Data Activation With Braze, Snowflake, and RudderStack
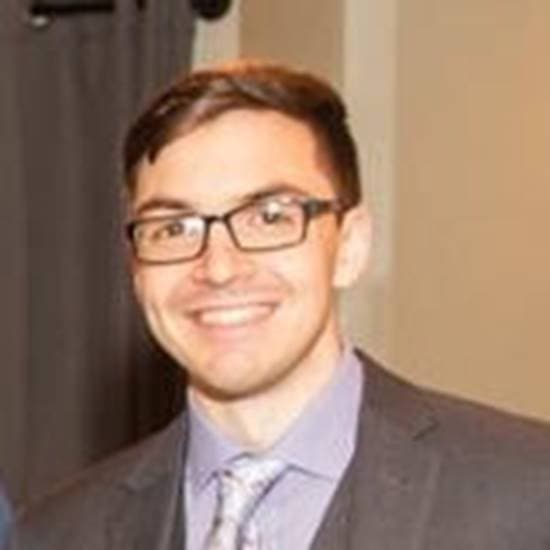
Benjamin Rogojan
Seattle Data Guy, Data Science and Data Engineering Consultant
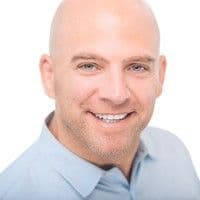
Matthew McRoberts
SVP Global Alliances at Braze
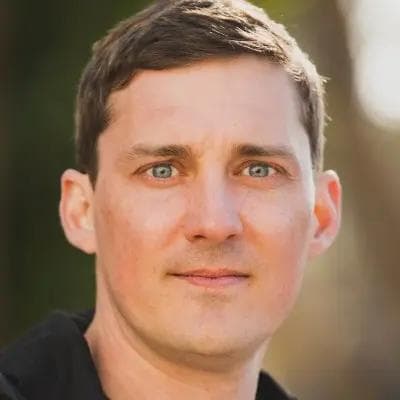
Eric Dodds
Senior Director of Product Strategy
In this webinar, you’ll learn how engineering and data teams use RudderStack, Snowflake, and Braze to power their customer data and glean key insights about their market.
As teams collect user behaviors from the web, mobile, and more, they route their data through RudderStack. This behavioral data is then loaded into Snowflake to build a comprehensive customer profile and pushed to Braze via RudderStack’s reverse-ETL tool. Cross-channel engagement metrics are then pulled from Braze Currents back into RudderStack.
- The feedback loop between Braze, Snowflake, and RudderStack
- Applications for customer data in campaigns
- Results engineering, product, and data teams are gleaning from this data architecture
Eric Dodds (00:00)
Thank you to everyone who's joining us. We're super excited to chat today. We're going to talk about data activation. If you heard what we were just talking about, we were talking really about the last decade and how we went from primitive technology to a world where we can accomplish pretty amazing things in terms of data activation. That's been a great journey. We have a special guest, so Ben has joined us. So say hi, Ben. We'll do an intro in a minute.
Benjamin Rogojan (00:27)
Hey.
Eric Dodds (00:28)
And we're going to start out actually giving this conversation some context along the lines of what we just discussed. So Ben does a lot of consulting, again we'll do an introduction for him. But he is going to give us some context about what he's seen in the industry and how stacks mature over time, which is just really helpful information. And then Matt and I will dig into where we've been and what we can do today with an architecture that delivers some pretty amazing engagement. So let's dig in. All right. Ben, you want to do a quick intro?
Benjamin Rogojan (01:04)
Yeah, sure. Hey everyone, my name is Ben, also known as the Seattle Data Guy. Basically I help companies do end to end data solutions, implementations, using a broad range of tools. And just helping them either modernize their data stacks or sometimes untangle the mess. There's plenty of times I just come in and data stack, maybe they've got all the right tools, but it's just been chaos or it's been developed in such a way that's hard to tell what's coming from where. And then also I'm a pretty big content creator across medium and YouTube in the whole data engineering space.
Eric Dodds (01:40)
Great. Matt?
Matt McRoberts (01:42)
Awesome. Thank you, Eric. Thanks, Ben. Money name is Matt McRoberts. I'm the senior vice president of global alliances at Braze. I've been in the business at Braze about seven years now. I look after what we call the three pillars of partnerships at Braze, which include technology integration, so RudderStack and Snowflake are two of our top tier partnership integrations that we're really excited to continue to build and innovate around. The second pillar would be folks like Ben in terms of working with consultancies, GSIs, growth agencies, folks that are building managed services around Braze and helping our shared customers drive best in class engagement. And then thirdly, Braze has a mature channel development program, mainly focused around regional reselling in the Asian Pacific, as well as Latin American markets.
Eric Dodds (02:36)
Very cool. And I'm Eric Dodds. I'm with RudderStack and I work on the growth team and actually manage our implementation of RudderStack. So I get to be in the guts of the product every day. And again, Ben, thanks for joining us. As you can tell, Matt and I love having a third party in here because we like to talk about RudderStack and Braze a lot, but we also want you to hear from people who are out doing the work every day. So Ben, give us some context here, we're going to spend about 15 minutes just building a little bit of context for what we're going to talk about with Braze and RudderStack.
Benjamin Rogojan (03:10)
Yeah. No, I'd love to do that. So basically I'm going to focus on talking about developing a mature data stack, talking about how I've seen different companies go about it. What I've seen in terms of like both working with specific clients, as well as just doing research and seeing other large companies and seeing what they're doing. So you can probably go to the next slide.
