection identity resolution is relevant.
Eric Dodds (06:29)
Yeah, absolutely. I'm Eric and I work on the growth team here at RudderStack and part of my job is actually managing our implementation of RudderStack and Snowflake and in fact Mixpanel. So we actually do this stuff for ourselves every single day. So it's kind of fun because we get to experience a lot of the same things our clients experience. I think Snowflake is such a powerful tool and I think one of the challenges that a lot of companies face is managing all of the various pipelines required to get the data into Snowflake.
Eric Dodds (07:09)
Snowflake comes with a lot of great stuff out of the box but especially when you think about things like real-time streaming data and dumping that into the warehouse to feed all sorts of interesting analytics use cases, machine learning models, other sort of interesting ML things you're doing on the warehouse. A lot of times the dynamic we see is that because every business has pretty unique needs, especially as they grow in scale, you start to spend a lot of time just engineering the plumbing as opposed to actually building a data product on Snowflake. Right. And so at RudderStack, we want to make it super easy to solve the plumbing problem. Right. You don't have to-
Ryan Koonce (07:54)
Eric, maybe take a real quick step back and talk a little bit about RudderStack and sort of what it does and where it fits in, just for people that maybe aren't quite as familiar here.
Eric Dodds (08:04)
Yeah, absolutely. Thanks for giving the breaks there for me, Ryan. RudderStack is a customer data platform focused on developers. So we provide you pipelines that make it easy to move customer data anywhere in your stack. So real-time streaming pipelines, you can think about behavioral data from websites and apps, also ETL pipelines. It sort of takes your traditional structured cloud data and dumps it into the warehouse and then also reverse ETL. So something we're seeing more and more is companies that want to do some sort of analysis inside of the warehouse, like Snowflake, and then push that value back out to other tools in the stack. So we are your place to come to move that customer data anywhere in the stack.
Ryan Koonce (08:51)
All right, great. Sorry, I didn't mean to interrupt you.
Eric Dodds (08:53)
No, it's great.
Ryan Koonce (08:55)
The connection points between the ETL in the Snowflake and what you guys do, I just wanted to back up a little bit and make sure everybody knew what those pieces were.
Eric Dodds (09:05)
Yeah, totally. And to get to your question, so moving data across the stack, so we make it really easy to move data across the stack. Snowflake is a primary destination for a lot of RudderStack customers. And one thing we see, you mentioned identity resolution, so one thing we see that's actually pretty hard, especially when you think about spending a bunch of engineering hours just on moving the data to different places in your stack, is a lot of times that's literally just the movement from point A to point B and every company is somewhere on the journey of trying to build Customer 360 or single paint of glass, whatever unified profile and the place to do that really is the warehouse. But in order to do that, you actually need some sort of way to reconcile identities, especially when you think about going cross-platform.
Eric Dodds (09:57)
So one of the things we see companies using RudderStack and Snowflake for is that RudderStack solves a lot of the identity issues on ingest into Snowflake so that it's really easy to make the joints that you need to join to actually build that unified customer profile. So on the incoming data, you can tie all the pieces together, whether that's from a mobile platform website, structured cloud data, you can sort of solve the identity resolution problem. Again, just trying to make it easier for someone to actually do a limited number of joins to get that unified profile within Snowflake.
Ryan Koonce (10:36)
That's great. And then I want to bring Vijay back into this and maybe you can introduce yourself real quick and we can talk about how Mixpanel fits into this puzzle as well.
Vijay Iyengar (10:46)
Yeah. Sounds great. Hi, everyone. I'm Vijay, Director of Product here at Mixpanel, been with Mixpanel for about five years in various engineering and product roles. And so Mixpanel's actually been around since, before I guess the evolution of this modern data stack and was hence historically, primarily been focused on engineering and product teams getting really fast self-serve exploratory answers from their product telemetry, like users using their app and turning that into insights, like, what actions do users take that lead them to retain long term or what kind of drives conversion in these core funnels that are relevant to your business, or even just seeing kind of active use or growth over time. And what we've really seen with the emergence of warehouses like Snowflake and this broader and modern data stack, is that it's been a really great tailwind for us for getting richer, trusted, well-governed data into Mixpanel. So that Mixpanel's not kind of just this silo that has your raw event data, but also has kind of this core transactional events that are coming in from systems of record into your data warehouse so that you can kind of do funnels on these really core, transactional events that are relevant to your business.
