What is Entity Resolution?
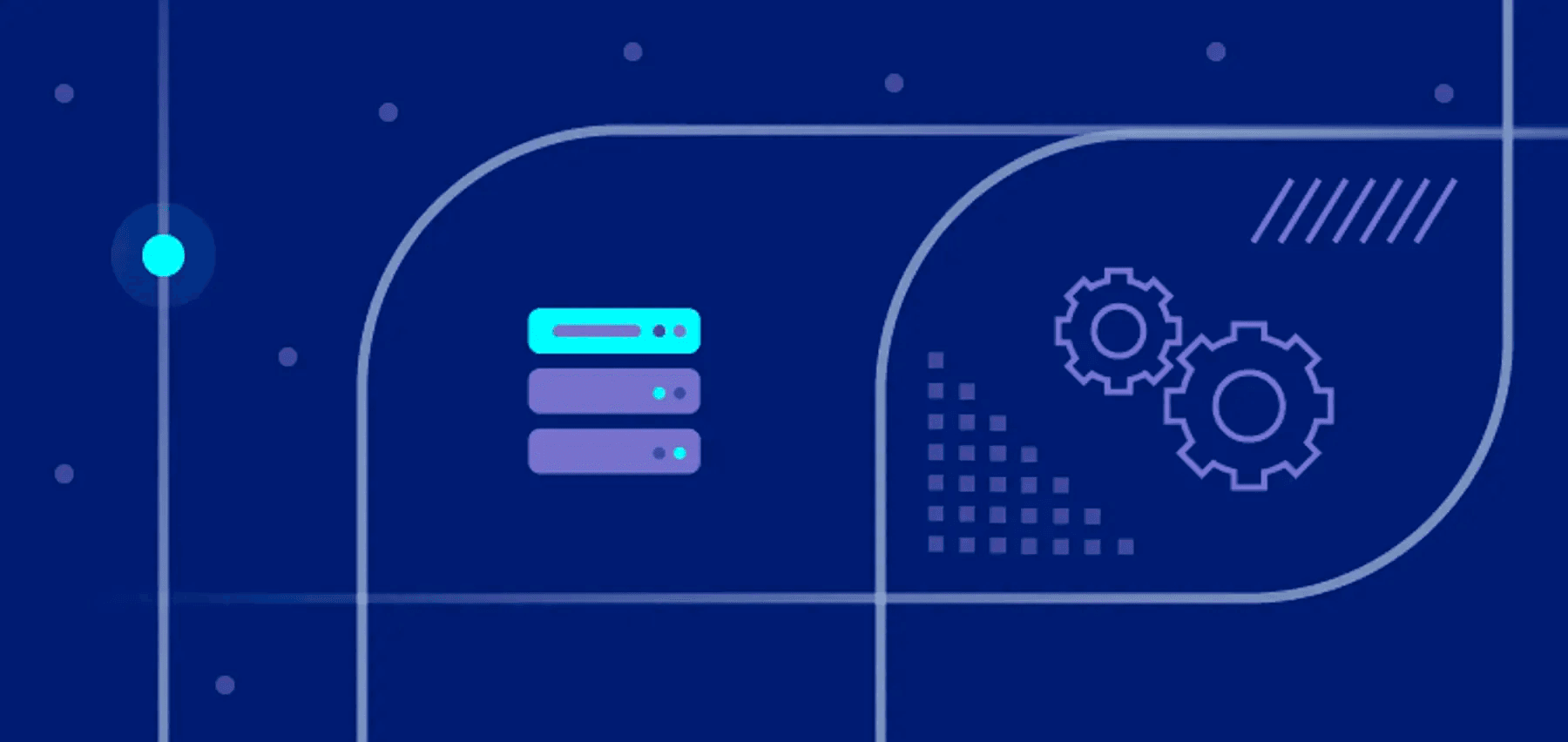
In your master data management (mdm), unique identifiers or "entities" such target demographics fields could see thousands of references in disparate data sources. These references can vary based on the time and place of customer interactions with your brand and offerings. Without proper data integration and data quality initiatives, your data can evolve into a complex jumble of data records and facts with no rule-based resolution process or validation, making them practically unusable.To enhance the data quality for informed decision-making regarding your customers and products, it's essential to have a comprehensive understanding of these unique identifiers. This involves a real-time view of customer interactions with your business, which can be passed onto downstream tools in your CRM. Furthermore, understanding the performance of your products and the purchasing behavior of each customer will require data integration from different data sources. This is the core initiative of master data management (MDM), which involves data matching and eliminating duplicate records to present a complete 360-degree view of each "noun" you care about. The resolution process is aimed at elevating your data records' quality, providing a comprehensive perspective for better decision-making.