Benjamin Rogojan (03:31)
Not to reiterate on the same thing, but again, just my background is in data engineering, a lot of end-to-end consulting, all over the place in terms of finance insurance. Also, worked at Meta and I like to say accidental writer and YouTuber. So those are just some fun things that I have found myself doing that I really like doing. So if you're interested in learning more about data engineering, you can check out that.
Benjamin Rogojan (03:56)
All right. So just going with this talk in terms of what the focus is, basically I wanted to start out with why data infrastructure and analytical maturity is more important than ever and understanding how in the process that companies get to some sort of final end point of having a data infrastructure that they can rely on. And I like starting this by thinking the fact that for some people Excel is their mature data stack. If they're just starting out, if they're a small company, if they're just not aware of all the tools, you might see a combination of just exported excels directly from even things like Workday and Salesforce being used and conglomerated together to do a lot of analysis.
Benjamin Rogojan (04:37)
Or if they're lucky, maybe they've got one developer who's duplicated a database and they're relying off that one duplicated database to do a lot of their analytics off of. Or finally they've got some sort of half automated system that's got some things connected via various tools or scripts or something to pull in all that data into something like Postgres or Snowflake. It's not really fully connected, but it's getting to the right place. They've got some dashboards.
Benjamin Rogojan (05:03)
But the problem that I think a lot of companies face is they're going through this process is that we're getting to a point where, as the slide points out, there's more data than I think there's ever been. There's more demand for that data. There's more data sources, I think that's one of the bigger things. It's one thing to deal with larger data sets, it's another thing to deal with a larger variety which equals more connectors, which equals more upkeep and code and all that. And then of course, more data tools makes it just hard to understand what to use where, and I just obviously poke at more hype articles, which I'm sure I put out myself.
Eric Dodds (05:37)
So many hype articles.
Benjamin Rogojan (05:39)
Everything is the next everything, every platform is the next solution. And so all of this I think makes it very difficult to understand where do I take my data stack? What tools do I pick? But going back to the more data portion of this and the more variety... Sorry. No, you can go back.
Eric Dodds (05:56)
Okay. Yep. No worries.
Benjamin Rogojan (05:59)
What was I going to say? Basically I like to think that we're getting to this point where we can no longer process data in the way that we have been, which used to be very manual connections, because we're getting to this point where we need to almost industrialize our data stacks. That is to say that before, when we only needed to create 10 cars, it's very easy to do a lot of that stuff more manually, put together a lot of those pieces. But as soon as you start having greater demand and greater variety. We're pushing for that need to develop these mature data stacks and analytical processes because that's the only way we're going to be able to manage all of the data. So now you can go next slide. Sorry.
Eric Dodds (06:40)
Yeah. One comment that I think the reason I'm so excited to dig into this a little bit with you, Ben, is that for the modern person working in data or the modern person trying to drive engagement with customers. With all of the amazing tooling, it's a really important question to say why is this still hard to do? It doesn't seem like it should be that hard, but it is hard because of all these things you just mentioned, mainly the hype articles.
Benjamin Rogojan (07:12)
Speaking of articles, I've recently been reading a ton, obviously, because as a content creator you read 10 times as much as you produce. So there's two articles that I think I came across recently that I think stuck out, especially in terms of maturity and watching companies, just right out their maturity process. Because I think a lot of companies externally assume that these big tech companies, they just automatically have these data stacks that were already from day one the best of the best. But I think data stacks are a process. So looking at, for example Netflix, which there was an article put out by an author who basically discussed who's the founding engineer of the real time data infrastructure streaming platform at Netflix and like how it happened there.
Benjamin Rogojan (07:59)
He has this whole article where he discusses it. Originally, he references that they were on a traditional OLAP system using Hadoop, using Hive, which is what a lot of companies do rely upon. But what they eventually started to realize in 2015 is that in about six months, the capacity that they currently had was no longer going to be able to manage their current needs. Both on the operational side, as well as more long term analytics retention, answering questions like retention and things of that nature. And so through this article, which I think is very well written if anyone wants to look it up, they end up just going through the different steps they went through in order to go from concept of we've got six months, what can we put together in six months that will work?
Benjamin Rogojan (08:44)
And so they talk about how they've put together this streaming analytical system that is able to handle their 500 billion events in a day. It's called Keystone. Again, that can also be looked up as well, if you're interested in the underlying architecture. And that took upwards of six months. And then in 2016, they've got that MVP ready and now they're testing it out. They're getting other teams to adopt it, they're seeing if it works. They've got at this point, dozens of people using it. And that's a great start. It's never immediate buy-in. Even when I go into a customer, I usually try to get things out in some sort of MVP within that three month period where it's like we've got something that's working, we've got a process in place.
Benjamin Rogojan (09:26)
And now, in 2017 to 2019 for them, they were like let's really start ramping it up, let's set up more processes. Let's mature around that. And now they've got hundreds of people using it. And in 2020 at that point, I think they've got thousands of people using that infrastructure. And they're now looking to what's next.
Benjamin Rogojan (09:42)
And I think that's a testament to the fact that data infrastructure is that step by step process. You're not going to be there day one. Something's going to push you to needing it. You're going to go from whatever your current setup is, for them it was OLAP, to something where you're pushed to do something more, take six months to deploy that. Now you've got an MVP, with that MVP now let's see what also can improve. And then you'll continually take steps to continually maturing that. And I thought that was a great story in terms of watching someone's data infrastructure improve slowly over time and then get buy-in as well. Because again, it's both sides, you got to make the data infrastructure and you got to set up the processes and get the people bought into using it.