Vijay Iyengar (11:52)
And second, being able to enrich the events in Mixpanel with kind of what Eric was saying, the 360-degree view of your customer or your accounts for B2B use cases, bringing that data in so that you can see, for example, not just how many unique users do I have using this particular feature, but what percentage of my revenue is associated with people using this feature or in which geographies or which customer success manager should I talk to for users who are power users of something that's in my product. So really being able to marry the kind of product telemetry with this single unified profile of users and accounts is what we're seeing is the benefit of using Mixpanel alongside the modern data stack.
Ryan Koonce (12:34)
Yeah, and from our view at Mammoth, we have a lot of people that show up and say, I need a warehouse and I need to visualize the data. And for us, it's really about getting to the answers to the business questions in the most cost-effective way. So for example, you want to have all of that data that might come in from a separate source into Snowflake in Mixpanel, and you're not going to build a funnel report in Tableau or Looker. I mean, you can, but it'll cost you a fortune, right?
Ryan Koonce (13:02)
And so the thing that we sort of focus on, which I think is really important and relevant to the conversation is this bi-directional sync that exists today, where we can get source data that's maybe not customary, or maybe not something that you would see natively in Mixpanel traditionally. We have the ability to now get it up into Mixpanel and run those reports that would've otherwise in the past been, to be frank, fairly difficult. And so Eric, it'd be helpful if you could talk a little bit about this idea of data in, data out and how it relates to what we can do at Mixpanel today with regards to sort of what ETL reverse ETL and making that data sync work because that's I'd say relatively new phenomenon and it's something that we're taking advantage of every day with our clients.
Eric Dodds (13:51)
Sure. I'll use us as an example, we kind of picked up on this pattern from what we saw a lot of our customers doing. So we use Snowflake and Mixpanel actually and what we see is really for Mixpanel, instead of being linear, a user performs an action, and you send that event directly to Mixpanel, you add an additional layer or you add an additional source to Mixpanel, which is Snowflake, right? So RudderStack feeds all the behavioral data directly into Mixpanel, so you have the real-time views, you can look at your funnel behavior, all that sort of stuff. But then-
Ryan Koonce (14:32)
It handles the identity resolution in both places, right?
Eric Dodds (14:35)
Exactly. Right. So then-
Ryan Koonce (14:36)
So you get that for free, right?
Eric Dodds (14:38)
Right. Exactly. Because it's the same payload, you get a matching profile on Mixpanel and a matching profile on Snowflake. Now, because Snowflake is a data warehouse and can handle all different forms of data to Ben's point, semi-structured data, structured data, all of that, what we do is we pull additional information into Snowflake that Mixpanel really isn't intended for. Right. So if you think about pulling your salesforce data in, information from whatever marketing automation tools you have, stuff from your app databases, and you actually run calculations on that. So you can sort of calculate a lead score and other components like that, right.
Eric Dodds (15:20)
Or other sort of what we call leader account intelligence and we push that from Snowflake using our reverse EDL pipeline back into Mixpanel and it creates this really interesting feedback loop. We call it a feedback loop where now because we have more user and account intelligence and Mixpanel coming directly from Snowflake, we can build richer cohorts, make better funnels, understand deeper user flows. And that unlocks more insights for us based on what we discover in Mixpanel and then we can actually pull the results from that from Mixpanel back into Snowflake. Right? So then we can sort of syndicate that to the BI team who may want to slice data by that particular cohort, sort of augmented cohort from Mixpanel so that they can update executive dashboards in some sort of BI tool or whatever. And so you have these feedback loops where Mixpanel's getting data from the RudderStack advance stream and Snowflake creating value and then syndicating that back into Snowflake. So it's pretty cool and actually has already unlocked some really interesting things for our customers.
Ryan Koonce (16:35)
Yeah. And getting that data from Mixpanel back into Snowflake is usually super relevant to the BI team. And Ben, kicking this back to you, are you seeing anything where teams are using Snowflake and ML, and is there any convergence there or is that still sort of in its own silo?
