The State of Data Engineering 2023: Reality Check
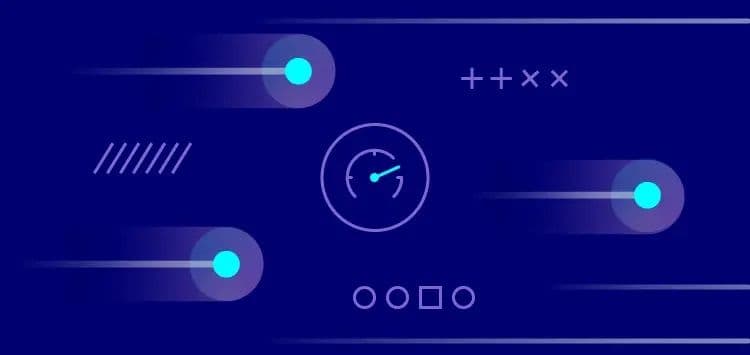
Every January our team steps back to reflect on what has happened in the data space during the prior year and discuss the major trends we believe will shape the year to come.
Multiple folks, whom we greatly respect, have already written about this, predicting everything from the rise of data contracts to operational analytics. Looking back at the developments and all the investment in the data space in the last couple of years, 2023 seems to be perfectly poised for these ideas to take off and become mainstream.
We generally follow suit in our annual analysis, focusing on the development of the data stack as a whole and the specific types of technologies that are driving that development.
But 2023 isn't an ordinary year, so we are going to switch things up (don't worry, we will write a follow-up post on our 2022 predictions).
2023: The Data Stack gets a Reality Check
After a bull run decade, the markets in general have seen massive corrections. While the jury is still out on whether we will enter a recession and if it will be as bad as the 2000 bubble, companies from startups to large public enterprises are playing it safe and actively cutting down burn by laying off people.
There are still exciting technologies being developed that will shape the future of the data stack, but the hard reality is that both executives and individual contributors are thinking less about emerging technology and more about whether their jobs will be impacted. Data teams are no exception.
In response to uncertain times, data teams are giving their stacks a reality check and going back to the basics, making sure their work is mission-critical to their companies.
This often looks less like shiny new tech and more like maximizing the value of core infrastructure so you can do more with less.
How data teams can make 2023 count
With this context, we'll outline the top 6 things we see our customers doing to ensure they and their businesses make every team member, tool, and data point count this year.
1. Find every cost efficiency in your data stack
This one seems obvious, but due the complexity of the modern stack, it will look very different at different companies.
Vendor cost control
Many companies are getting rid of tools that are underutilized or not renewing contracts, but we also see customers getting creative on how to maintain tooling with better cost control.
Here are a few specific examples from our customers:
- Leveraging data lakes for cost savings at scale: regardless of the warehouse/lake/lakehouse debate, most companies run both a data lake and warehouse. We've seen multiple high-scale companies move ingestion primarily to their lakehouse and run jobs to get the data into their warehouse downstream.
- Controlling volume for specific integrations: Khatabook collects hundreds of millions of events per day, not all of which need to be sent to their marketing automation tool. They use RudderStack's Transformations to filter events to that destination, significantly decreasing cost (check out the webinar to get more technical details).
- Leveraging APIs instead of SaaS: Here at RudderStack, we hit the Clearbit API for enriching leads in our event pipeline (as opposed to paying for the Salesforce app).
Eliminating hidden costs of in-house builds
We've seen many data teams planning to mitigate one of the most pernicious costs burdening them: engineering time spent on maintaining internal builds.
Ironically, these often start as cost-saving exercises, but at scale, and when engineering resources are more scarce than ever, it can make far more sense to leverage your talent on business-critical work as opposed to pipeline plumbing.
Two specific examples we've seen recently from our customers:
- Deprecating in-house or forked SDKs: it's not uncommon for companies to build their own web and mobile SDKs for various kinds of data capture, but at scale dealing with cookies and schema management is a huge lift and easier for a dedicated vendor to manage.
- Deprecating basic in-house pipelines: we see in-house pipelines for things like reverse ETL all of the time. As a one-off connection, they aren't too hard to build and run. As things become more complex, though, dealing with maintenance and volume becomes a time suck, causing many data teams to look for out-of-the-box replacements that cost far less than a data engineer (and can give those engineers their valuable time back)
2. Mitigate security risks
Companies can't afford unforced data security issues in 2023. A PR nightmare, security review and massive cleanup project could mean competitors get the upper hand, or even worse, threatens survival.