Benjamin Rogojan (10:23)
Airbnb's... Oh, sorry. I should breathe more. But Airbnb's story is slightly different, but I thought it was also interesting because they pointed out the fact that for them they have this giant data quality initiative where they realized that a lot of people that were building data pipelines tended to be data scientists, software engineers, which often led to problems like ownership, who actually owns this pipeline. Who's going to track this. If something goes wrong, who's responsible for this pipeline. And that was causing tons of bottlenecks in their whole process.
Benjamin Rogojan (10:54)
And so basically in 2019, they committed to the idea of this whole data quality initiative where they revamped their pipelines, they looked into standardization, they looked into setting SLAs. They looked into hiring data engineers more and setting up a culture of data engineering and building that out slowly over time. So I think they went through a similar process in terms of steps that I often see with a lot of companies, which is you often see another path that people will take is well I've got an analyst that's pulling things manually. Or, I've got a developer who's created a pipeline that works for now, but it's not his main job, or it's not their main job. So they're not focused on it 100%, which makes sense. Again, they're usually focused on delivering features and so they've just not fully taken it.
Benjamin Rogojan (11:40)
So that's generally why things eventually come to a point where you have that next step where you realize maybe we need to improve our data engineering culture, which is what I think Airbnb talks about in that article, is not just setting these new standards, but also improving their overall data engineering focus and culture. But yeah, we can probably jump to the next slide unless there are some comments.
Eric Dodds (12:04)
Yeah. I think those are great lessons because even large enterprises, Netflix is dealing with a type of data at a scale, very high definition media, that just a lot of other companies, even if you have really high scale aren't dealing with. And so it's encouraging to see that they actually follow the same process that other companies need to follow. It just happens that they're trying to solve problems with the type of media and data that's pretty different than a lot of other companies.
Benjamin Rogojan (12:35)
Yeah, exactly. And basically the point is data infrastructure, regardless of what it means to you, whatever modern data stack means for a company, whatever tools you've picked and you're relying on, it's going to be iterative. It's not going to be immediate gratification of all of our data is right, everything is perfect. I think I've got this chart from Taylor Brownlow that is pretty good. Again, another great content creator, she works at Count. This chart I think covers almost what I think about data infrastructure, but more from a team side. Just how over time you go from originally, just like I said, having a developer and an analyst where maybe just that developer somehow gets that data to that analyst. Maybe it's just a CSV dump, maybe it's a duplicate database, whatever it is. And there's no real necessary process in there. It's just they need answers, so here it is. It works for now, we don't have time to hire someone in to set up all this.
Benjamin Rogojan (13:32)
But through that, I think you start figuring out what questions you want to answer, what's important to your business. And then as you mature along that process, you realize that the next step is, like Airbnb was like we need someone that's going to manage this. Be both a combination of governance as well as maybe modeling and cleaning up some of our processes, productizing some of our processes so it's more automated, which is that next step there that they've got in that picture where it's like we got a data engineer. They're managing this a little more, they're delivering that data and data scientists and analysts can use it.
Benjamin Rogojan (14:06)
I think one thing I could say is like maybe the data scientists sometimes go a little bit further back because maybe there's a data lake and they're acting on very raw data to do ad hoc analysis. But other that, that's a mix in terms of where you are in your maturity and that's what you see in that final picture where data scientists, analytics, engineers, which often find are some split off of a data engineer one way or the other, that just act as someone that defines things a little more at a little bit of a higher level like metrics and things like that and tries to just keep everything orderly. Whereas the data engineer might just be getting the data out of logs and parsing things.
Benjamin Rogojan (14:39)
So I think this is just a great representation of what I often try to think of in terms of maturity. You don't get their day one and it's not necessarily the end of the world if you're not there day one. Most people want their data stacks to look like in this next slide, this perfect thing. And I didn't even include everything here because I didn't include reverse ETL or your ML strategy and all of these other things, which if look at the ML pipeline, that adds another set of what you're seeing in front of you in terms of like feature stores and all of that. But this alone here is your pretty general process for a lot of people where you've got your data sources, you're pulling it out using multiple ways often. Maybe you've got your CDP, maybe you've got something that's just doing direct event processing. You've got batch processing, you've got streaming, it's all maybe going to raw. Maybe it's getting staged and cleaned up a little bit. Maybe you've got some DBT in there to do some transforms.
Benjamin Rogojan (15:30)
But all of this is like heavy lifting. If you've got like a data governance tool and a data lineage tool and all of that, all of those are different initiatives that take a long time to implement. Even working at Facebook, or now Meta, it felt like we would add a new piece to data lineage every few months where now I got to go in my pipelines again and add in a new little ticker to say are we going to track this piece of information?
Benjamin Rogojan (15:52)
So most companies are constantly adding in a new piece of this whole stack. And I think that's okay. I think the next slide is where you find most people. If you start here and do this well, you're already, I think, winning. If you can somehow get your data from raw to some sort of core data set and you know you can rely on it, that's a great place to start, because that will let you build up all the use cases that you want. And then you can start iterating, if you need streaming, how are we going to add in streaming? If you want to start doing some sort of loop backs or feedback loops that you guys are going to show here in a second. You've got good data, you know you can rely on it. Now you can you go on that next step.