Ben Gotfredson (16:54)
Well, I think one comment I was thinking of when you were all talking about that, that flow is just one big trend that we didn't really touch on, right, which is just the continuous breakdown of the data silos that's occurring. You see RudderStack being an enabler of it, and then you see Mixpanel making that [inaudible 00:17:14] their product. Right. And having our CIO write an article recently. He talked about just because you have a bunch of cloud-based SaaS applications in your environment as a company doesn't mean you're really like a cloud-friendly or full cloud adopting company, right? You still need the ability to have a horizontal view of these SaaS applications that's in a single source of truth of some kind, right. And the benefit is Eric, what you were talking about, like the enrichment you can do by looking at two different SaaS applications in your stack and the benefits you might get from having those two datasets from the same source.
Ben Gotfredson (17:54)
So I just wanted to touch on that. I thought that was a big trend that this whole conversation is kind of touching on. And then I think to your second point, whereas machine learning potentially playing a role in this, I think that's sort of the direction this is going, and it's the same trends that are driving the warehouse first evolution in the CDP space, or what's eventually driving this evolution ML space. And the two are simplicity, right? Lowering barrier entry, and also democratizing the data, like having a single source of truth for your ML workloads that are in one location and having super scalable storage on that end. Right. You do not want to have a siloed-out ML tool that you're having to send the data to. One of the big reasons is you're just not going to be able to run the same amount of compute or run the same amount of storage as you would, if you're acting on your own data warehouse.
Ryan Koonce (18:55)
That's absolutely true. And I think one of the things we find is that particularly for people that want to do ML projects today, the sample size is an issue. You need a massive sample, you need the ability to process and compute all that sample. Otherwise, you're just going to end up with sort of garbage in, garbage out. And for us that accurate, reliable, consistent data foundation is really important. And we're finding that this, both the ability to store, but also the ability to audit and to leverage that continuous loop is making a huge difference in the conversations we're having around the data.
Ryan Koonce (19:26)
Vijay, I'd like to bring it back to you and maybe talk a little bit about how we think about using the operations of the data to get it into a framework where now Mixpanel can take some of this information that historically is not just to identify and track and instrumented in some app, but now is being enriched and augmented by other sources, which, it's Eric's point like sales force is always kind of a thorn in our side because people are, oh, well, there is this closed one and there's this revenue associated with it and maybe a pipeline stage. Typically, we wouldn't mess around with Mixpanel in that context, but now we can. So are there other things like that, that maybe we should highlight around Mixpanel's ability to get to the truth more quickly, now that we have some of this additional information?
Vijay Iyengar (20:16)
Yeah, totally. I think, I mean, getting great insights from your analytics tool begins with trusting the data and I think historically what we've seen is there's always this kind of painful trade-off between trust and effort, where to get high trust data, you have to put in a ton of effort, or you can put in very little effort and kind of get data that is you can't really trust. And I think this ease of moving data from systems of record, which is kind of the canonical source of truth into a warehouse, joining in to get into one view that's kind of managed by tools like DBT and building like that controlled 360-degree view of whatever entities you want that can evolve over time and then being able to refresh Mixpanel with that, kind of beats that trade-off where you can kind of get with high degree of convenience you can align on the kind of core trusted data sets and then bring them into Mixpanel for fast self-serve exploration like you always could. So I think really what we're seeing there is, it kind of lets you get this fast self-serve exploration on data you already trust.
Vijay Iyengar (21:11)
I think Mixpanel superpower over something like a BI tool in addition to Snowflake is largely the exploratory nature of it where the way I think about it is kind of like web 2.0 versus web 1.0, rather than it being kind of a static dashboard that one person produces and everybody consumes as a static snapshot. It's really like being able to dig in and click into a spike and then drill down and zoom in and pan and create sub-segments and really get to the truth and I think Mixpanel's really good at that. Historically, it was a challenge to get the data in, but now I think that's become a lot easier so you can kind of get this feedback loop, as Eric was mentioning.