What is Entity Resolution?
Entity resolution, often referred to as entity matching, is the procedure of linking records that pertain to the same real-world entity, like an individual, company, or household. This process enables a better comprehension of the relationships each entity has to the others, rectifying data inconsistencies, and integrating data. It's an essential part of the data integration exercise, particularly when it comes to connecting offline and online data from different data sources. This process is critical in the master data management (MDM) initiative to ensure data quality.In the realm of master data management (MDM), a real-world entity can be any discrete unit that your business aims to quantify. For instance, if you're part of a grocery delivery company, individual people might be your primary data records. However, your data sources may also include larger "households" as unique identifiers, crucial for your initiatives such as sales and service delivery. The culmination of this data integration process is a unified, rule-based record for each entity. This record contains all pertinent information about the entity, consolidated from different data sources into one place. It's ensured through a rigorous resolution process that the data quality is top-notch, with no duplicate records or conflicting records hindering the data matching process.Let's delve deeper with an illustration of a real-world entity, such as a household, as tracked by a grocery delivery firm. On Monday, a unique identifier - an individual residing in the household - navigates to the grocery delivery company's website and engages with a customer-service chat feature. On Tuesday, a different entity from the same household dials the customer service phone number. The customer service agent assisting the second user is interested in understanding the data points discussed in the first user's web chat, as well as any previous billing or interaction history related to the household members. Thus, the data teams at the grocery delivery company should initiate entity resolution algorithms and processes to link records and achieve record deduplication of all household-related data records. This enhances the quality of customer data available for decision-making, thereby improving customer service and sales initiatives. The process also illustrates a practical use case of data integration from different data sources to create a single view of customer records.
Entity Resolution vs Identity Resolution
Identity resolution is a critical part of data quality management. Identity resolution is essentially a type of entity resolution where the primary focus is on individual users. It involves data matching and deduplication of customer records to achieve a 'Customer 360' perspective - a comprehensive single view of a customer's interactions on a site. On the other hand, entity resolution, in general, is a broader term referring to the method of linking related data records. The objective is to attain a complete view of the targeted real-world entity, which may include different entities, not just individual users. It involves decision-making based on unique identifiers, data points, and machine learning algorithms to ensure a consolidated view from disparate data sources. Both these resolution processes play a vital role in master data management (MDM), improving data integration and data quality across different data sources, and effectively aiding in record linkage and record matching.The notion of identity resolution has long been a common topic of discussion in business circles, more so than entity resolution. Marketing professionals, advertisers, and other business stakeholders have been striving to develop an effective single view of the customer for years. Various Customer Data Platforms (CDPs) are competing to solve this challenge. Up to now, entity resolution has mainly been a topic considered by data teams. The comprehensive practice of entity resolution can include the advantages of identity resolution, as well as the benefits of clear views of other crucial entities for your company, like user accounts or households. This process is integral to data quality and decision-making, involving techniques like data matching, record linkage, and duplicate records deduplication. It also employs different data sources, unique identifiers like phone number or date of birth, and machine learning algorithms for real-time resolution. The goal is to ensure accurate customer records in your CRM, which is key to successful data integration initiatives and master data management (MDM).The primary methods for identity resolution and entity resolution bear a remarkable similarity. If your organization possesses the capabilities to perform entity resolution on your datasets in your data warehouse, you're already well-prepared to execute identity resolution within the same environment. The resolution process for different entities in your data sources, be it customer records or unique identifiers like a date of birth or phone number, can be streamlined using the same algorithms and machine learning techniques used in data matching and record linkage. With the right data quality and data integration initiatives, you can effectively manage duplicate records or false positives, ensuring a single view of your data for improved decision-making.
Common Types of Entities
Every business prioritizes different core aspects tailored to its specific business model. For consumer-facing businesses (B2C), the key focus is usually on the individual consumer, as they are the primary market for their products or services. Meanwhile, in business-to-business models (B2B), the focus often shifts to a business account or a prospective corporate client, encompassing several individual users.
The dynamics of these central aspects can be quite varied and detailed, depending on the company's specific market. Take, for instance, a company that sells pet food online; it might center its attention on each pet as a separate entity and link them to their owners to send targeted, pet-specific food recommendations.