Benjamin Rogojan (16:34)
I put this joking part below with data governance. It probably exists to some extent, but it's a combination of Excel sheets and your observability. Well, you're kind of doing some things or you're kind of tracking some of the lineage. But when you're just starting, that might be okay because you don't know what you're going to keep, you don't know what's actually going to be valuable. So I think it's okay to just get started with even with testing, it's like well we've got some manual tests, but they're there. So when we automate them, they're ready to just plop in somewhere into some other tool like Bigeye or something. So all of these things I think just are step by step process and where you are I think it's just not a race. It's definitely a marathon, it's definitely like every year there's going to be a new tool anyways. So if you need to spend a few extra months focusing, it's okay.
Benjamin Rogojan (17:20)
All right. So, basically I wanted to give some ideas in terms of how to mature your data stack from more of a philosophical standpoint in terms of what you should be doing. I think having clear processes and standards make sense, especially standards around how you're naming data and even little things like that I think make a big difference. Even small silly things like having consistent naming conventions for dates, make it easier for analysts to work with. I often think of data as a product. With any product, you should think about how you're signifying what a value is. I don't always want someone to have to go back to some data dictionary to figure out what it is. I want them to know this is a boolean field because all our boolean fields is underscore, etcetera. It seems boring. But I think those little things just take that data stack to the next level.
Benjamin Rogojan (18:14)
And then having clear processes, just make sure you're not just like doing ad hoc work all the time. Obviously it's unavoidable to do completely no ad hoc work, but making sure you're actually productionizing things in a way that is sustainable, robust, and will continue beyond just the time that you work at the company. I think having us all to understand the data is also good, not just pulling in data because you think it might be useful, but understanding if that data is supported, if that data's still valid, I think this doesn't exist as much as it used to. You used to have SAP tooling. You pull in a table and it have 100 columns and half of them wouldn't be filled or something or they'd be there just because they wanted it more flexible. So I think we do live in a world where a lot more fields tend to be valid. But having a valid understanding of how your data operates, whether it updates old data points or whether you're just relying on pens, whatever it might be, I think those little things make a difference.
Benjamin Rogojan (19:11)
More from a higher level, having clear goals I think are very important, obviously. Why am I actually pulling in this data? What am I going to do with it? What decisions is it going to drive is really going to guide you and everything else.
Benjamin Rogojan (19:21)
And then also being honest with what your company needs. With Netflix, they didn't start with streaming because they didn't need it at first. But then eventually they were like we need streaming analytics. So there often comes a point where streaming becomes much more important and that's when you push over to that. And per use case because there are pros and cons to basically every tool. Machine learning's great. But if you don't need it, it's just another thing you have to manage. So that can really weigh down a small tech team, depending on the size.
Benjamin Rogojan (19:50)
I can go ahead and go to the next slide. And then how to ensure just your data team succeeds. I like to think of this as, again, a ship that's sailing through the ocean. You can't really neglect the daily tasks like making sure it's clean, making sure you're not getting too many barnacles on the bottom of a boat or something like that. But at the same time, you can't forget the big picture because you have to know where you're going. You have to know if I want to go to Canada, I want to get to Canada and not Antarctica.
Benjamin Rogojan (20:20)
So you have to also at the same time constantly make sure you know where you're going. And this is just done by, on the little picture side, doing things again, like I said, setting standards, limiting technology to what you need and trying to avoid duplicate technologies as much as possible, because that just adds into the possibility of creating some spaghetti pipelines because you've got so many different forms of an iPASS system here, a normal ELT system here, and all of this just bumps into each other and you don't know where or what data's going. So trying to limit to what you need.
Benjamin Rogojan (20:51)
Making sure you're doing things like testing for quality data, especially if you're using that data for something that's high level reporting that needs to be accurate. And then also treating people like people and not computers. I just like creating data sets that are very easy for people to use because obviously and computers can translate a lot, they can translate a lot of complex data sets and data structures. But oftentimes analysts and people who maybe range in terms of their technical abilities, maybe some of them know SQL, some of them don't. Making sure you have a data set or data sets that can be usable by a wide variety of people I think is valid since I think a lot of people have good intuition in and can use data well, as long as it's structured in such way where they're not going to start pulling data inaccurately. So I often like to say treat people like people.
Benjamin Rogojan (21:36)
And then big picture, kind of the same thing. Set your big goals, reassess them occasionally. You don't need to constantly look every month, but every so often reassess those goals, make sure you're going towards them. Make sure you're aware if you're going to run out of space and you need to switch over to streaming or your pipelines are getting too slow so you need to address that, all of these things. But overall, set those big goals. I like saying set big goals because I think it's really easy to get stuck in goals like I want our team to complete 10,000 Jira tickets this month. Which sure, it sounds big. But I think generally when it comes to me, it's like how do I set a goal where I want to eliminate the need for those 1000 tickets altogether, work and projects in terms of goals. So always trying to figure out how you can do bigger process improvements goals and not just very work and operational goals.
Benjamin Rogojan (22:32)
And then obviously don't constantly pivot. Obviously some pivoting is always required, but if you constantly pivot, you'll never know where you end up with the constant pivoting. So, that's my tips there.