We've seen customers tackle security on a small scale and large scale. Here are examples of each:
- Implementing a data governance plan (for real) - Data governance is a journey, not a destination, but we've seen multiple data teams cleaning up data governance debt by updating and implementing things like integration-specific PII policies.
- Deprecating risk-prone tooling like Google Analytics - Litigation related to 3rd-party storage of personal data is showing up more often and we've seen many of our customers deprecate tools like Google Analytics and work with their analytics teams to rebuild that functionality on their data warehouse with a visualization layer.
3. Talk to stakeholders on other teams
Data and analytics are only a means to an end, which is some sort of business outcome like improving website conversion rates or product engagement. In 2023 the teams responsible for those use cases will be under immense pressure.
While data teams power the data flows behind the projects, they can be disconnected from the end business outcomes themselves. 2023 is the time to build those relationships and it starts with getting in touch with marketing, product, and CS leaders and developing deeper understanding and empathy for their use cases.
Not all these conversations need to be at leadership level—if you are an individual contributor on a data team, proactively reach out to a peer analyst working on a marketing use case and figure out what's most important.
For example, a senior data engineer who works for one of our customers began spending time with the paid advertising team to understand their needs and identified opportunities where data could help improve targeting and decrease acquisition costs significantly (20-30%!).
4. Identify skill gaps on the data team and proactively fill them
In the face of layoffs and hiring freezes, data leaders will be under pressure to deliver with limited resources.
Ideally the team as a whole can come together and determine how to fill gaps, but individuals can make a huge difference on their own, even if it's taking a course like Andrew Ng's introduction to ML to better support data science projects.
We recently saw a pipeline engineer at a customer company work with the analytics team to scaffold a dbt model for analytics. Their knowledge of the schemas and data sources sped up the project significantly and they were able to level-up their SQL modeling skills through a real use case.
5. Ship projects that impact business outcomes
If companies can’t afford to face unnecessary security problems in 2023, they can’t afford NOT to ship the key data projects that will impact the core business.
Many companies already know what these projects are, but have struggled, for a variety of reasons, to ship them.
In 2023, it will be critical for data teams to demonstrate business outcomes from their projects.
It's not enough to ship the recommendations project—it needs to demonstrably move the needle or be shut down quickly. Did the model actually increase repeat purchases? If so, by how much and what is the estimated revenue impact?
This is ultimately about turning the data team into a revenue center, not a cost center.
Whether data teams are building churn models, attribution models, full-funnel analytics or personalization, this is where we are seeing the most creativity relative to the cost-cutting measures mentioned above.
For example, one of our retail customers put off buying expensive enterprise software to drive recommendations and had their engineering and marketing teams work together to leverage existing infrastructure like Redis and their headless CMS to power personalization use cases.
6. Be vocal about the impact you are having
Taking on a personal marketing project might not sound appealing to data engineers, but the current environment means it's critical to create visibility around the impact you are having, both in terms of cutting costs and in driving results.
This is true for both leaders and individual contributors.
Present at an all-hands meeting, write internal (and external!) blog posts and look for opportunities to highlight your work at conferences (like our customer Acorns recently did at Coalesce).
We'd love to chat with you about your plans for 2023 and share more examples from our customers. Reach out to our team.