Common focal points for B2C businesses typically include:
- Individual customers
- Product range
- Subscription services
Whereas, B2B businesses often emphasize:
- Users within a business
- Work teams
- Other businesses as clients
Benefits of Entity Resolution
Entity resolution is critical for several reasons, particularly in the context of data management and analysis:
- Accurate Data Analysis and Decision Making: Entity resolution ensures that each entity (like a customer or product) is unique and distinct in a database. This clarity is essential for accurate data analysis. When entities are not correctly resolved, it can lead to misleading insights and poor decision-making.
- Enhanced Customer Experience: In customer relationship management, entity resolution helps in accurately identifying and understanding customers. This understanding enables businesses to provide personalized services, recommendations, and support, leading to improved customer satisfaction and loyalty.
- Efficiency in Data Processing: Resolving entities reduces redundancy and inconsistencies in data. This streamlining makes data processing more efficient, saving time and resources in managing and analyzing data.
- Compliance and Risk Management: In sectors like finance and healthcare, where compliance with regulations is crucial, entity resolution helps in accurately identifying individuals, thereby aiding in fraud detection, risk assessment, and compliance with legal requirements.
- Enabling Advanced Technologies: For technologies like machine learning and artificial intelligence, clean and well-structured data is a prerequisite. Entity resolution contributes to the quality of data fed into these systems, enhancing their performance and the accuracy of their outputs.
- Data Integration: In cases where data is collected from multiple sources, entity resolution helps in merging and reconciling this data effectively, providing a unified view that is crucial for comprehensive analysis.
- Cost Savings: Following the "1:10:100 rule" in data quality, resolving entities at the point of entry is more cost-effective than correcting or dealing with the consequences of unresolved data later. This proactive approach saves significant costs related to data cleanup and the fallout of poor-quality data.
Entity Resolution Use Cases
Entity resolution has a wide range of applications across various industries and functions. Here are some notable use cases:
- Customer Relationship Management (CRM): By resolving customer entities, businesses can avoid duplicates in their CRM systems, enabling them to provide better, more personalized customer service and targeted marketing efforts.
- Fraud Detection and Prevention: In banking and finance, entity resolution helps identify fraudulent activities by linking seemingly disparate transactions or accounts that actually belong to the same entity, aiding in the detection of complex fraud schemes.
- Healthcare Data Management: In healthcare, entity resolution ensures that patient records from various sources are accurately merged, leading to better patient care, more accurate medical histories, and improved research data quality.
- Supply Chain Management: In supply chain and inventory management, entity resolution aids in accurately tracking products, parts, and components, leading to more efficient operations and reduced redundancies.
- Marketing and Sales Analytics: By resolving customer and product entities, companies can more effectively segment their market, tailor their marketing strategies, and understand customer preferences and behaviors.
- Compliance and Regulatory Reporting: For industries subject to stringent regulatory requirements, such as finance and healthcare, entity resolution helps in ensuring accurate reporting and compliance with regulations like KYC (Know Your Customer) and AML (Anti-Money Laundering).
- Machine Learning and AI Training: In the field of AI and machine learning, clean and accurately resolved data is essential for training models. Entity resolution ensures the data used is free of duplications and inconsistencies, leading to more reliable and accurate AI models.
- Social Network Analysis: Entity resolution is used to identify and understand relationships between individuals in social networks, which can be vital for market research, political campaigns, and social science research.
These are just some of the use cases we are showing for inspiration but the possibilities are endless.
B2B Entity Resolution Sample Use Case
In B2B environments, the importance of entity resolution lies in its role in delineating customer interactions with a product. This involves identifying each specific entity and then grouping these entities under related and parent categories.
Consider, for example, a fictional company like DataStream. DataStream tracks a variety of entities related to its products and users, such as:
Product-Related Entities:
- Software Solutions
- Data Streams
- Integration Tools
- Output Formats
- Data Sync Processes
User-Related Entities:
- Individual Users
- Customer Accounts
- Project Teams
- Client Companies
To effectively understand how a client company engages with DataStream's services, the company must perform entity resolution for all these nuanced entities, encompassing both their product offerings and user interactions. DataStream then aggregates this detailed data to the company level, which is crucial for deal-making. Through this process of entity resolution, DataStream ensures a consistent use of language and metrics across all entity levels, providing a comprehensive 360-degree perspective of each user, team, and client company.
What is an Entity Relationship Diagram?