Benjamin Rogojan (22:44)
So basically just wrapping this up. My key takeaways is data infrastructure is a process. It will always take, I say, a few iterations. It'll just continue iterating forever in perpetuity. As data gets more complex, as we continue, there's going to continuously be either better tooling or updates to your current tooling that you can constantly take advantage of. Again, depending on where you're at in your data maturity level, it's going to constantly grow and change based on your needs of the company and the different tooling that come in. I like to say again, that data is a product, that comes from my data engineering side. Quality matters, what it looks like matters, usability matters just as much as a product would be if you're building a software product. And then of course finally just have clear goals on where you want to go with that data. What do you want to actually do with it? What problems are you really trying to answer overall? And just make sure that's clear from the get go I think is very important. Yeah. That's my... I think Eric is on mute.
Eric Dodds (23:48)
I was muted. That was super helpful. And I think having that big picture and those reminders of the data stack as a whole and how you step towards a place of maturity and not necessarily bring on things that you don't need is super helpful. So thank you for that.
Eric Dodds (24:08)
Now we're going to zoom in on a really particular use case within the data stack. So really helpful information on how do we structure this? How do we step towards a mature data stack? And so now Matt and I are going to talk about as you step towards that, what does it look like to deliver, and I love the concept of a data product. And so Matt and I are going to talk about a big data product that's always the tip of the spear when it comes to any customer data specifically. Is how do you drive really good engagement and communication with your users, customers, potential customers?And that in and of itself is a data product that's composed of multiple different pieces of infrastructure.
Eric Dodds (24:56)
So quickly, we'll talk about what RudderStack is and what Braze is just to make sure everyone's on the same page. And then we'll get really specific and show you how to do some pretty cool stuff with some modern tools. RudderStack, so we're an end to end warehouse first CDP. And you may be asking what that means. When we say warehouse first in the context of the CDP, our belief is that you should build a CDP on top of your warehouse. The warehouse should be the source of truth. It's where you can get all of the data, and you need a tool that actually makes it easy to pull that data in and then get it back out from any source to any destination. And then that enables you to build a best of breed stack around that. You have full control over the data on your own infrastructure, and then you can use best of breed tools to activate it, whether that be analytics or engagement tools. Matt.
Matt McRoberts (25:57)
Awesome, great explanation. Appreciate that Eric. And Ben really enjoyed your section as well. So just a quick top line on who Braze is and where we operate is Braze is one of the leaders in the customer engagement space. We were founded in 2011, so we're over 10 years old now at this point. And really Braze is about again, providing our customers the ability to listen to their end users, understand their respective preferences, and then use that listening, that insight, to deliver best in class engagement experiences across a suite of cross channel messaging.
Matt McRoberts (26:35)
We work across a whole host of different customers across different categories, including travel and hospitality, media and entertainment, retail, healthcare, and wellness. And you can see in the middle there where Braze operates and again it delivers these best in class engagements. It's whether it's an email or a push notification or SMS, we've got a whole host of what we call in product experiences around again, working within whether it's your .com or your mobile app. Again, it delivers against these memorable messaging experiences at scale. And as Eric had alluded to, is we are very much part of the data ecosystem. So we work in tight partnership from an integration standpoint with RudderStack to ensure that we're streaming data in so that we've got the most accurate, real time portrayal of your users. So you can have those most relevant, personalized experiences.
Eric Dodds (27:28)
Awesome. Okay. We're going to go on a quick journey and look at some ways that companies throughout the past have delivered a data product of engagement. So we'll just start here. This is the oldest, but most common way to engage. And Matt and I like to call this the spray and pray setup. So you have a website, a mobile app or multiple properties. You're collecting data, maybe you have a tool in there that's helping do some of the integration and have a data layer like RudderStack. But in the end you send this data to a terminal destination and it just fires off all these messages. That's not bad, but what's happening is that you have just a local optimization. You can only get this one type of data into this tool that probably does a limited number of things. And you're just sending out a lot of messages based on data that's probably old, stale, all that sort of stuff.
Eric Dodds (28:33)
But let's say even if you are doing that in real time, which is great, you still only have one type of data, so you can only send a limited number of types of messages. So, an autoresponder if someone signs up. Okay, that's great. Or the drip campaign. Well, you can only do that based on behaviors. But there's a lot of other things happening. So this is the old, but most common way to engage. Get this one type of behavioral data in through a linear system and push out a bunch of messages. And Matt, I'm sure are this is the world that you've lived in and this is still pretty pervasive, right?
Matt McRoberts (29:11)
Yeah. And you hit it on the head Eric, it's so one-dimensional when you look at the concept of consumers expectations in terms of how they want to be spoken to, how they want to have these interactions with brands, is this batch and blast world. This spray and pray world. Again, in today's day and age where you've got these really robust iterative technology stacks, this has become the legacy way of how brands engage.
Eric Dodds (29:39)
Yep. Okay. Here's a better way to do this. So we're collecting the behavioral data from apps and websites. That's key. That's just a key component. Understanding how our users behave and triggering real time messaging through a tool that is multi-channel and has a robust feature set in order to engage with a customer where they're at, as opposed to just a one dimensional channel communication strategy.
Eric Dodds (30:09)
But then the other side of it, and this is where things get really cool, with a technology partner like Snowflake that both Braze and RudderStack work really closely with, is that you can add more context. This is where things start to get really powerful. So instead of just the behavioral data, you can pull in additional data. So let's say you bring in ad performance data and combine that with the behavioral data. We all know that maybe users who are required from certain channels may behave differently, or have higher propensity to churn or other things like that, and bringing in that other data is really helpful.