Ryan Koonce (21:46)
Yeah. We talk about four levels of data and it starts with the count, then segment, then forecast, then predict, and you can see where each of those areas can be leveraged across all of these systems. And I think in particular, in Mixpanel, we always talk about peeling back layers of the onion, right? Because the answer to a business question ultimately, and often results in more questions like, wait a minute, revenue, who was that revenue from? Where did they come from? What did they buy? The company, how often did they buy? And so those are the kinds of things that you have to do that exploration with and obviously, Mixpanel's a great solution for that. How do you think about the difference between sort of real-time and batch data? Does it matter? Is that something that Mixpanel's well suited for? How does it time with this warehouse-centric framework? Maybe you can talk a little bit about it.
Vijay Iyengar (22:29)
Yeah, it's a really interesting question because Mixpanel historically was built, I mean, our whole API and data model is all real-time, in the sense that events are real-time. They happen at a point in time and they get sent to Mixpanel and we can analyze that in real-time. And I know there's like a kind of, it's a contentious point because it's like who's making these real business decisions on data that's coming in the last five minutes. But I think there are really two big use cases for real-time even in this batch kind of centric world of the modern data stack. The first is kind of this immediate feedback loop of, I shipped a feature, put it out there, are people using it?
Vijay Iyengar (23:01)
Just today actually Mixpanel shipped the feature and we shared kind of this graph of the line going up and the raw log of events coming in of like, hey, people are actually using this thing. And that kind of creates this data-driven culture internally where people can just like, there's that excitement of just consuming like, oh, someone in New York has just signed up for this thing, or someone here just used this feature. That's kind of exciting. And then the second is, I think the data governance piece where you can kind of, for this like raw clickstream events, you can kind of do an event in your app and see it happen in Mixpanel instantly and you build that cause and effect. That said, I think it's kind of a hybrid world that we're building for now where it's kind of you have that real-time stream coming in and you can enrich it with this batch created on a daily, hourly basis in Snowflake, this like enriched kind of canonical source of truth data sets. So you can kind of get the best of both worlds-
Ryan Koonce (23:49)
That's right. And really look, all data isn't time series data. Right?
Vijay Iyengar (23:52)
Yeah.
Ryan Koonce (23:52)
And so I think that's where that connection happens is that some of these user traits can be updated whenever and it doesn't have to happen on a continuum.
Eric Dodds (24:01)
Yeah.
Vijay Iyengar (24:01)
Right. Exactly.
Eric Dodds (24:02)
One, just to jump in there Ryan, with a specific example, I think Vijay's point around what is real time, it really is relative and I'll just give one example. So, we had a customer who was running sort of analytics jobs from their warehouse or they were loading stuff into the warehouse and basically were producing sort of their reports on a daily basis, every 24 hours. Big e-commerce company running hundreds of tests across their site and they need to know pretty quickly when one of those tests wins so that they can implement the change and it's all about conversion and optimization. And they actually load the behavioral data now into their warehouse and refresh dashboards every 15 minutes.
Eric Dodds (24:52)
So that's really fast and it's a huge amount of data, but sort of the tech enables you to actually do that now and so for them, they consider that real-time because it's actually not practical for them to look at the dashboards any faster than that because it's not like a test is going to change, you launch a test, it's not going to change in 15 minutes. Now, there may be some other things that you actually do want to look at a live stream in real-time, but you can account for that sort of stuff. But the warehouse is really fast. Ben, I don't know if you want to jump into that, but we're seeing a lot of companies log data in the warehouse at an extremely high rate.
Ben Gotfredson (25:32)
Yeah. It is bleeding into one of the questions I saw that we got in the chats. It's good that we're answering it. I think Vijay had some really interesting points. I think a lot can be accomplished by batch but there are use cases where streaming is important and it's going to be a deal-breaker for a company. I'm thinking personally, the most relevant use case at Snowflake is analyzing customer usage, right? If a customer's deploying a new warehouse, a new feature, a new product that's powered by Snowflake, it's helpful to have real-time insights or as close to real-time as you can get on what the associated number of users are, storage growth, compute growth, and ultimately, not Snowflake specific, but even just industry-specific, I think it's a workload that will eventually get fueled off by the major data cloud providers. I don't think it's there yet, but I think that's the trend that it's going to.
Ryan Koonce (26:36)
Can you maybe drill down that a little bit? What do you mean when you say that there's a trend going there in that direction?
Ben Gotfredson (26:44)
Of streaming use cases being supported by maybe historically OAP, the [crosstalk 00:26:51].
Ryan Koonce (26:50)
Okay. Got it. Excellent.