An Entity Resolution Diagram (ERD) serves as a model that outlines the relationship between different entities that are of significance. The ERD can be employed to devise a conceptual scheme for the other entities that your organization focuses on, thereby determining how to utilize the data from these interconnected entities to guide your record linkage process. This data integration strategy is integral to the resolution process, aiding in accurate decision-making and enhancing data quality. By leveraging unique identifiers such as a phone number or date of birth, ERD can aid in data matching and record matching, reducing duplicate records in your datasets. This process forms a critical part of master data management (MDM), contributing to a more effective and efficient data entry and validation system, ultimately leading to improved customer data handling in your CRM.
For instance, imagine you manage an enterprise specializing in online plant sales. In this scenario, the primary real-world entity of interest is the customer, who forms the backbone of your business by purchasing your plants. You might also want to track different entities, like each plant bought by a customer. The unique identifiers of these entities can then be used to tailor future initiatives such as personalized offers for related plants or products that assist in the care of their current plants. This approach enhances the customer data quality and aids in effective decision-making for your business.In the resolution process, it's crucial to link individual actions taken by a user back to the real-world entity, in this case, the user itself. An Entity Relationship Diagram (ERD) similar to the one described below illustrates the entity matching between different entities - the user, a plant, and distinct user activities such as viewing products on the website. This type of data integration is a critical part of entity resolution and helps in enhancing the quality of datasets from various data sources.
Deterministic vs Probabilistic Entity Resolution
When it comes to entity resolution, deterministic entity resolution, also referred to as "rules-based matching," is a popular choice. This approach involves establishing specific criteria to identify and merge duplicate records. Deterministic entity resolution is known for its simplicity and efficiency, making it ideal for straightforward use cases with well-structured data. For instance, when matching and consolidating zip codes within household entities, rules-based entity resolution can be highly effective.
Probabilistic entity resolution, also known as fuzzy matching, utilizes machine learning, artificial intelligence, or predictive models to effectively detect and merge entities through record deduplication. In numerous use cases of entity resolution, data is often stored in diverse formats and locations, making it impractical to establish exact rules for record unification in advance. At large-scale enterprise companies, fuzzy matching logic is commonly employed for entity resolution.
How Does Entity Resolution Work?
At a broad level, the process of entity resolution consists of four key steps:
- Data ingestion is a crucial step in optimizing entity resolution and machine-learning models. It involves making sure that data is easily accessible in a centralized location. Often, this begins with consolidating data into a data warehouse, which serves as the foundation for entity resolution processes.
- Deduplication is the process of consolidating duplicate records to minimize complexity and redundancy within each entity.
- Record linkage involves using rule-based or fuzzy-matching algorithms to determine which records pertain to the same entity despite having different data, such as various interactions on different dates.
- Canonicalization involves the process of gathering and merging data from linked records to create a consolidated entity. This ensures that all relevant data points are stored within a single entity, promoting data quality and accuracy.
Tools for Entity Resolution
There are several companies and tools that specialize in entity resolution, offering solutions that range from software platforms to consultancy services. These tools and companies help businesses accurately identify and link data across different sources and formats. Here are some notable examples:
Data Integration and Quality Tools
- Informatica: Specializes in data integration and quality, offering entity resolution as part of its broader data management solutions.
- IBM Entity Analytics: Part of IBM’s analytics portfolio, focusing on data quality and insights, including entity resolution.
- SAS Data Management: A comprehensive platform that includes entity resolution within its data integration and management solutions.
- Talend Data Quality: Offers a suite of data quality tools, including entity resolution, as part of its data integration services.
Machine Learning and AI-Driven Solutions
- Tamr: Utilizes machine learning for entity resolution, catering to large-scale and diverse data environments.
- Senzing: Focuses on AI-powered entity resolution, identifying relationships and enhancing data analytics accuracy.
Specialized Entity Resolution and Data Matching
- Dedupe.io: Tailored for deduplication and straightforward entity resolution tasks, suitable for smaller datasets.
- Data Ladder: Offers specialized solutions in data matching and entity resolution, frequently used in marketing, CRM, and MDM.
Final Thoughts
The process of entity resolution is crucial for companies as it allows them to gain a comprehensive understanding of important aspects like customers, households, and products. Whether businesses opt to develop their own entity resolution solutions or utilize algorithms and platforms provided by third-party vendors, it is essential to have a complete view of the entities that play a significant role in driving their operations.