Eric Dodds (30:42)
Also, there's a lot of other data that lives in different parts of the stack. As Ben was talking about, a lot of companies, just by the nature of them being around for a while and going through the process of iteration, they may have transactional data in a system that's been around for a long time. And to Ben's point, they don't need to change that. It works really well for what it does, but it also has this contextual information about how the users made purchases or engaged in certain ways that's really important context for the engagement and communication.
Eric Dodds (31:18)
So in this more modern way of doing things, we're pulling the behavioral data in, we're pulling other contextual information both from cloud platforms and then also other parts of the stack. So that in Snowflake, you're building a fully complete picture of what's going on with that customer. And I think this is important Matt, both in terms of their journey from a behavioral standpoint, but so the context around all of those touch points and really building that and having a complete journey and profile in the warehouse.
Matt McRoberts (31:51)
That's exactly it. The world is really started to calibrate around first party data. Because again, you need to be confident. You need to, again, provide that personalized experience, and you've got to be able to have a lens to that consumer across a number of different touch points. And I think again when you talk around the benefits of working along a RudderStack and a Snowflake. And again these robust, real time iterative user profiles, that's exactly what you're looking at.
Eric Dodds (32:18)
Yep. And I just saw a really good question come in. Please feel free to put your questions in. We'll plenty of time at the end to do Q&A, so please put your question to the Q&A. Thank you to Caleb who just asked a question. We'll pick that up in the end, but great question.
Eric Dodds (32:36)
But Matt, this is way better, but it's still not the best way to do it. And the missing piece of the puzzle here is that this actually still has the engagement piece as a terminal destination. And that's really the biggest problem. And in reality, if you want to say it the irony of the linear architecture, is that the engagement data itself, the messages, the users' interaction with those messages, is actually customer journey data. Those are touch points that are really helpful context to complete the picture. And so we have this missing piece of we have all the behavioral data, we have all of this other data from cloud platforms and from other places of the stack, great, we have all of this. And then it's like, we're generating a lot more data here that's good context, but we want that to be available to the rest of the stack.
Eric Dodds (33:38)
So this is the coolest way to do this. And actually, I'll just say on a personal note, going back in 10 years to your point. I dreamed of being able to create an architecture where essentially every destination for data is also a source. And that was possible 10 years ago, but now it's actually, I don't want to necessarily say easy, but I'm going to say easy. You can wire this stuff up pretty quick.
Eric Dodds (34:11)
So I'm going to just walk through this really quick, and then Matt I would love for you to talk us through some examples. So we collect the behavioral data. RudderStack collects that in real time, can send to Braze. So the baseline use case that we talked about of user signs up, we want to send an automated message to them in real time. Great. You have that linear flow. Super easy. All of the other customer data is loaded into Snowflake. Again, RudderStack has multiple different types of pipelines to get all the data into Snowflake. And then you can build all those really interesting audiences, segments, insights, push those actually back into Braze so that you get that complete user profile back in Braze. Great. Well now I have way more context, way more interesting segments, et cetera.
Eric Dodds (34:54)
And then the coolest piece of the puzzle, which would love to hear you explain the Braze Currents product. But all the engagement data that's happening as users interact with these messages, get routed back through the system. So RudderStack pulls all of that data in, and you can use that data in Snowflake to further refine those segments, further understand the journey. And then again, push that value back into Braze so that Braze has the most up to date, most complete picture of the customer in their journey. And that really drives the highest level of engagement. So, Matt, do you want to talk a little bit about Braze Currents?
Matt McRoberts (35:33)
Of course, and you hit it on the head Eric, is this concept of the iterative feedback loop. Braze, one of our foundational beliefs is this idea around democratizing data across the ecosystem because it's only going to lead to better customer experiences, better engagement and really better personalization for your end users. And so we have a proprietary product, as Eric alluded to, called Currents that allows you to stream all that marketing performance data back in the third party tools. So in this case, as Eric alluded to, what was opened, when it was opened, how it was opened, in terms of all these messaging experiences. You get to stream that data in real time back into tools like RudderStack. So that when you look at this concept of a user profile, it is up to date, is enriched. And again, it can benefit the broader messaging experience and the broader engagement experience across the ecosystem. And so Currents is a very important product of how Braze democratizes data throughout the technology ecosystem.
Eric Dodds (36:36)
We didn't put it on this diagram just because architectural diagrams can get really busy. But selfishly, as someone who does a lot of work with analytics and helps to build these architectures, I think one of the interesting things here is that it really makes a lot of the analytics pieces way more powerful because you can actually get that engagement data and visualize the customer journey in way more interesting ways. Usually that's a lot of behavioral data or stages that people go through. Maybe you're getting that from whatever, some other system.
Eric Dodds (37:11)
But actually having the email engagement data pulled in. And even if you think about understanding things like a multi-touch attribution model or beginning to build that, having the engagement data is such a key piece of that puzzle. And it's been really hard to get until now, especially from a streaming standpoint. I remember back in the day, it's like I do the nightly job and the data's a mess. So you have to run a transform and I'm doing so much work here just to get it. So to have that in a flexible format streaming real time is pretty incredible.
Matt McRoberts (37:45)
Yeah. And one of the things, a common theme of today is this concept of just iteration. As Ben alluded to, that there are different maturity curves in terms of how people are going to continue to build out the respective growth, growth teams and growth strategies. That's another thing that a benefit of the concept of democratizing data is it breaks down a lot of organizational silos. It used to be, as Eric alluded to, 10 years ago you had an email team, maybe you had an advocacy or a loyalty team, you had obviously maybe top of the funnel advertising team. And again, it's being able to get this data across the organization, you're breaking down both those data silos, as well as those organizational silos, which at the end it benefits everyone in terms of just better engagement, better messaging experiences and better customers, happier customers at the end of the day.