Ben Gotfredson (26:53)
I just think there's more use cases now than there are been in the past for why [inaudible 00:26:57].
Ryan Koonce (26:56)
And Eric, talking a little bit about these different types of databases, how do you feel about the future of big data? Is it sort of a silos or constellation of services or what do you guys see at RudderStack and how this evolves?
Eric Dodds (27:18)
Yeah, absolutely. To some extent, I think we're all trying to answer those questions a little bit, right? Five years ago, everyone was asking, what is the stack going to look like? And so today, it has a certain flavor and so I think everyone's kind of figuring out what is that going to be in five years. I think we know a lot of things, right? It's very clear that the cloud data warehouse, cloud data platform is really still going to be the center and I think that comes down to a single source of truth and a lot of things that Ben mentioned. I think one of the principles that we have at RudderStack is that you need to maintain a high level of flexibility. And one thing that we see and actually Ryan, will be interested in your thoughts on this, is that the stack is getting more complex. It's not consolidating.
Eric Dodds (28:10)
Additional tools are being added and tools are actually being changed out at a fairly high rate. And so whatever the tools are, I think it will be a constellation and I think companies will increasingly adopt technology that has the bi-directional capability to maintain flexibility, right? So that you don't have to do sort of a massive migration or other components like that. You sort of establish your source of truth and then you build a constellation of valuable tools around that. And I think for sure, what we will see is the bi-directional emphasis, right? Like I want infrastructure that allows me to send data and pull data from every source in every destination to sort of maintain maximum flexibility because I think the challenge that a lot of companies face is, the business grows, it changes its response to the market and guess what? We have different needs, whether that's for sort of data, for different components of the tech stack. And so I think being able to respond to those with agility will be increasingly important.
Ryan Koonce (29:14)
Yeah. Well, I agree. I think ultimately we're seeing a lot of things happening, obviously, the martech landscape or product tech landscape or whatever you want to call us, involved a lot over the last five years. The, sort of from our seat, we don't believe there's a perfect stack. It's really, for any particular business, there's a perfect stack and it oftentimes has to do with whatever the constraints are for that business. So one, I already bought something, two, I've got particularly unique technical issues. My team has certain knowledge capital, there's lots of things going into that conversation. I think what we're seeing is, and it's going to be interesting is because in some sense tools aren't raised, right? Who can release the next newest best feature?
Ryan Koonce (30:01)
And so I think for the consumer that's great and from our perspective, everybody kind of wins because we can take advantage of these things and tell a better story faster with the data. And so, that sort of leads me into a conversation about sort of the difference between this time-series data and BI and maybe Vijay, speak to that a little bit about is there ever a situation where we sort of see, in some sense, merging of the capabilities or saying like Mixpanel where, we know there's this massively rich ability to explore and view the time series data, but also now with this bi-directional sync where I can get data into Mixpanel that before I would only think about surfacing and say maybe, a Looker or a Tableau, how do you guys think about that? Because that's to me an exciting trend that opens up some opportunities from a reporting perspective that we didn't have in the past.
Vijay Iyengar (30:57)
Yeah, totally. I think one of the kinds of core insights we had early on at Mixpanel that I think we still strongly believe is that events, when it comes to time series events are kind of the universal data model, in the sense that they're really simple, like when something happens in the real world, an event, that that is an event, right? It has a person that did the action, the details about the action and timestamp and events kind of have this natural chronological order and allow you to see kind of cause and effect, this happened before something else. And so what we're seeing is by building kind of this event native user experience and database and data model, it's really easy to model kind of the core, kind of user flows, like a funnel, kind of is somewhat universal. You can see a user funnel, you can see a recruiting funnel, you can see a sales funnel.
Vijay Iyengar (31:38)
So I think we see a lot of potential for this event-based, event-centric data model, to model all sorts of business processes in a way that's, I think, a bit more flexible and a bit more kind of maps a bit closer to what people think about when they think about a user or company or whatever else going through a series of steps. So I think that kind of gives us the ability to model a lot of the use cases in a more flexible way than a BI tool can. I think BI is great from the standpoint of generality, right? It basically boils down to supporting anything that SQL can support, which is a kind of a much vaster ocean of answers. But I think in terms of self-serve exploration and in an intuitive way, I think something like Mixpanel on top of these natural events that are generated from transactions or business interactions is really powerful.