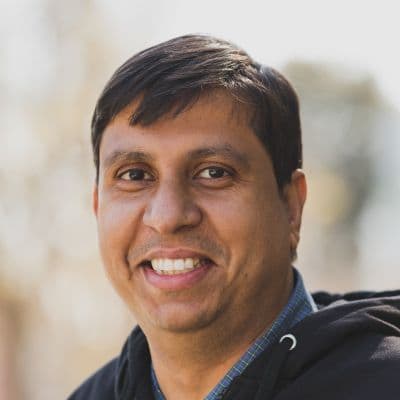
Soumyadeb Mitra
Founder and CEO of RudderStack
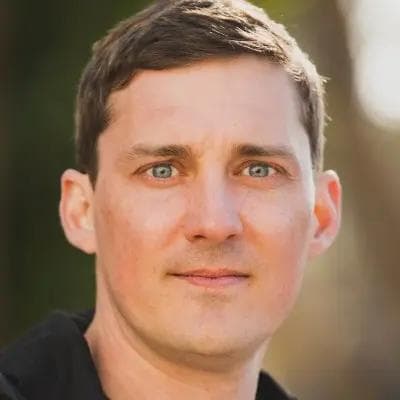
Eric Dodds
Senior Director of Product Strategy
Recent Posts
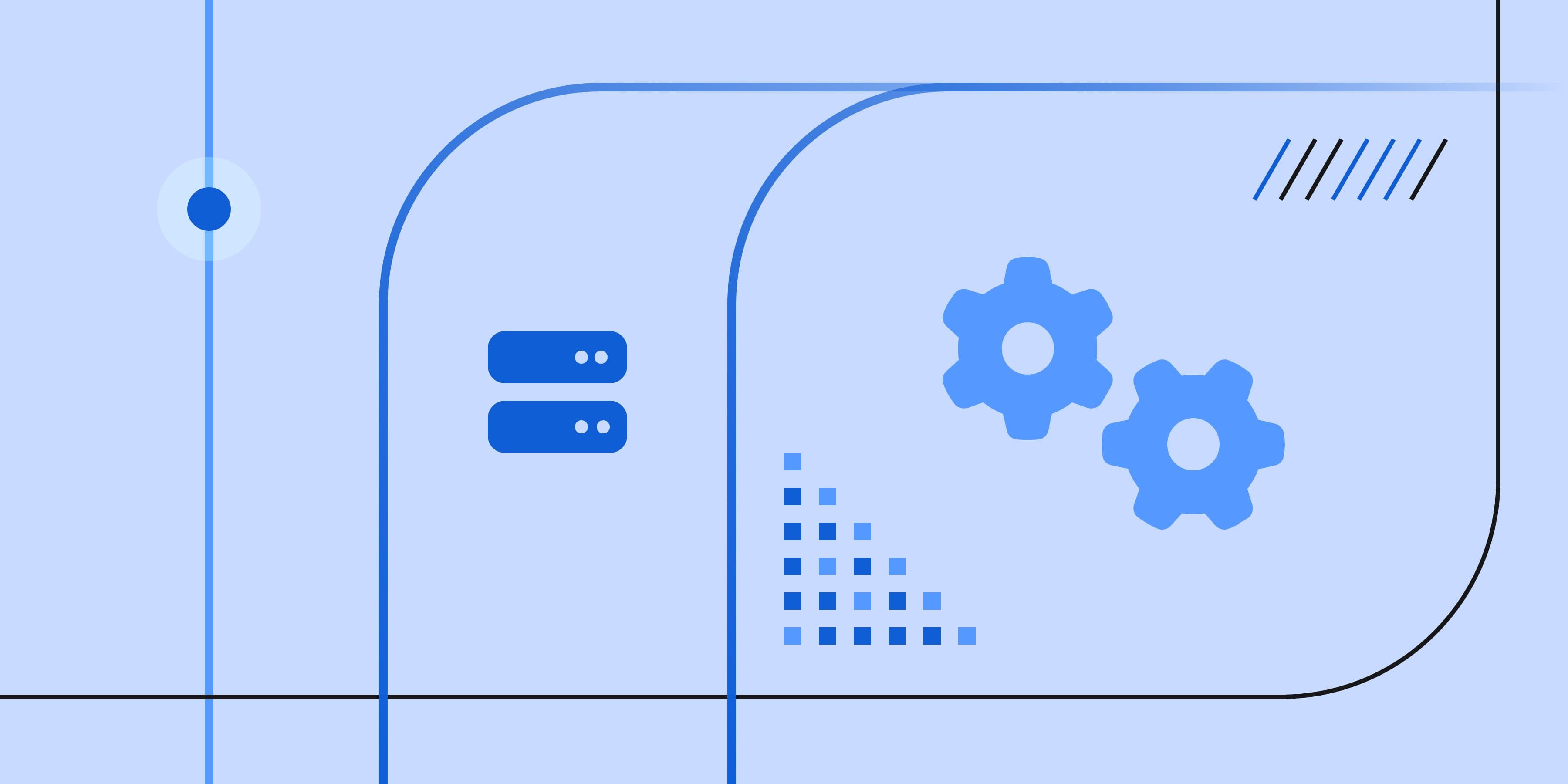
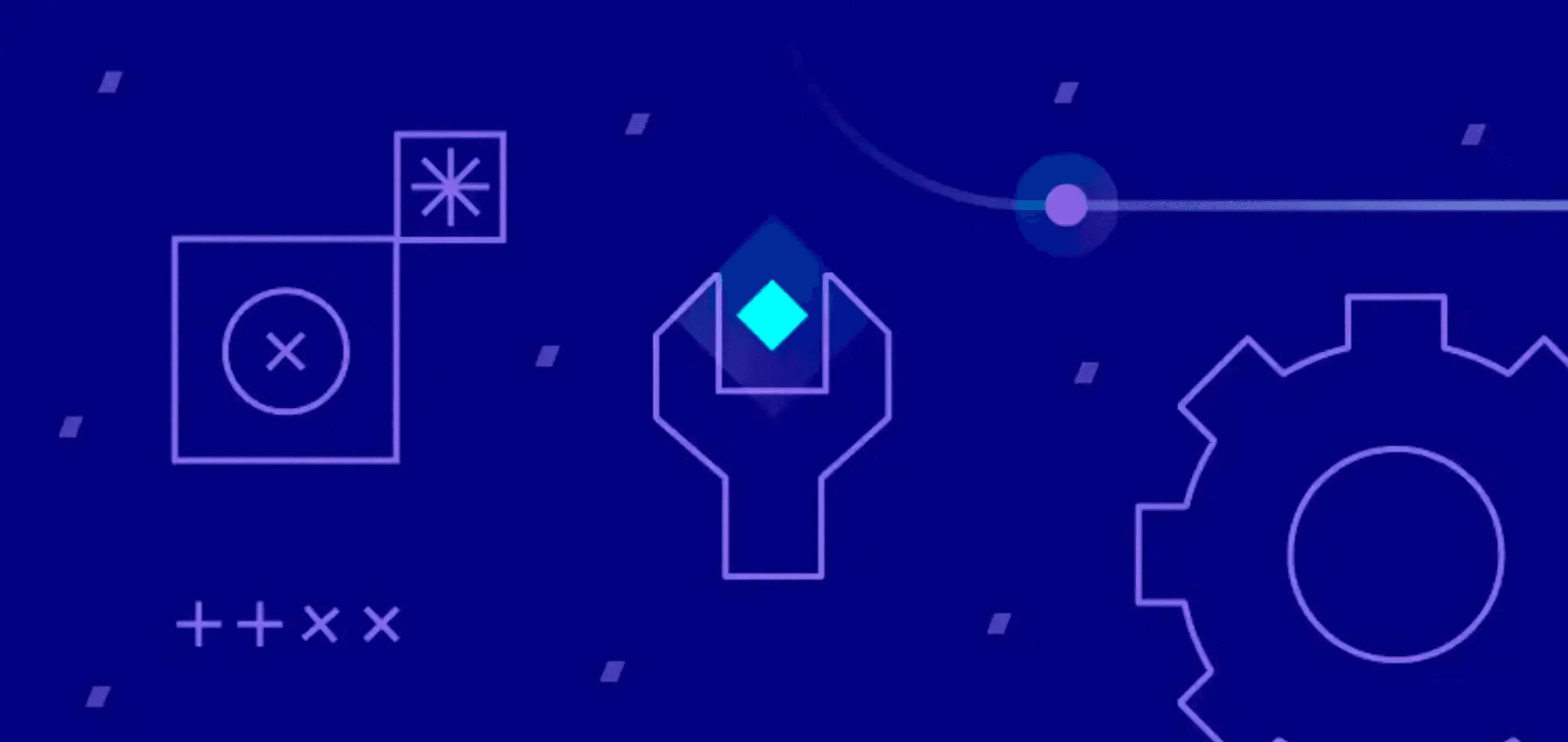
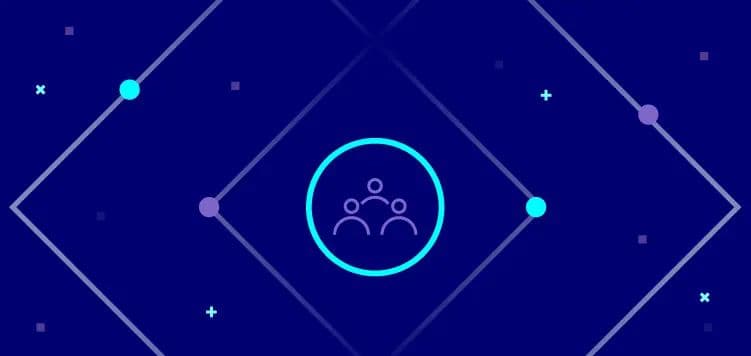