Eric Dodds (38:37)
Absolutely. Very cool. Did you want to touch on these? Well, I guess you touched on these pieces, didn't you?
Matt McRoberts (38:45)
Yeah. this is a great summary slide, isn't it? In terms of the benefit and the value of working with RudderStack and Braze. As we alluded to, you've got this concept of real time, which has honestly been a farce for many years in terms of really what real time truly means. But again, if you think around interactions and having conversations with brands, if it's not real time, you're going to be left behind. So, that becomes absolutely critical. And again, identifying the right technology partners to build out your stack itself. This concept of a continuous feedback loop, and making sure that as you're able to push your data to continue to hydrate and basically enhance those user profiles so they're up to date and as personalized as possible. And then just again, is making sure that you're looking at advanced engagement tools that work together in concert. So again, you're not doing things in piecemeal, you're breaking down those organizational and those data silos to just get the best full picture of your consumers.
Eric Dodds (39:45)
Yep. For sure. And I think the only thing I would add to that is as you think about this slice of the stack, to Ben's point, data infrastructure can become very complex. What we're seeing is that companies who are implementing use cases like this are choosing tools where there is freedom of data and where most tools in the use case are both a source and a destination in the stack. And so that allows you to create these interesting loops or get data into places way more easily. And you don't have to build bespoke solutions in order to move data out of one system into another. All right. This is where you can learn more about Braze. Anything else you want to say here, Matt?
Matt McRoberts (40:41)
Just a little plug in terms of where you can get more information on RudderStack and Braze and Snowflake and how we all collectively work together. Braze has a repository that we call Braze Alloys, which is in essence our portfolio of different technology partners. And so you can go in and see case studies in terms of how customers have used both Braze and RudderStack. You can see documentation of how to stand this up. In addition, within Braze Alloys you have a repository of all our consultancies, like the Bendz of the world. That again, can help you define your best in class engagement strategies, get your data architecture into shape and help you iterate towards creating best in class engagement.
Eric Dodds (41:19)
Awesome. All right. And RudderStack, we love talking to people. So feel free to reach out to us. You can read our blog, you can also sign up for the app and I would really encourage you to do that as a first step. You can get data flowing from a mobile app or a website into Braze very quickly. It's just simply a matter of installing an STK, putting your Braze credentials in, and the data will start flowing, which is pretty cool. And then of course we have documentation about Currents and all that stuff as well.
Eric Dodds (41:55)
Let's do some Q&A. So we had a great question come in. I'll take this. And please put your questions in the Q&. Let me pull the little window over here so I can read it. Caleb asked and then kid of answered his own question, but it's such a great topic we'll dig in. Does the Snowflake aggregation layer create enough latency to be limiting for real time use cases? And then Caleb responded to his own question, I just have to give him credit here. He said, two RudderStack pipelines, one site to Snowflake to update the profile in real time, one Snowflake to Braze to update. That makes sense.
Eric Dodds (42:33)
And yeah, Caleb, I would just add to that. There are two pipelines feeding into Braze, so there is the real time pipeline directly to Braze. So, when you think about the basic use case of behavioral triggering. So maybe a user requests a coupon code in the mobile app. Great. You want to send them an in-app message, an SMS, an email, wherever you engage with them on their customer journey, you want to send that immediately. Great. That is a real time data feed directly from RudderStack to Braze. And then the pipeline from Snowflake into Braze can really run on any schedule you want. And that just depends on the use case. We have some customers who run them really fast, we're talking minutes, so Braze is getting a pulse of the latest thing. But depending on your use case, a lot of customers will run that every 30 minute or so. And that's more around profile demographics, segmentation, all that sort of stuff. So, great question. Anything to add to that, Matt?
Matt McRoberts (43:44)
Yeah. Furthermore, Braze is a very large customer of Snowflake. So we use Snowflake as our proprietary data lake. And so again, a lot of times, like there is that latency because the data is already in Snowflake within the Braze instance. So again, that's why combinations like Braze plus RudderStack plus Snowflake work so efficiently.
Eric Dodds (44:04)
Yep. For sure. And then don't want to get too technical because there's a couple other questions coming in, but if your use case is what I would say, like Matt I loved that you said real time has been a term that's abused. So RudderStack goes to Braze realtime, realtime, if we want to call it that. If you need the complete profile updated realtime, realtime, and presently available, there are some really low latency options that allow you to essentially pipe the data so the data's flowing from Braze into RudderStack in realtime via Currents. And then RudderStack is also feeding the behavioral data in. And so you can literally pipe that into a low latency store and have that per available across your stack. Some really interesting architectures there and having the engagement data from Currents as part of that real time feed is really interesting.
Eric Dodds (45:00)
Okay, cool. Another great question came in. How long does it take to actually set up the architecture? So let me go back here. How long does it take to, to set up this architecture? Really good question. So in terms of just getting the end to end data flow set up, and I'll just speak from our experience doing this a bunch of Braze customers, Matt. But you can get this set up in days really and have data flowing literally from RudderStack realtime into Braze, RudderStack, Snowflake, Braze, and then Braze, RudderStack, Snowflake, Braze. All around the circle in a matter of days. It's pretty easy to set these pipelines up. And then of course what takes time to mature to Ben's point is that you're building your interesting segments in cohorts in Snowflake. And you're doing whatever transformations you want to do on the warehouse in order to set that up. But in terms of getting the data flowing in this, we see this happen in a couple days to get the basic use case running. How about you Matt?