Ryan Koonce (32:25)
Awesome. So we've got the data store, we've got the data pipe and we've got the data biz and that's a powerful combination. Anyone of you guys, I want to, before we sort of wrap up for some Q&A, wanted to make sure if there was anything else that you wanted to talk about, we jump in and say today. Did I miss anything in our little chat?
Eric Dodds (32:47)
I'll just jump on piggyback a little bit on what Vijay was saying about sort of time series data and your question around that. One thing that's really interesting, that we've seen several customers do is actually what we call sort of manufacture events or create synthetic events in Snowflake, and then send those into Mixpanel, right? So there are a lot of situations where you have non-time series data or even the absence of behavior that is helpful to track as a time series data point. And so there's this really amazing pattern we see emerging where you can create that and you have the flexibility to do that. So they're just building these tables and Snowflake represents these synthetic events in sort of the time series format, and then sending them through to Mixpanel. And it's really cool. So companies are... I think to summarize it, I would say the barriers around that are coming down just because you can do a lot of really different neat things with actually sort of creating events.
Ryan Koonce (33:59)
Oh, well, and for us it's table stakes at this point. If the data is in Snowflake and it is something that needs to be turned into some sort of synthetic event, we're going to get it up in a Mixpanel-
Eric Dodds (34:09)
Totally.
Ryan Koonce (34:09)
... and leverage it at the customer level and also gives us the opportunity to do things in Mixpanel where you're grouping cohorts and doing things in a way that, again, otherwise would've been for us, maybe more expensive in time than the value we would see out of it because there's a lot of low hanging data typically and so now again, those things start to become table stakes and every company should be doing them.
Eric Dodds (34:34)
Yeah.
Ryan Koonce (34:37)
Any last thoughts, Ben?
Ben Gotfredson (34:41)
No, I think we covered some really fun topics here. I think the big theme that I see across through the four logos here is just like the same concept of breaking down data silos and what benefits come with that and what technology exists today that helps enable that. Right? And Ryan, it's also on you, on some of these projects that you're working on, on how to connect the dots and pull that off. So I really do think Mixpanel and RudderStack stand out as two companies that are breaking down silos in a pretty unique way. So I really just appreciate getting invites to talk about these things. It's been fun.
Ryan Koonce (35:20)
Awesome. Vijay, any last thoughts?
Vijay Iyengar (35:23)
Yeah. I think totally agree with everything that's been said. I'm super excited to get more of these richer kinds of a source of truth data sets from tools like Snowflake and RudderStack into Mixpanel and see what companies do and hope they surprise us. I think there's lots of interesting use cases out there.
Ryan Koonce (35:38)
Yeah. From our perspective, it's all a win because for us, it's getting accurate, reliable, consistent data across the stack and doing something with it and that obviously starts with counting the data and segmenting the data and forecasting it and predicting the data in a way where the organizations believe it and they have the stuff that they need.
Ryan Koonce (35:58)
And so want to, let's see, hop on some of these questions here. It looks like, we have a Q&A box, I think somewhere. So somebody hops in on that. I don't see it in front of me, but I know it's there. And then let's see. So there's a question about real-time use cases for marketing. So obviously we are talking about these batch processes and getting things going and then to the extent that we want to talk about these real-time use cases for marketing, where the example was maybe somebody just completed a workout, that's an event and we would say, yes, there's lots and lots of those. And so maybe, if one of you guys want to jump in and talk about where we can leverage these events in the context of marketing, or even in the context of real-time personalization or something where we're taking advantage of these things and leveraging them against an app or a site.
Eric Dodds (36:55)
Yeah. I can jump in and speak to that. And I would say, that's actually a pretty straightforward use case.
Ryan Koonce (37:01)
Yeah.
Eric Dodds (37:01)
So someone finishes a workout in a mobile app and you want to basically sum the number of workouts they've done and if it passes 10, then you want to take over the screen and have confetti and do a celebration of their 10th workout or whatever. Right. And from the RudderStack perspective, you can stream that event in there with the incremental count of the total number of workouts that they've completed, and then that's going to hit some downstream marketing automation tool that will have some sort of logic built into the campaign that says, if a user's workout count is 10 or hits 10, then sort of do this takeover. That's actually a fairly straightforward use case and that's a reality when we think about the two pipelines I talked about where you feed a Mixpanel directly in real-time, and then you also feed it with sort of enriched data from Snowflake, the same is true of a marketing automation situation where you can actually sort of delivering things in real-time.