Organizations must take action with their entity data. RudderStack enables the extraction of data from company data repositories and facilitates its integration with various tools essential for business purposes. RudderStack assists data teams in connecting entity data from different sources and creating unified customer profiles. Try RudderStack Profiles today.
Recent Posts
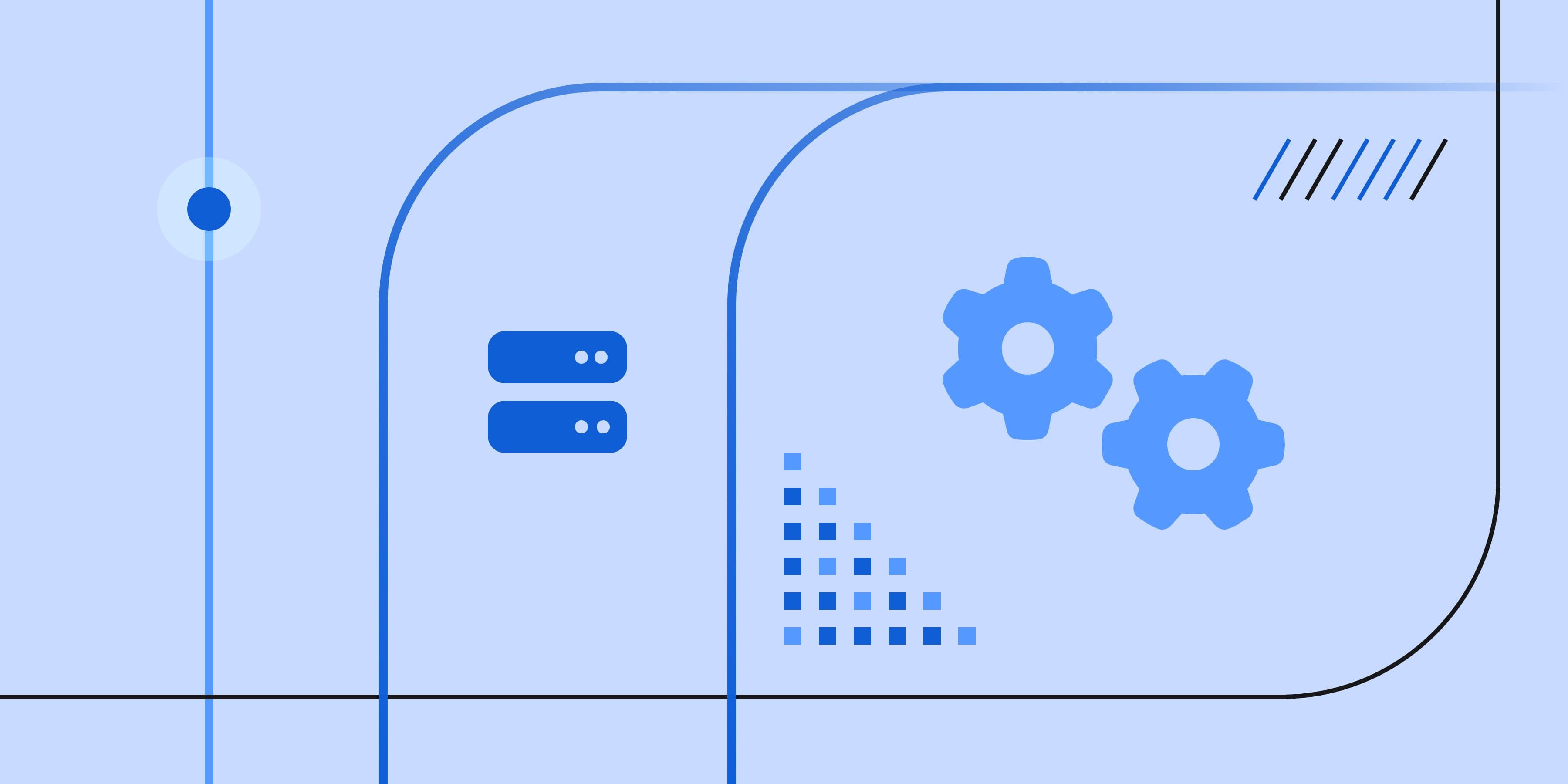
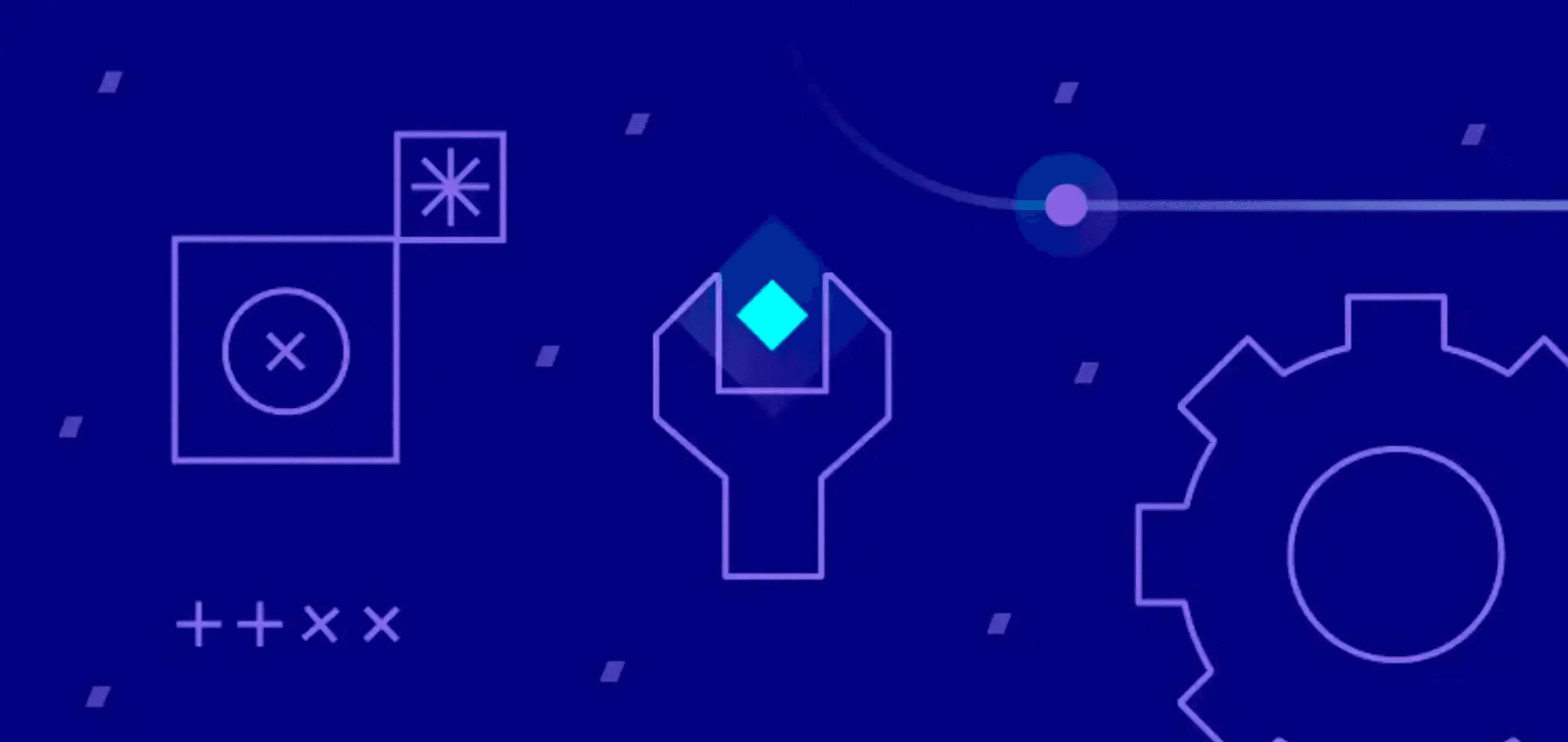
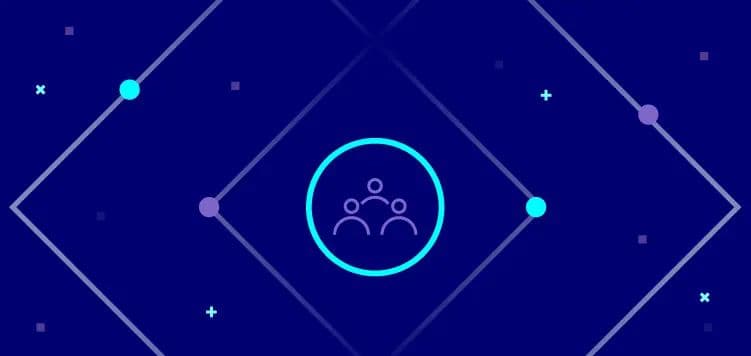