Matt McRoberts (46:16)
Yeah, that's exactly it. The great thing about today's modern streaming ecosystems is that you can get that data flowing, that lifeline of what's driving your engagement strategy, literally to your point Eric, you can get that set up in days. And then contingent on what become your core strategies from a messaging standpoint, you start to set that up. And again, you can start to get that data flowing through the process itself. So again, legacy technology has the reputation of taking too long in terms of just getting stuff stood up, a lot of complications along the way. And I think in today's modern streaming ecosystems, you're able to get these tools working together in concert very quickly. And then again, a common theme that we keep coming back to is iterate from that. Continue to iterate on that strategy, test and learn and continue. Get better from an engagement standpoint.
Eric Dodds (47:11)
Totally. I think one thing that's a small detail, but that is I think really important piece of the puzzle here that's worth mentioning, is that one of the reasons you can get these use cases running really quickly is that from the Braze and RudderStack perspective, we know the schemas really well by now. And so all the schemas from the Braze data flowing through RudderStack are standardized. And then Snowflake is a first class citizen as a warehouse destination for RudderStack. And we have standardized schemas that we put on the warehouse. And so we actually have DBT models that are prepackaged to do some of that interesting segmentation for you automatically because we know the schema of the Braze Currents data, we know the schema of the behavioral data, we know the schema of the data from the other stack that's pulled in.
Eric Dodds (48:02)
Maybe not necessarily data from a legacy system, but of course you can transform that. But all these are known schemas, and so you can actually do some really interesting things really quickly because you're not having to build a data model. All of that comes out of the box of the tools. And then Snowflake is world class in terms of their support for our standardized schemas. And so it's a pretty elegant solution, is what we hear from a lot of our customers.
Eric Dodds (48:30)
Okay. Matt, question for you. This is a great question. This looks great, personalized customer experience. But the question is, what are some specific examples of things that you've seen Braze customers do with this sort of feedback loop or iterative flow that they weren't able to do with a linear flow? Do you have just a couple of actual use cases that you've seen customers implement with this that are cool?
Matt McRoberts (49:04)
Of course. When we were just going through that broad background slide in terms of how long Braze has operated and customers and categories that we have strong credentials in, I alluded to hospitality in particular. And so we work with a broad array of different folks in the hospitality space, from the likes of Delivery Hero and Glovo. And so folks that have built on-demand delivery services, all the way through to QSRs themselves. In particular Pizza Hut is a great example of really a pioneering brand that is continuing to really push how they leverage data and technology to drive best in class engagement.
Matt McRoberts (49:43)
And so with Braze, and in this case using other tools within the ecosystem, they're able to build these multi-threaded cross channel personalized engagements that deliver the right experience at the right time through the right channel. And then again, it's based on the performance or the interaction of that. Be able to fuel that data back into their ecosystem so that they understand what are preferences around time of delivery, maybe day parting in terms of, was it lunch? Is it dinner? Again, understanding specific preferences in terms of whether it's ingredients or different specials. Activating that across their promotional schedule as well.
Matt McRoberts (50:24)
And so, one of the things that you kept coming back to Eric is how do you get that data in terms of marketing performance back into that user profile, so that you're continuing to really iterate and understand who that consumer is? Because personalization has come such a long way as well, it's no longer just first name, last name, it's all these other spectrum of how folks want to be spoken to when they want to be spoken to, what channel they want to be spoken into. And so by having that continuous feedback loop with the RudderStacks and the Snowflakes, that really helps us hydrate those user profiles as I alluded to earlier.
Eric Dodds (51:02)
Yeah, totally. That reminded me of one use case. So we have a customer in the FinTech space, and I think having the feedback loop is not only good for driving strategic engagement, but this particular company had a massive spike in usage and call it unusual behavior during the whole GameStop saga. And so it's FinTech, and so you just have this flurry of activity that is really different than what you normally see, because it's over-indexing. It's not the day to day, because there's some external thing happening. And so it was really fascinating for them to observe the way that, and they are also a Braze customer, but observe the way that users are engaging with the app and even engaging with messages. And then really be able to ingest that data in real time and understand we probably need to adjust the way that we're talking with people, because the way that they're engaged with our standard day to day messaging is not appropriate for the context of what's happening now.
Eric Dodds (52:16)
They're opening the app 20 times an hour or something like that, and the standard messaging is not appropriate for what's happening now. And so it was really cool for them to be able to pick up on that, respond to that really quickly. We're seeing something here that's abnormal, we're going to go into Braze and adjust the engagement with the customer for this period of time. But it's hard to do that unless you have all those touch points happening on the feedback loop.
Matt McRoberts (52:45)
No, that's a great point. We talk a lot about sometimes it's knowing not when to send that message and being able to have that data to inform that holistic perspective. You're exactly right.
Eric Dodds (52:58)
Awesome. Well, thank you everyone. No more questions, so we'll actually give everyone five minutes back. We like to be generous as possible with the time and respect your time. And it's always nice to have a few minutes before the next meeting. So we want to give you five minutes back. Thank you so much for joining us. Please reach out. I'll just really quickly show you this if you want to get in touch with Braze, and then show you this if you want to get in touch with RudderStack. And we will catch you on the next webinar.