Eric Dodds (38:05)
Of course, you can also, another example, we see a lot in terms of personalization that's not necessarily like the user does X and then Y needs to happen immediately is on next type of behavior, is what we're seeing and a lot of that's fed from the warehouse, right? So user hasn't opened the app in three days and so you want to give them an offer next time they log in to increase their engagement or activity. Right. You can calculate that in the warehouse and sort of push that up as a trigger. So a lot of times it can be easier to build those cohorts and logic, or at least have them live in the warehouse or even build it in Mixpanel, then pull it into the warehouse. And so it can be easier to sort of do that next behavior at any time type personalization using what you've built in the warehouse.
Ryan Koonce (38:53)
Yeah. More importantly, using Mixpanel, you can define what events are actually important. So is it finish the workout or is it started the workout or is it got halfway through the workout? There's so many things that we can key on in order to determine what those events that are going to ultimately result in the trigger are and we would leverage Mixpanel to understand each of those pieces.
Eric Dodds (39:15)
Yeah.
Ryan Koonce (39:18)
Vijay, do you have something to add there?
Vijay Iyengar (39:20)
Yeah, I think that's right. For the pure real-time use case, I think RudderStack works great in terms of routing those raw events to both the marketing tool and Mixpanel. I think the other piece is around if there is something that you determine as part of your product analysis workflow, where you see that there is a sharp drop off at a particular step, one thing that you can leverage in Mixpanel with kind of this wider ecosystem of integrations or with our kind of pushing cohorts out to your own servers or to something like Snowflake, you can kind of activate those cohorts that you've built as an outcome of your analysis in Mixpanel, which again, kind of goes back to that feedback loop piece.
Ryan Koonce (39:57)
Eric, you were going to jump in on a question around CDPs?
Eric Dodds (40:02)
Yeah. And I'll just read this one out, because it's a little bit longer, but I think it's a great question. I love a warehouse standard customer data approach, can you give some insight into customer hesitancy around a warehouse for CDP versus a productized CDP like segment and particle dealing, et cetera, specifically around the speed of accessing basic things like latest event occurrences and aggregates for a given user?
Eric Dodds (40:29)
Basically asking about sort of a productized audience builder, which is a really great question. So we're seeing some really interesting architectures around this. So one is that a lot of companies are building audiences in a number of downstream tools, right? So like Mixpanel, for example, we talked about cohorts, but you see a ton of that in terms of actually acting on the various audience traits, et cetera, in the context of sort of a marketing automation tool, sales, CRM, whatever. People are building list segments, groups of people.
Eric Dodds (41:08)
And what's really cool is you can actually, and many of those tools trigger outbound webhooks that can actually feed other systems across this stack in real-time. So we have a lot of customers who actually sort of taking traits from audiences or things that happen in a downstream tool, hitting a webhook, sending that back through RudderStack so that it can actually syndicate that to the entire rest of the stack. The other thing we're seeing that's really interesting is companies and this is I think one of the coolest architectures we've seen, basically setting up a super low latency database in the cloud, like a key-value store that they can access via an API layer, so that you basically have, you're constantly populating with the event stream and from the warehouse with enriched data, a complete customer profile that use, that lives on sort of your infrastructure and is accessible via API.
Eric Dodds (42:07)
And the reason I believe we're seeing that across a lot of our customers is that when you sort of outsource the customer profile building to a third party closed SaaS tool, as opposed to doing it in something like Snowflake and then pushing it to somewhere that it's available centrally, inevitably you lose control and flexibility. And so that's just actually not working for a lot of companies now because they need to deliver more complex use cases around real-time personalization or other things like that, where the infrastructure that supports sort of that, what we would call like a black box sort of customer profile store doesn't make sense anymore. So, great question. I think, companies are doing some really neat things around that space.
Ryan Koonce (42:58)
Yeah. And from our seat, we feel like it's kind of the first inning. We're seeing cohorts and segments and different pieces of data built in a variety of places. And again, the key is how do we sync that data across these systems in a way that provides value in each of these tools where they excel and it obviously goes beyond just participants today. I mean, there's like tons and tons of different things that sort of touch this data-centric ecosystem. And so the more flexibility that we have in our ability to affect the customer and provide that information the better from our perspective.
Ryan Koonce (43:39)
All right. So I guess we can give it another minute or two, if anyone wants to drop something into Q&A, we can get some answers going otherwise we'll leave it with last thoughts and wrap up for the day. [crosstalk 00:43:55].
Eric Dodds (43:54)
I think there's another question here, Ryan. It looks like it might be a good one for you to tackle.
Ryan Koonce (44:00)
Let's see here. Oh, cool. Okay. Got it. So this question's around handling transactional use cases with regard to notifying a user around some sort of policy and having identity resolution in place can cause issues with sending the message to the wrong person. And so I think the answer is no, in the sense that, for us identity resolution again, is sort of like table stakes. We can have session based data, but if we're not tying it to a user identity in the most appropriate way, then we're losing a lot and leaving a lot on the table.
Ryan Koonce (44:47)
And so in the context of the appropriate identity resolution, what you're doing is you're basically taking anonymous identities and mapping them to known users through an identified call and Eric and Vijay, you can speak more to this about how it works in your specific systems. And so often, it's unlikely that we're going to send the wrong message to the wrong person, if we're doing identity resolution right which is sort of the point of identity resolution. And so I don't know if you guys have any comments around that, but that's how we would sort of think about that situation.
Eric Dodds (45:18)
Yeah. From my perspective, number one, great question and I know we've all felt the pain of sort of sending the wrong thing to the wrong person and that causing business problems and you-
Ryan Koonce (45:29)
We've never done that, by the way.
Eric Dodds (45:34)
In a past life.
Ryan Koonce (45:35)
Right.
Eric Dodds (45:37)
Usually just because you are trying to solve identity resolution sort of on multiple fronts or even have multiple teams handling that and I think to Ryan's point, the technology and sort of governance is there to solve it on ingest and then whatever you can't solve on ingest, because there are always edge cases you solve on the warehouse and then syndicate that back out. And so it's really, I know maybe even just a couple of years ago is a huge pain point, but it's really, the ingredients are there to fix it. And I mean-
Ryan Koonce (46:15)
You have to go through the effort and to your point, you do have to do it across the teams and you do have to have governance and you do have to have a process, right? And so that ability to audit and really look at the data and make sure it's accurate is something that also identity resolution gives you that you don't have a session-based world because you're just counting random sessions and there's no way to tie it back to a source of truth whether that's in something like Mixpanel or that's down in the warehouse, or it's in a third-party system or data source that you can map that data to, to make sure that you're doing it the right way.
Eric Dodds (46:49)
Yeah. There's another question here, is RudderStack aware of any marketing automation tools that run on top of a brand's Snowflake? That's a great question. Yeah. So I would check out a tool called message gears. It's a marketing automation tool that sits on top of your warehouse and sort of is a hand in glove fit with sort of the identity resolution in the scheme as that RudderStack flows into Snowflake automatically and sort of allows you to do sort of really powerful stuff from all sorts of marketing automation, sort of marketing segments and all that sort of stuff. So message gears, I would check out if you're interested in a marketing automation tool [crosstalk 00:47:39].
Ben Gotfredson (47:38)
I would second that. Yeah, actually I was going to mention.
Ryan Koonce (47:46)
All right. Great. Any other questions? Going once, going twice. All right. Well, listen, on behalf of everybody here, I want to thank all the attendees today and thank the panelists for showing up. It was, I think, a good session and we took away a lot. This has been recorded as well as live. I think you can get it from the Mixpanel team or the RudderStack team or the Snowflake team, I'm pretty sure. And so take advantage of that if you can.
Eric Dodds (48:27)
Thanks, everyone.
Ben Gotfredson (48:28)
Great. Thank you everyone.
Ryan Koonce (48:28)
All right. Thanks, everybody.
Eric Dodds (48:30)
Thank you.
Vijay Iyengar (48:30)
Had fun. Thanks, Ryan.
Ryan Koonce (48:32)
You bet.